Data-driven, early-season forecasts of block sugarcane yield for precision agriculture
Field Crops Research(2022)
摘要
The Australian sugar industry is heavily regulated for on-farm inputs due to nutrient-rich runoff which flows into the Great Barrier Reef. Variable rate application of N fertiliser allows sugarcane (Saccharum spp.) growers to optimise the use of limited resources and match crop needs in space and time. This study outlines an approach and the merits of a data-driven, site- and season-specific forecast of yield at the block (field) resolution, by using publicly available spatio-temporal data such as satellite imagery, radiometrics, weather, and terrain attributes, in tandem with commonly available grower and mill data, namely yield, ratoons, harvest dates. The study used harvest data collected from two sugarcane properties (380 ha) in the Isis District of Queensland, between 2007 and 2018. Yield forecasts were produced with random forest models at two key management points in the season; early- (1 December) and late-season (1 June). Using a robust leave-one-season-out cross-validation technique, sugarcane yield could be forecasted early-season with a Lin’s concordance correlation coefficient (LCCC) of 0.61 and root-mean-square error (RMSE) of 32.1 t ha-1, with an improvement later in the season with 0.63 LCCC and 30.9 t ha-1 RMSE. Accurate early-season forecasts of yield at fine spatial supports offers growers the opportunity to make better-informed decisions regarding crop nutrition. This leads to more targeted and accurate applications of fertiliser, improving on-farm profitability, and critically decreasing off-site environmental damage to the Great Barrier Reef. The cost-effective, widely-applicable and scalable forecasting approach described in this case study could be expanded to a regional or industry scale, providing benefits to all industry stakeholders.
更多查看译文
关键词
6ES,DYP,N,LOSO,LCCC,RMSE
AI 理解论文
溯源树
样例
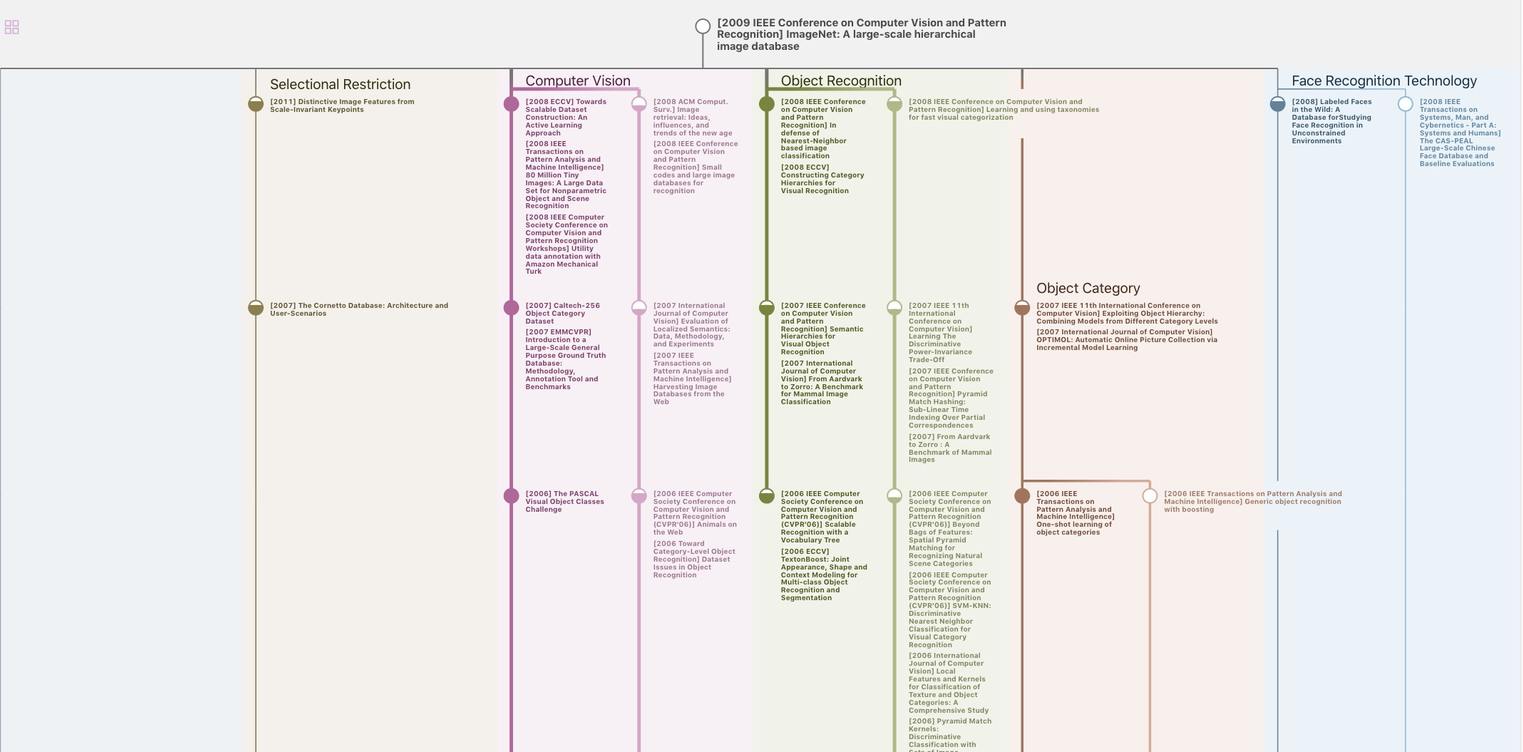
生成溯源树,研究论文发展脉络
Chat Paper
正在生成论文摘要