CGT: Consistency Guided Training in Semi-Supervised Learning
PROCEEDINGS OF THE 17TH INTERNATIONAL JOINT CONFERENCE ON COMPUTER VISION, IMAGING AND COMPUTER GRAPHICS THEORY AND APPLICATIONS (VISAPP), VOL 5(2022)
摘要
We propose a framework, CGT, for semi-supervised learning (SSL) that involves a unification of multiple image-based augmentation techniques. More specifically, we utilize Mixup and CutMix in addition to introducing one-sided stochastically augmented versions of those operators. Moreover, we introduce a generalization of the Mixup operator that regularizes a larger region of the input space. The objective of CGT is expressed as a linear combination of multiple constituents, each corresponding to the contribution of a different augmentation technique. CGT achieves state-of-the-art performance on the SVHN, CIFAR-10, and CIFAR-100 benchmark datasets and demonstrates that it is beneficial to heavily augment unlabeled training data.
更多查看译文
关键词
Semi-Supervised Learning, Consistency Regularization, Data Augmentation
AI 理解论文
溯源树
样例
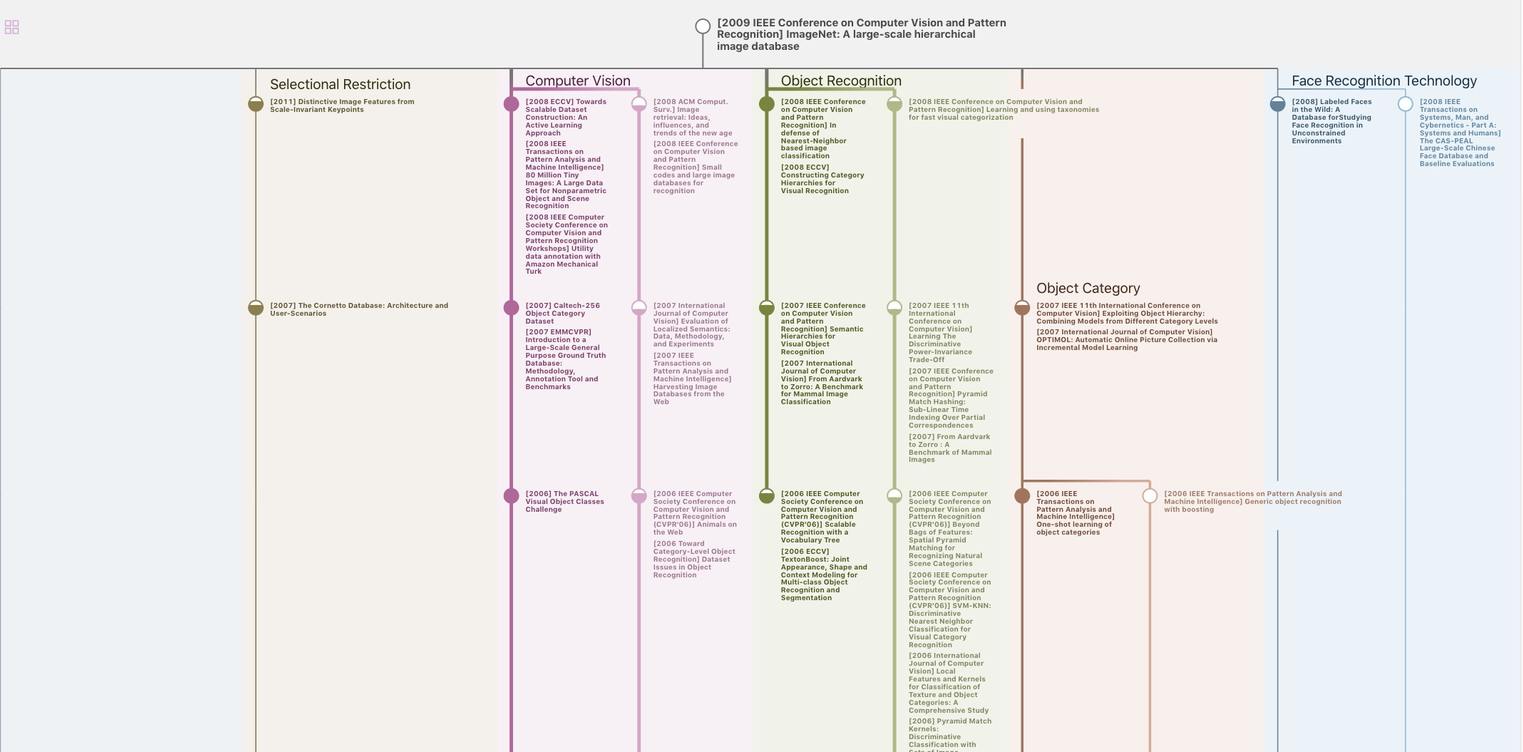
生成溯源树,研究论文发展脉络
Chat Paper
正在生成论文摘要