Pinball loss support vector data description for outlier detection
Applied Intelligence(2022)
摘要
Support vector data description (SVDD) has been widely used in outlier detection. The conventional SVDD employs the hinge loss function and the sphere classifier is decided by only a small amount of data around the sphere surface (namely support vectors), which makes it sensitive to noise and unstable for re-sampling. In this paper, we put forward a novel support vector data description method with pinball loss (pin-SVDD). In our method, all the training data, including those lying inside the sphere, is decisive to the sphere classifier. A small amount of noisy data has little influence on the classifier, which makes our method more robust to noise and achieve scatter minimization in the sphere center. Pin-SVDD has two main merits. (1) Different from the conventional SVDD which employs the hinge loss function and is sensitive to noise, pin-SVDD applies the pinball loss which makes our method more robust to noise and achieve scatter minimization in the sphere center. (2) Distinguished from the existing anti-noise SVDD methods which are based on weight varying and need an extra preprocessing time to generate the instance weights, pin-SVDD does not need preprocessing time and has the same time complexity with the conventional SVDD. Hence, pin-SVDD shows better robustness than the conventional SVDD, but has the same time complexity. The experiment result shows that pin-SVDD has better outlier detection performance than state-of-the-art SVDD-based outlier detection methods, and needs less time on training.
更多查看译文
关键词
Support vector data description,Pinball loss,One-class classification,Outlier detection
AI 理解论文
溯源树
样例
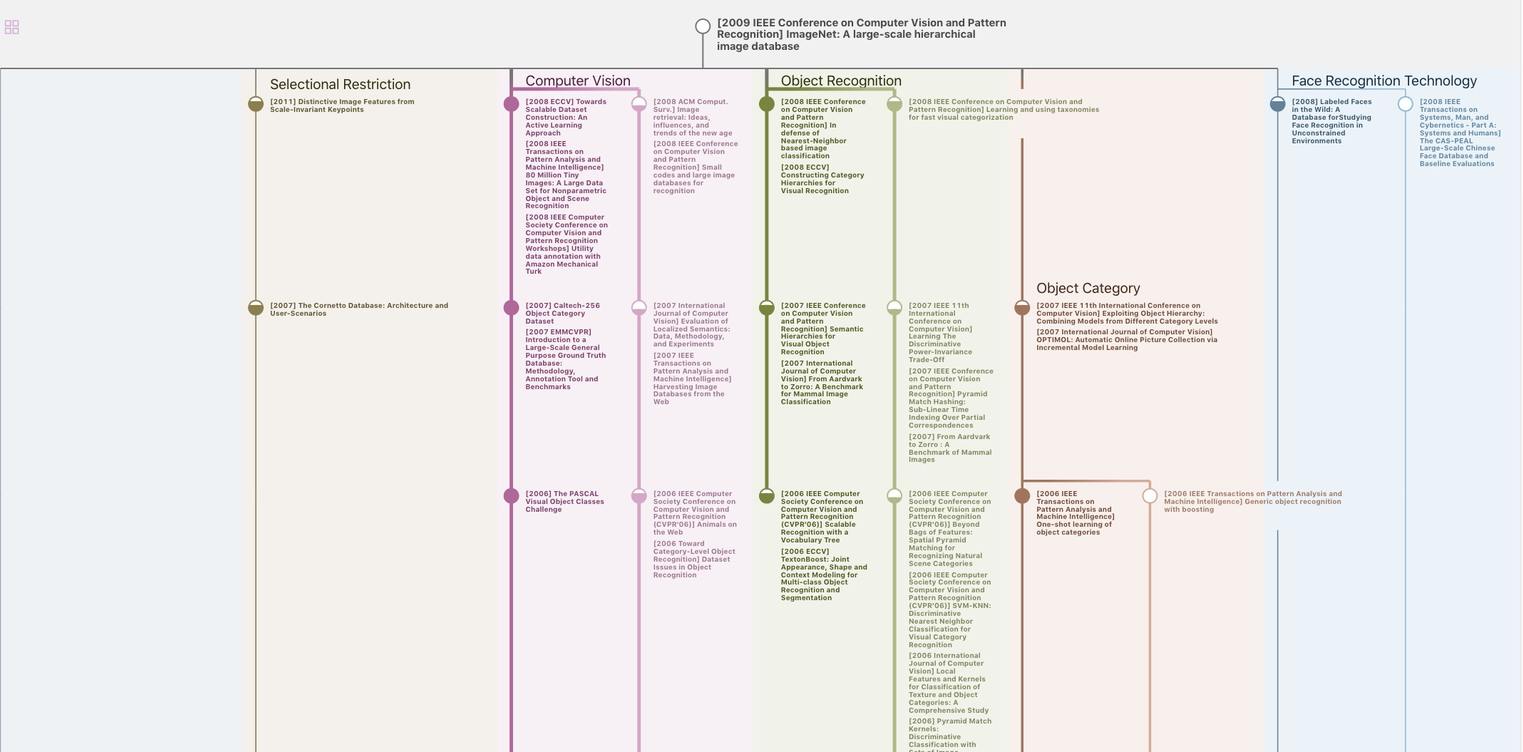
生成溯源树,研究论文发展脉络
Chat Paper
正在生成论文摘要