Buildings Extraction from Historical Topographic Maps via a Deep Convolution Neural Network
PROCEEDINGS OF THE 17TH INTERNATIONAL JOINT CONFERENCE ON COMPUTER VISION, IMAGING AND COMPUTER GRAPHICS THEORY AND APPLICATIONS (VISAPP), VOL 5(2022)
摘要
The cartographic representation is static by definition. Therefore, reading a map of the past can provide information, which corresponds to the accuracy, technology, as well as scientific knowledge of the time of their creation. Digital technology enables the current researcher to "copy" a historical map and "transcribe" it to today. In this way, a cartographic reduction from the past to the present is possible, with parallel visualization of new information (historical geodata), which the researcher has at his disposal, in addition to the background. In this work a deep learning approach is presented for the extraction of buildings within historical topographic maps. A deep convolution neural network based on the U-Net architecture is trained by a large number of images patches in a deep image-to-image regression mode in order to effectively isolate the buildings from the topographic map while ignoring other surrounding or overlapping information like texts or other irrelevant geospatial features. Several experimental scenarios on a historical census topographic map investigate the applicability of the method under various patch sizes as well as patch sampling methods. The so far results show that the proposed method delivers promising outcomes in terms of building detection accuracy.
更多查看译文
关键词
Building Detection, Historical Maps, Deep Learning
AI 理解论文
溯源树
样例
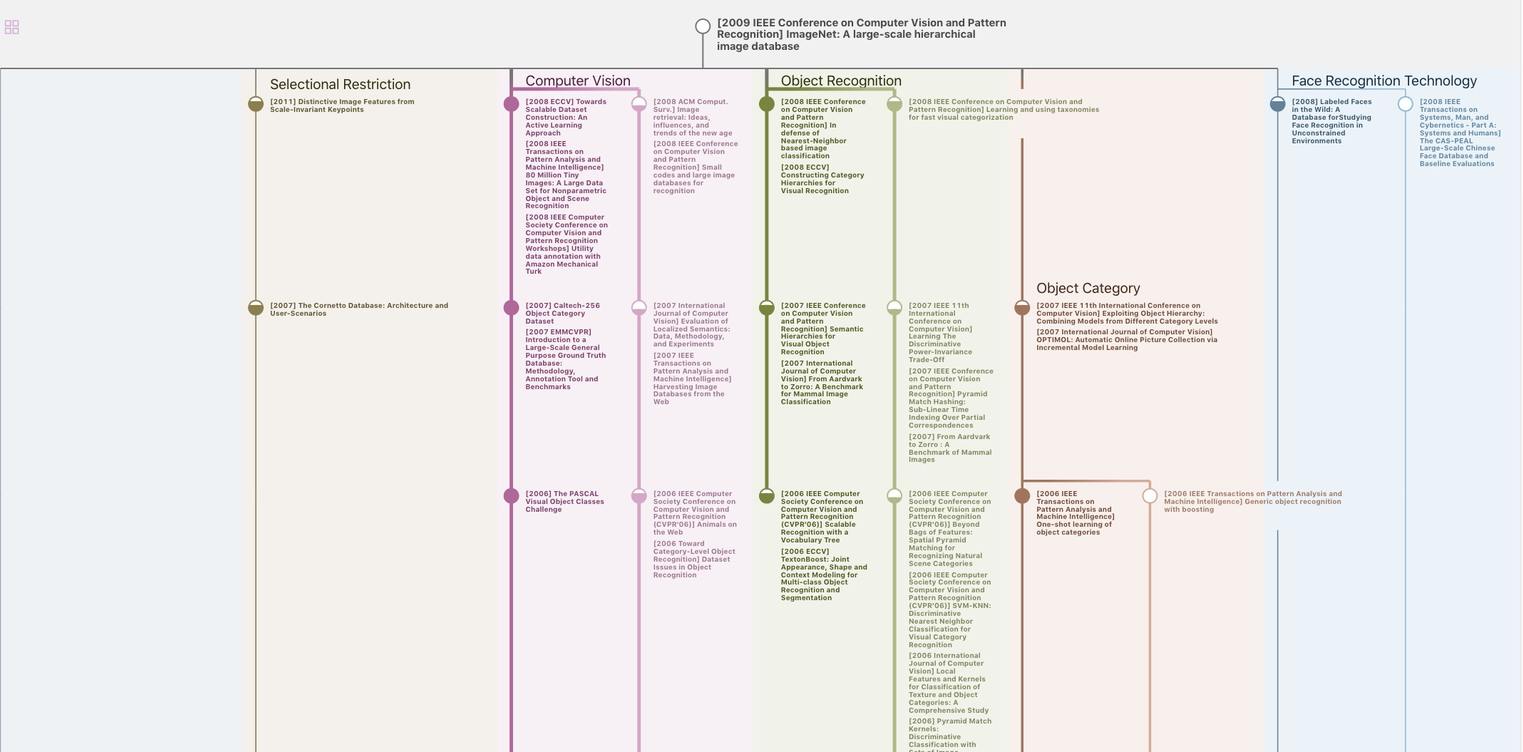
生成溯源树,研究论文发展脉络
Chat Paper
正在生成论文摘要