EmBoost: Embedding Boosting to Learn Multilevel Abstract Text Representation for Document Retrieval
ICAART: PROCEEDINGS OF THE 14TH INTERNATIONAL CONFERENCE ON AGENTS AND ARTIFICIAL INTELLIGENCE - VOL 3(2022)
摘要
Learning hierarchical representation has been vital in natural language processing and information retrieval. With recent advances, the importance of learning the context of words has been underscored. In this paper we propose EmBoost i.e. Embedding Boosting of word or document vector representations that have been learned from multiple embedding models. The advantage of this approach is that this higher order word embedding represents documents at multiple levels of abstraction. The performance gain from this approach has been demonstrated by comparing with various existing text embedding strategies on retrieval and semantic similarity tasks using Stanford Question Answering Dataset (SQuAD), and Question Answering by Search And Reading (QUASAR). The multilevel abstract word embedding is consistently superior to existing solo strategies including Glove, FastText, ELMo and BERT-based models. Our study shows that further gains can be made when a deep residual neural model is specifically trained for document retrieval.
更多查看译文
关键词
Natural Language Processing, Information Retrieval, Deep Learning, Learning Representations, Text Matching
AI 理解论文
溯源树
样例
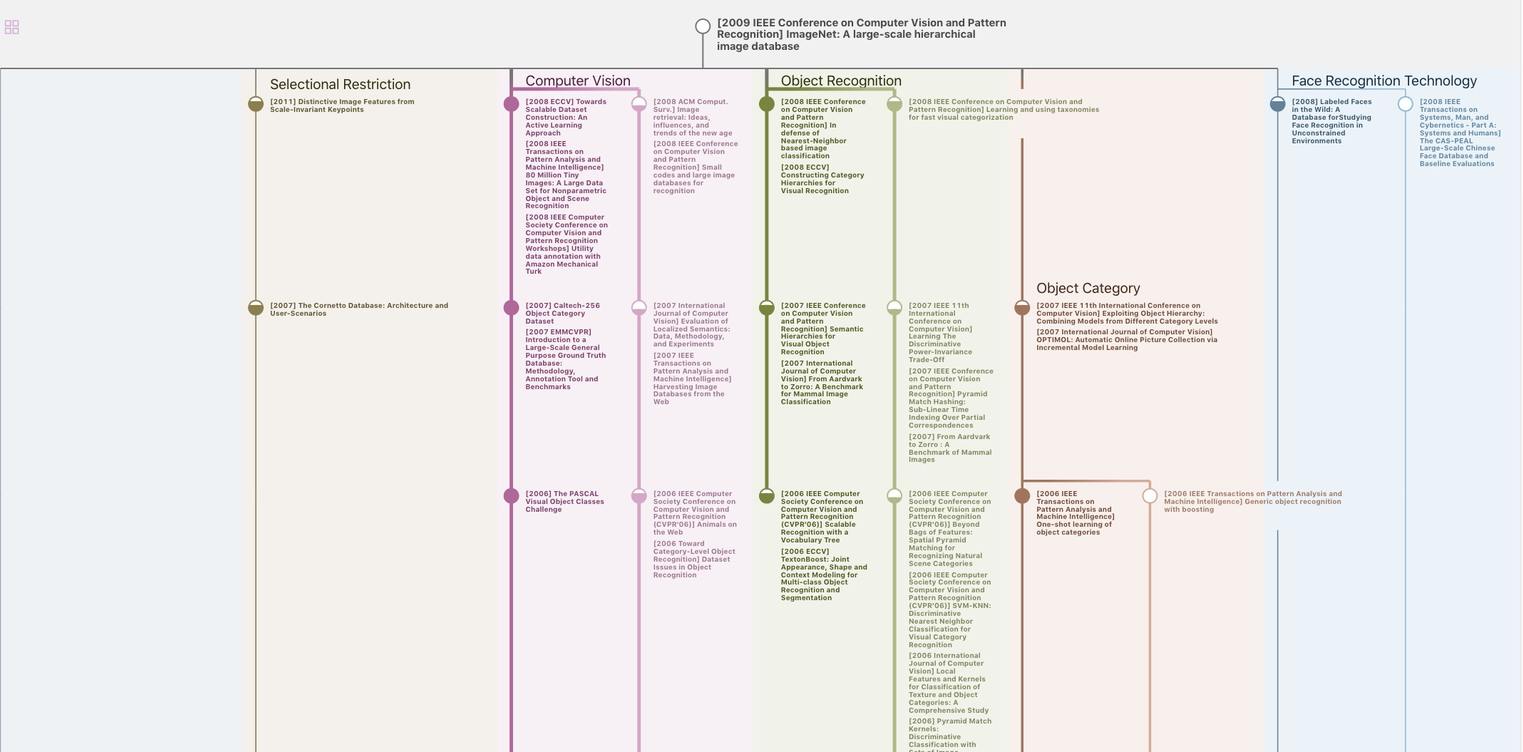
生成溯源树,研究论文发展脉络
Chat Paper
正在生成论文摘要