Extended recommendation-by-explanation
USER MODELING AND USER-ADAPTED INTERACTION(2022)
摘要
Studies have shown that there is an intimate connection between the process of computing recommendations and the process of generating corresponding explanations and that this close relationship may lead to better recommendations for the user. However, to date, most recommendation explanations are post hoc rationalizations; in other words, computing recommendations and generating corresponding explanations are two separate and sequential processes. There is, however, recent work unifies recommendation and explanation, using an approach that is called Recommendation-by-Explanation ( r-by-e ). In r-by-e , the system constructs an explanation, a chain of items from the user’s profile, for each candidate item; then, it recommends those candidate items that have the best explanations. However, the way it constructs and selects chains is relatively simple, and it considers only one way of representing item’s elements—in terms of their features. In this article, we extend r-by-e . We present a number of different ways of generating chains from a user’s profile. These methods mainly differ in their item representations (i.e. whether using item elements as features or neighbours) and in the weighting schemes that they use to generate the chains. We also explore r-by-e ’s approach to chain selection, allowing the system to choose whether to cover more aspects of the candidate item or the user profile. We compare the extended versions with corresponding classic content-based methods on two datasets that mainly differ on their item feature sets. We find that the versions of r-by-e that make explicit use of item features have several advantages over the ones that use neighbours, and the empirical comparison shows that one of these versions—the one that assigns weights to the item features based on their importance to that item—is also the best in terms of recommendation accuracy, diversity, and surprise, while still generating chains whose lengths are manageable enough to be interpretable by users. It also obtains the best survey responses for its recommendations and corresponding explanations in a trial with real users.
更多查看译文
关键词
Explanation, Recommendation, Sentiments, User trials
AI 理解论文
溯源树
样例
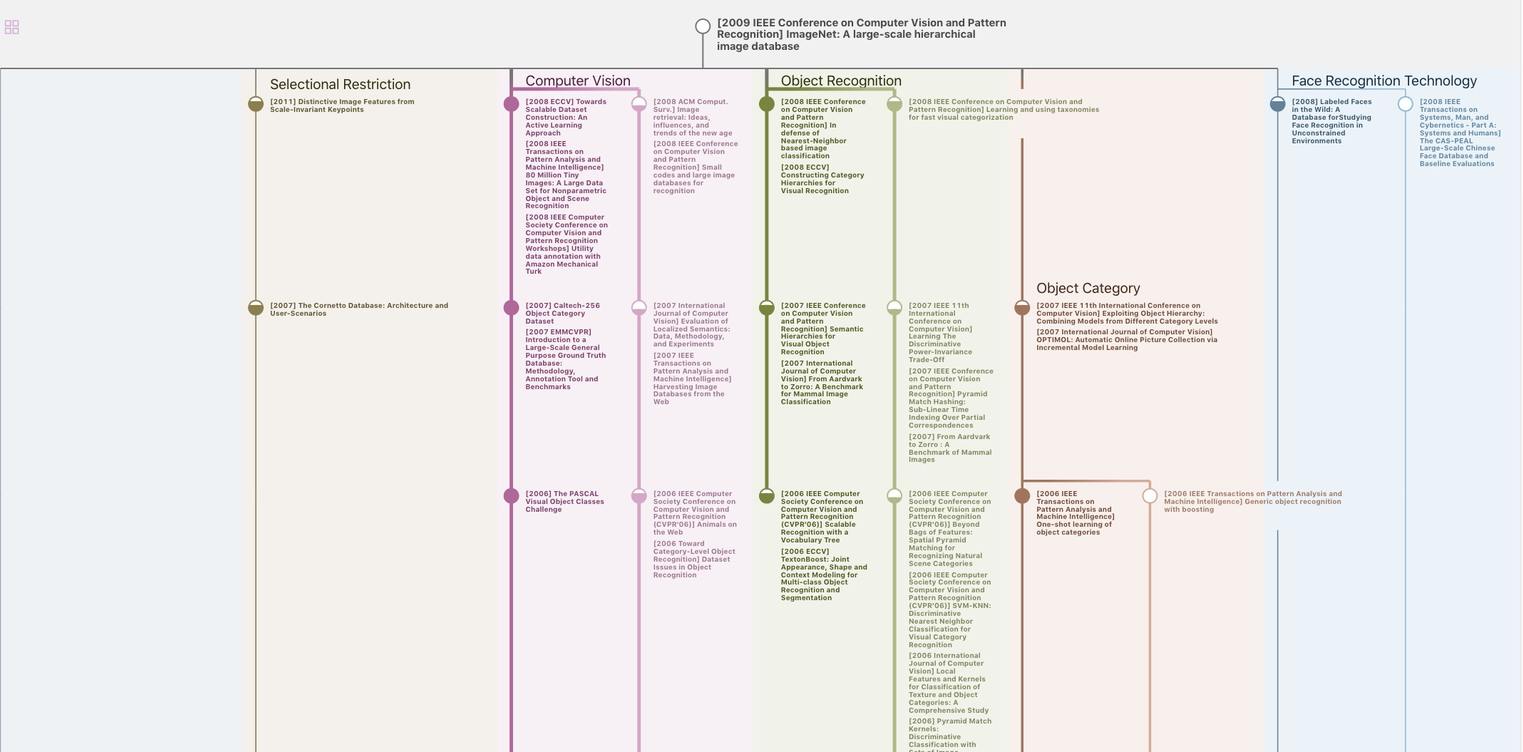
生成溯源树,研究论文发展脉络
Chat Paper
正在生成论文摘要