Memetic quantum optimization algorithm with levy flight for high dimension function optimization
Applied Intelligence(2022)
摘要
Quantum-inspired heuristic search algorithms have received widespread attention in solving the function optimal solution. However, the existing quantum search methods may lose their efficiency for high dimension function problems. To solve these problems, this study develops a novel memetic quantum optimization algorithm with levy flight (called MLQO) by exploring the principle of memetic computing and Levy flight. First, a memetic framework based on quantum mechanism is designed to balance the global search and the local search, which makes all particles gain a better experience from a local search. Second, an efficient local search strategy with levy flight is designed to enhance the searchability due to short walking distance and occasionally long jumps to be made by the particle. Furthermore, the lifetime of each particle is defined to determine whether a particle needs to be redistributed using the levy flight method in the search space. Numerical experiments are performed to evaluate the performance of the proposed algorithm by comparing it to other optimal algorithms. The results demonstrate the superiority of the proposed algorithm on well-known unimodal and multimodal benchmark functions.
更多查看译文
关键词
Quantum evolution,Levy flight,Memetic algorithm,High dimension function optimization,Swarm intelligence
AI 理解论文
溯源树
样例
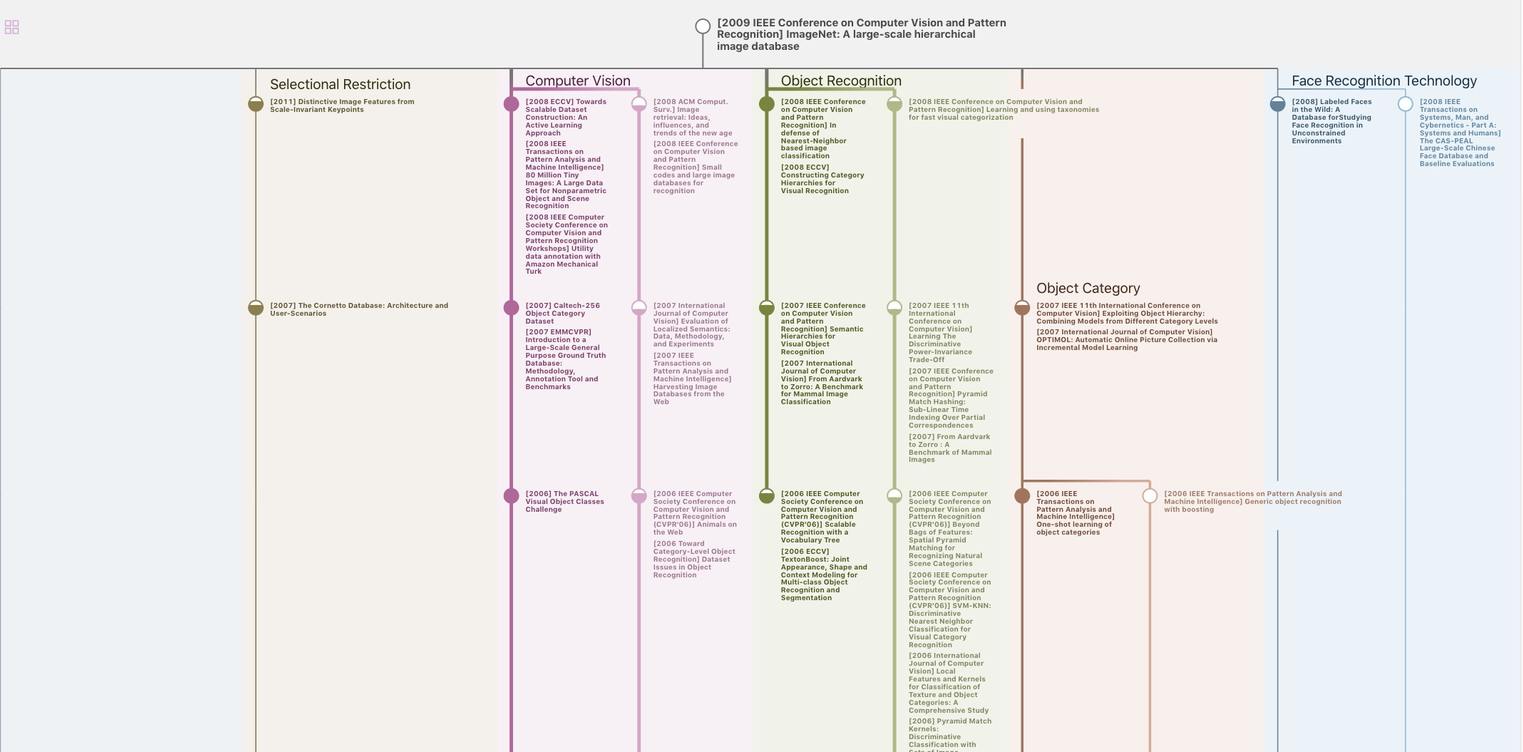
生成溯源树,研究论文发展脉络
Chat Paper
正在生成论文摘要