Opportunities and Limitations of in-Memory Multiply-and-Accumulate Arrays
2021 IEEE Microelectronics Design & Test Symposium (MDTS)(2021)
摘要
In-memory computing is a promising solution to solve the memory bottleneck problem which becomes increasingly unfavorable in modern machine learning systems. In this paper, we introduce an architecture of random access memory (RAM) incorporating deep learning inference abilities. Due to the digital nature of this design, the architecture can be applied to a variety of commercially available volatile and non-volatile memory technologies. We also introduce a multi-chip architecture to accommodate for varying network sizes and to maximize parallel computing ability. Moreover, we discuss the opportunities and limitations of in-memory computing as future neural networks scale, in terms of power, latency and performance. To do so, we applied this architecture to various prevalent neural networks, e.g. Artificial Neural Network (ANN), Convolutional Neural Network (CNN) and Transformer Network and compared the results.
更多查看译文
关键词
In-memory computing,deep neural network,deep learning,DRAM,Transformer,memory bottleneck
AI 理解论文
溯源树
样例
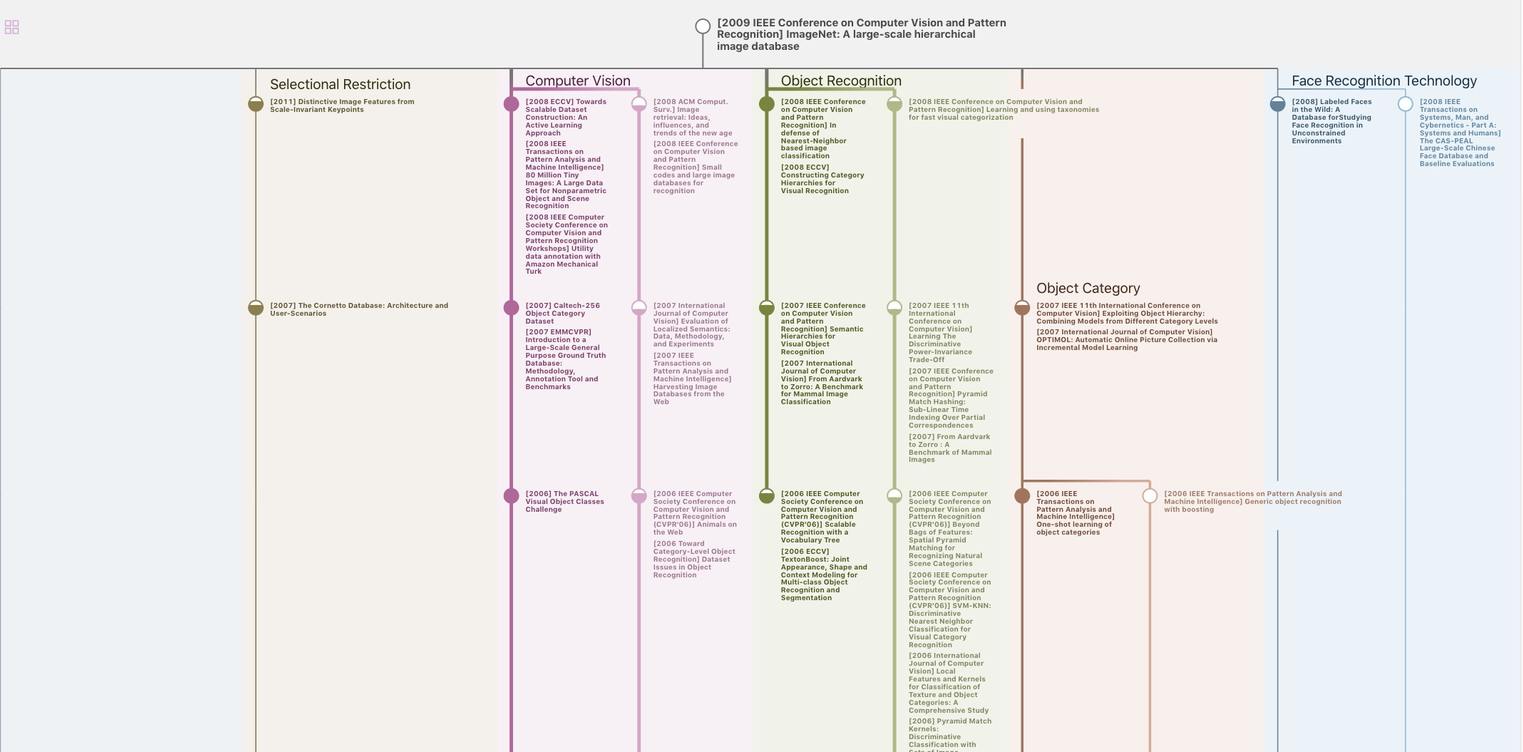
生成溯源树,研究论文发展脉络
Chat Paper
正在生成论文摘要