Diversity in news recommendations using contextual bandits
Expert Systems with Applications(2022)
摘要
Contextual bandit techniques have recently been used for generating personalized user recommendations in situations where collaborative filtering based algorithms may be inefficient. They are often used in cases when input data are dynamically changing as new users and content items, such as news, constantly change. Contextual bandit methods sequentially select articles for recommendation to a user and continuously modify their strategies so as to present users with articles that maximize clicks. However, exclusively focusing on maximizing the number of clicks can lead to over-exposure of certain articles, while under-representing others. In an era of ever growing demand for digital news delivery, this, in turn, invokes the important notion of presenting news content to users in a “socially responsible” way. To this effect, we introduce a technique based on the contextual bandit framework that, in addition to maximization of the click rate, also considers historical frequency of an article as the “cost” associated with recommending it. It is demonstrated that this approach results in a more balanced distribution and a diverse set of recommended articles. Experiments utilizing a benchmark news dataset demonstrate the trade-off between clicks and diversity of recommended articles.
更多查看译文
关键词
Recommender systems,Contextual bandit,Fairness,Equitable machine learning
AI 理解论文
溯源树
样例
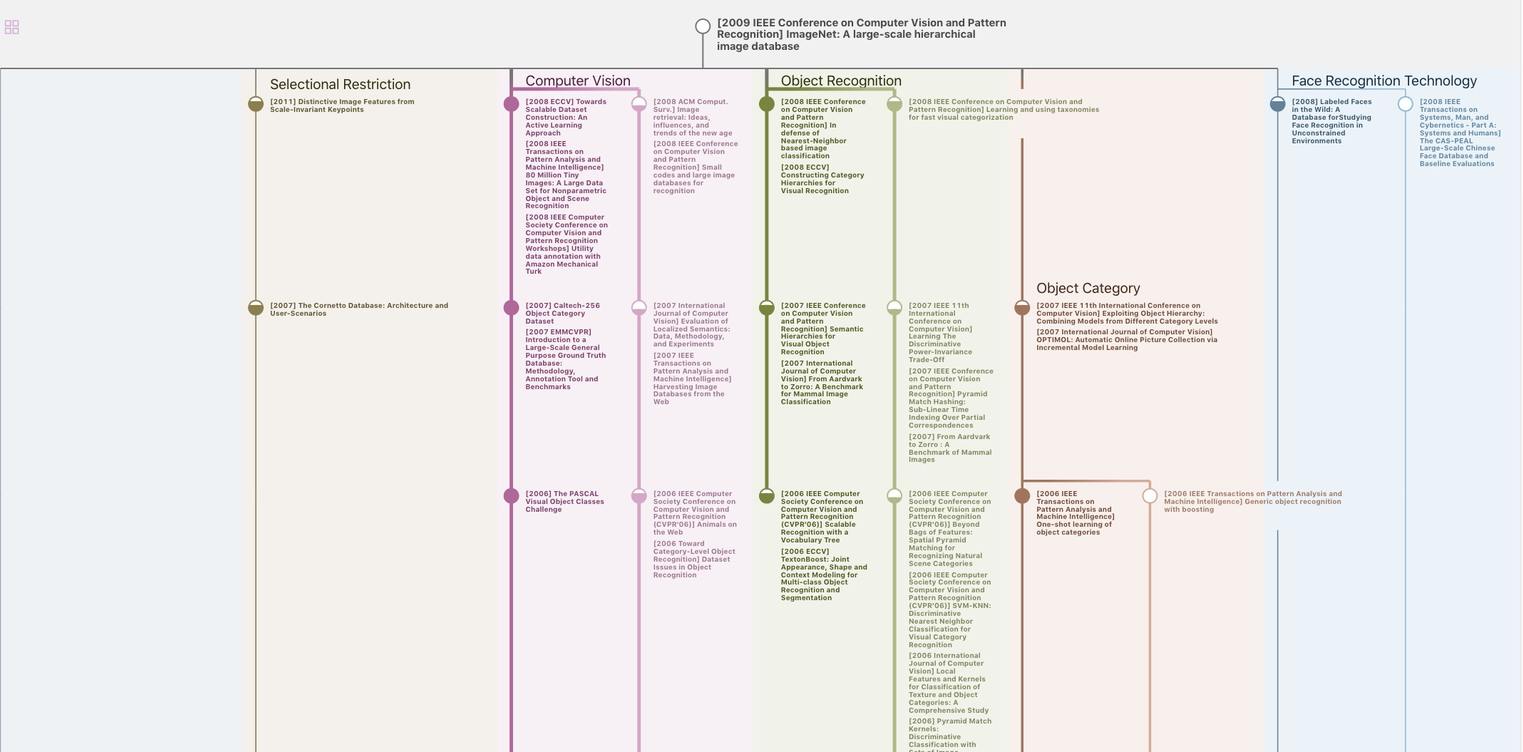
生成溯源树,研究论文发展脉络
Chat Paper
正在生成论文摘要