Classification Performance of RanSaC Algorithms with Automatic Threshold Estimation
PROCEEDINGS OF THE 17TH INTERNATIONAL JOINT CONFERENCE ON COMPUTER VISION, IMAGING AND COMPUTER GRAPHICS THEORY AND APPLICATIONS (VISAPP), VOL 5(2022)
摘要
The RANdom SAmpling Consensus method (RanSaC) is a staple of computer vision systems and offers a simple way of fitting parameterized models to data corrupted by outliers. It builds many models from small sets of randomly selected data points, and then scores them to keep the best. The original scoring function is the number of inliers, points that fit the model up to some tolerance. The threshold that separates inliers from outliers is data- and model-dependent, and estimating the quality of a RanSaC method is difficult as ground truth data is scarce or not quite reliable. To remedy that, we propose a data generation method to create at will ground truths both realistic and perfectly reliable. We then compare the RanSaC methods that simultaneously fit a model and estimate an appropriate threshold. We measure their classification performance on those semi-synthetic feature correspondence pairs for homography, fundamental, and essential matrices. Whereas the reviewed methods perform on par with the RanSaC baseline for standard cases, they do better in difficult cases, maintaining over 80 % precision and recall. The performance increase comes at the cost of running time and analytical complexity, and unexpected failures for some algorithms.
更多查看译文
关键词
Multi-View Stereo, Structue-from-Motion, RanSaC, Semi-synthetic Dataset, Benchmark
AI 理解论文
溯源树
样例
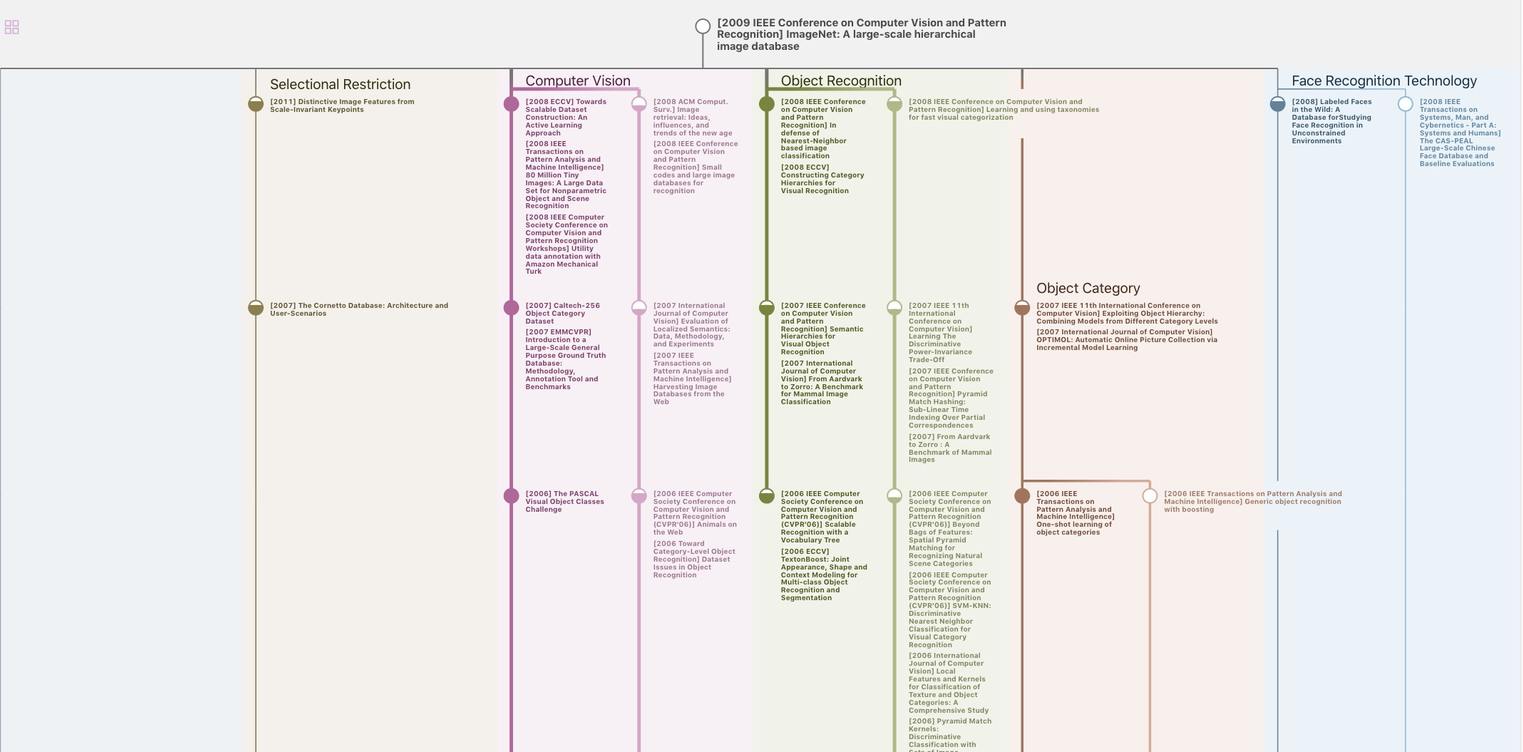
生成溯源树,研究论文发展脉络
Chat Paper
正在生成论文摘要