Using machine learning algorithms to multidimensional analysis of subjective thermal comfort in a library
BUILDING AND ENVIRONMENT(2022)
摘要
The thermal comfort in public buildings is affected by multiple psychological and physical factors. A deep understanding of these factors is necessary for intelligent ventilation control and architectural design. In this study, it was quantified the physiological and psychological parameters of the occupants in a library situated in Changsha using a questionnaire. Then, with the use of Principal Component Analysis (PCA), the dimensionality of the database was reduced. We found that the Zero-mean and unit variance projection is better than the Zeromean projection, and 43-dimensional data can replace more than 90% of the original 61-dimensional data. Machine learning algorithms were used to analyze the results after PCA, which showed that the effect of thermal comfort on emotions is greater than that of emotions on thermal comfort. In addition, the performance of six machine learning algorithms (Linear Regression (LR), Linear Discriminant Analysis (LDA), K-Nearest Neighbors (KNN), Classification and Regression Trees (CART), Gaussian Naive Bayes (NB), and Support Vector Machine (SVM)) were compared. It is found that the predicted results of LDA were more accurate, and other algorithms showed different performances in different cases. These findings can contribute to the study of the subjective and objective feelings of indoor thermal comfort in public buildings, thereby guiding architectural design, intelligent control of ventilation systems, and realizing human-building interaction interfaces.
更多查看译文
关键词
Thermal comfort, Machine learning, Principal component analysis, Multidimensional psychological parameters, Public building
AI 理解论文
溯源树
样例
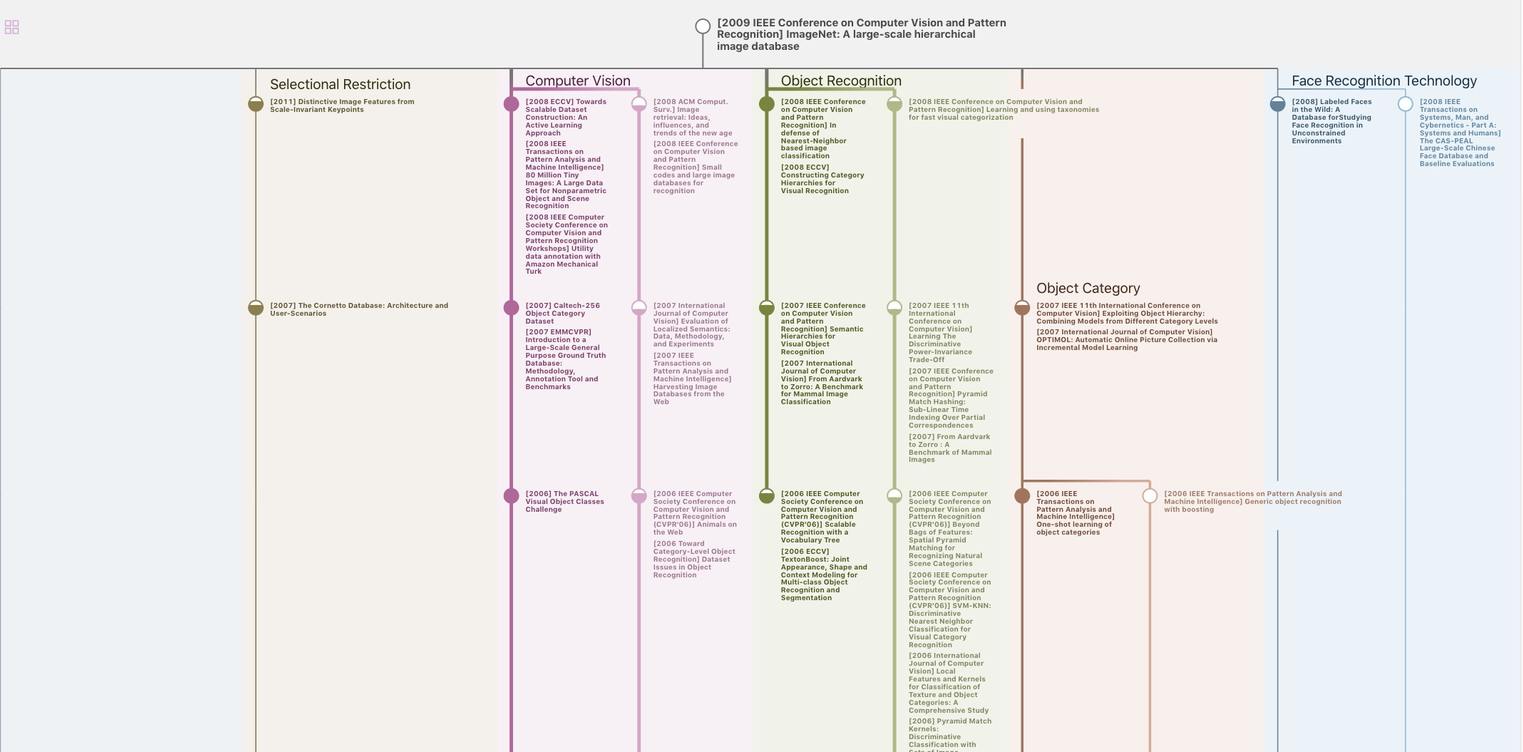
生成溯源树,研究论文发展脉络
Chat Paper
正在生成论文摘要