Contextual anomaly detection for high-dimensional data using Dirichlet process variational autoencoder
IISE TRANSACTIONS(2023)
摘要
Due to recent advances in sensing technologies, response measurements of various sensors are frequently used for system monitoring purposes. However, response data are often affected by some contextual variables, such as equipment settings and time, resulting in different patterns, even when the system is in the normal state. In this case, anomaly detection methods that do not consider contextual variables may be unable to distinguish between abnormal and normal patterns of the response data affected by the contextual variables. Motivated by this problem, we propose a method for contextual anomaly detection, particularly in the case where the response and contextual variables are both high-dimensional and complex. The proposed method is based on Variational AutoEncoders (VAEs), which are neural-network-based generative models suitable for modeling high-dimensional and complex data. The proposed method combines two VAEs: one for response variables and the other for contextual variables. Specifically, in the latent space of the VAE for contextual variables, we model the latent variables using a Dirichlet process Gaussian mixture model. Consequently, the effects of the contextual variables can be modeled using several clusters, each representing a different contextual environment. The latent contextual variables are then used as additional inputs to the other VAE's decoder for reconstructing response data from their latent representations. We then detect the anomalies based on the negative reconstruction loss of a new response observation. The effectiveness of the proposed method is demonstrated using several benchmark datasets and a case study based on a global tire company.
更多查看译文
关键词
Contextual anomaly detection,Dirichlet process mixture model,high-dimensional data,variational autoencoder
AI 理解论文
溯源树
样例
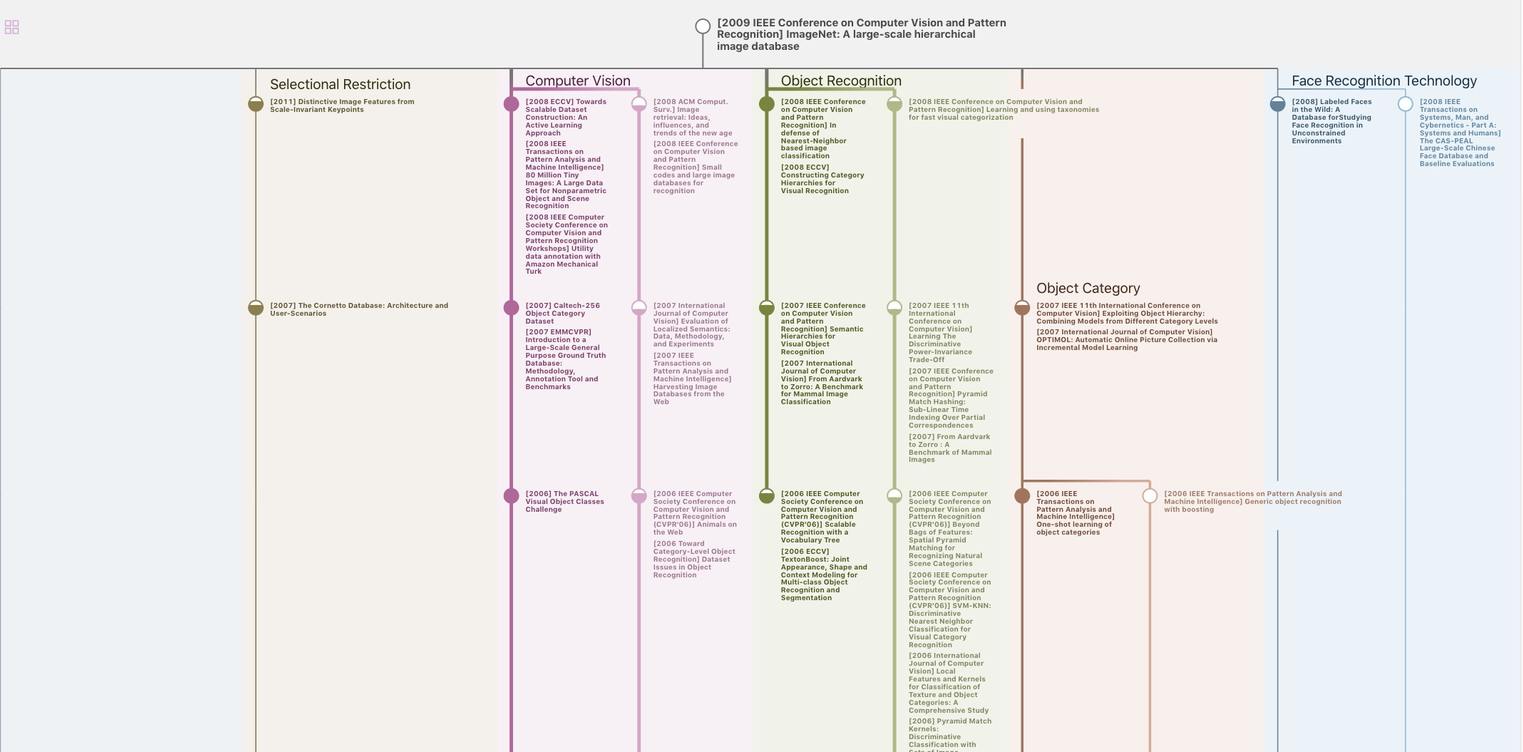
生成溯源树,研究论文发展脉络
Chat Paper
正在生成论文摘要