Wasserstein Graph Auto-Encoder
ALGORITHMS AND ARCHITECTURES FOR PARALLEL PROCESSING, ICA3PP 2021, PT I(2022)
摘要
Conventional Graph Auto-Encoder-based(GAE) minimizes the variational lower bound by Kullback-Leibler divergence in link prediction task and forces a variational posterior distribution for each node to match the prior Gaussian distribution. During the matching process, different variational posterior distributions may intersect. The nodes located at the intersection are subjected to multiple variations which is not suitable for graph reconstruction. We propose Wasserstein Graph Auto-Encoder (WGAE) to minimize the penalty in the Wasserstein distance between the model distribution and the target distribution, which encourages continuous mixed distributions to match the prior distribution. The latent codes of different nodes are far away from each other in the mixed distribution, which is conducive to reconstruction. In addition, gradients are employed to update the mean and variance of the Gaussian distribution in Variational Graph Auto-Encoder(VGAE), which may fall into a kind of local optimization. For each node, the variational posterior distribution is always equal to the prior distribution, and the latent vector is treated as a kind of noise and be ignored by the model. WGAE uses a d-1 dimensional manifold distribution on a d-dimensional hypersphere von Mises-Fisher distribution, controlled by mean direction and concentration parameter. For the prior distribution and posterior distribution, different concentration parameters can be selected and set as hyper-parameters to avoid the local optimum. The von Mises-Fisher distribution also learns a richer topology in non-linear dimensionality reduction. Extensive experiments show that the accuracy of our proposed WGAE in the Cora data set is 2% higher than those of the GAE and VGAE, and 3% higher in the Citeseer data set.
更多查看译文
关键词
Link prediction, Graph auto-encoder, Wasserstein distance, von Mises-Fisher distribution
AI 理解论文
溯源树
样例
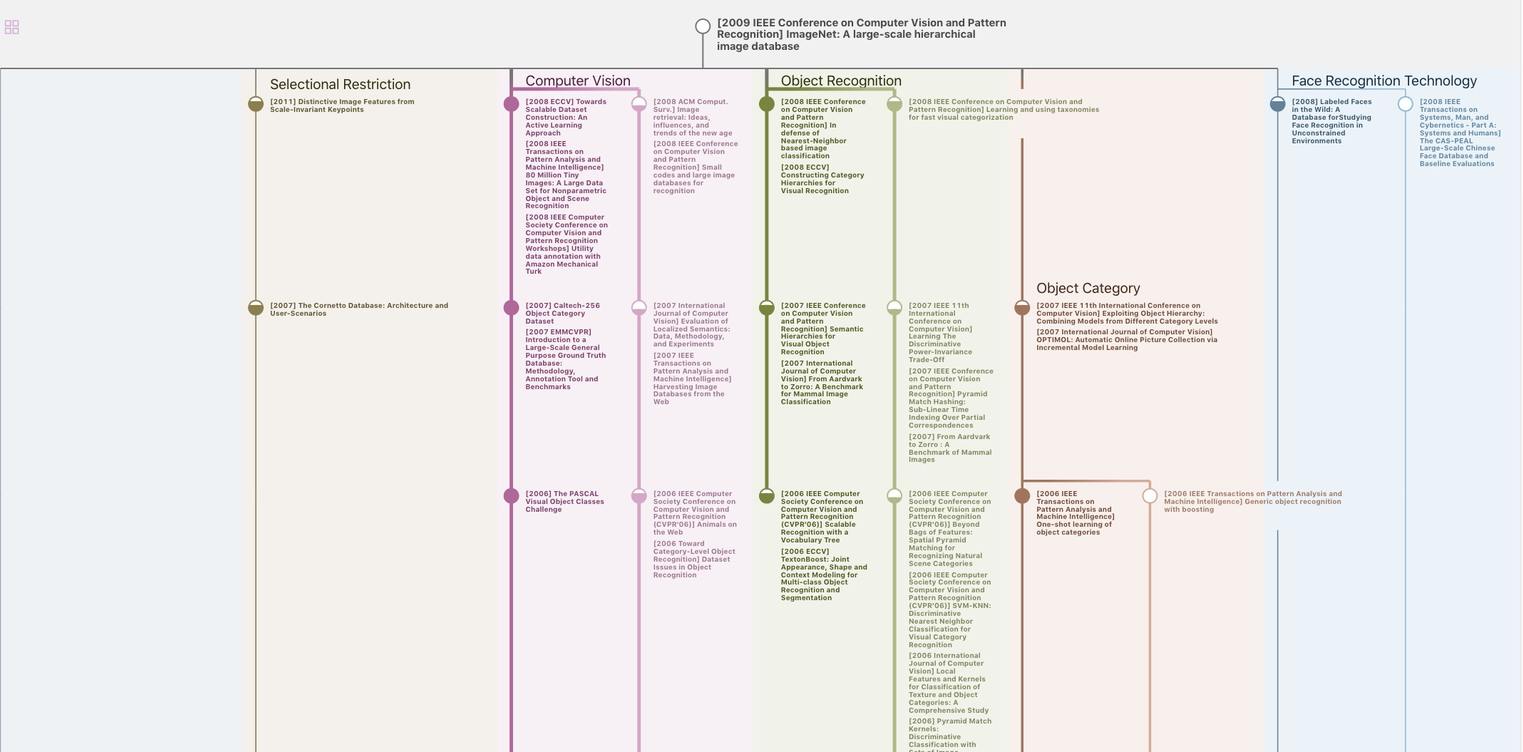
生成溯源树,研究论文发展脉络
Chat Paper
正在生成论文摘要