Machine-learned Behaviour Models for a Distributed Behaviour Repository
ICAART: PROCEEDINGS OF THE 14TH INTERNATIONAL CONFERENCE ON AGENTS AND ARTIFICIAL INTELLIGENCE - VOL 1(2022)
摘要
Dynamically organised multi-agent systems that consist of heterogeneous participants require cooperation to fulfil complex tasks. Such tasks are commonly subdivided into subtasks that have to be executed by individual agents. The necessary teamwork demands coordination of the involved team members. In contrast to typical approaches like agent-centric and organisation-centric views, our solution is based on the task-centric view and thus contains active task components which select agents focusing on their Skills. It enables an encapsulated description of the task flow and its requirements including team cooperation, organisation, and location-independent allocation processes. Besides agent properties that represent syntactical and semantic information, agent behaviours are considered as well. The main contributions of this paper are hyperplane-based machine-learned Behaviour Models that are generated to capture the behaviour and consider the Behaviour Implementations as black boxes. These Behaviour Models are provided by a distributed behaviour repository that enables tasks to actively select fitting Behaviour Implementations. We evaluated our approach based on agents playing chessboard-like games autonomously.
更多查看译文
关键词
Multi-agent Systems, Autonomous Systems, Self Organizing Systems, Agent Models and Architectures, Task Planning and Execution
AI 理解论文
溯源树
样例
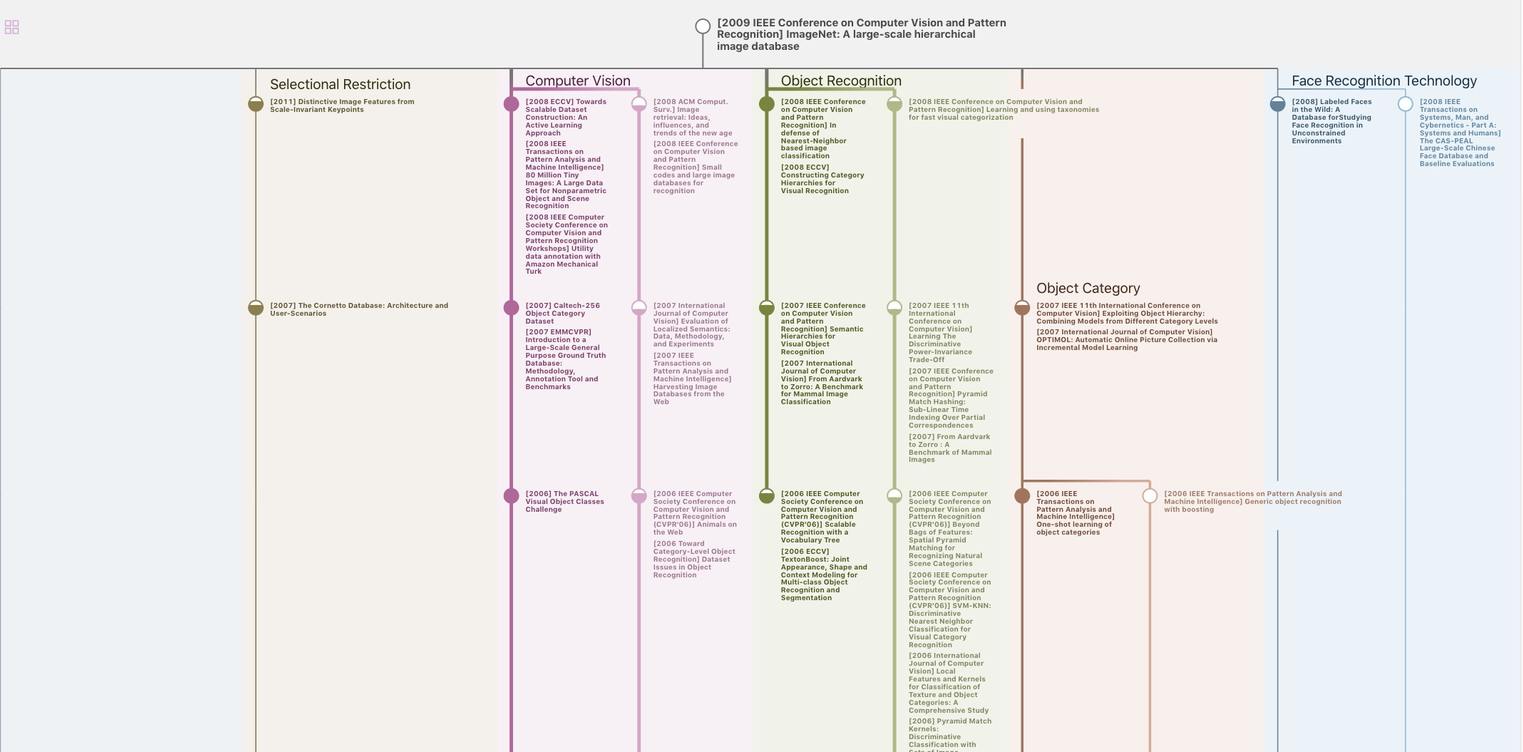
生成溯源树,研究论文发展脉络
Chat Paper
正在生成论文摘要