Data-Driven Predictive Electrochemical Behavior of Lignin-Based Supercapacitors via Machine Learning
ENERGY & FUELS(2022)
摘要
Plant-derived lignin-based supercapacitors have shown strong potential as light and flexible electronic devices. To design a safe, reliable, and efficient supercapacitor, it is important to predict its electrochemical performance. To date, there is no reliable theoretical model to serve the purpose. In this work, four machine learning algorithms are developed and compared to predict the specific capacitance variation for lignin supercapacitors. Specifically, linear regression (LR), support vector machine (SVM), decision tree (DT), and artificial neural network (ANN) models are analyzed. Specific capacitance variation obtained by cyclic charge-discharge tests is modeled as a function of weight percentages of material constituents (lignin, nickel tungstate nanoparticles, and polyvinylidene fluoride) and the cycle number. The accuracy of the models is ranked as LR < SVM < DT < ANN and validated further using the F-test. The superior model fit of ANN shows the highest accuracy and applicability with excellent robustness. ANN can generalize the learned specific capacitance variation to material ratios excluded in the training set. This work provides an understanding of different machine learning techniques for predicting the specific capacitance variation and retention of lignin-based supercapacitors, demonstrating the strong potential of ANN to be used as a predictive tool to aid in supercapacitor optimization.
更多查看译文
AI 理解论文
溯源树
样例
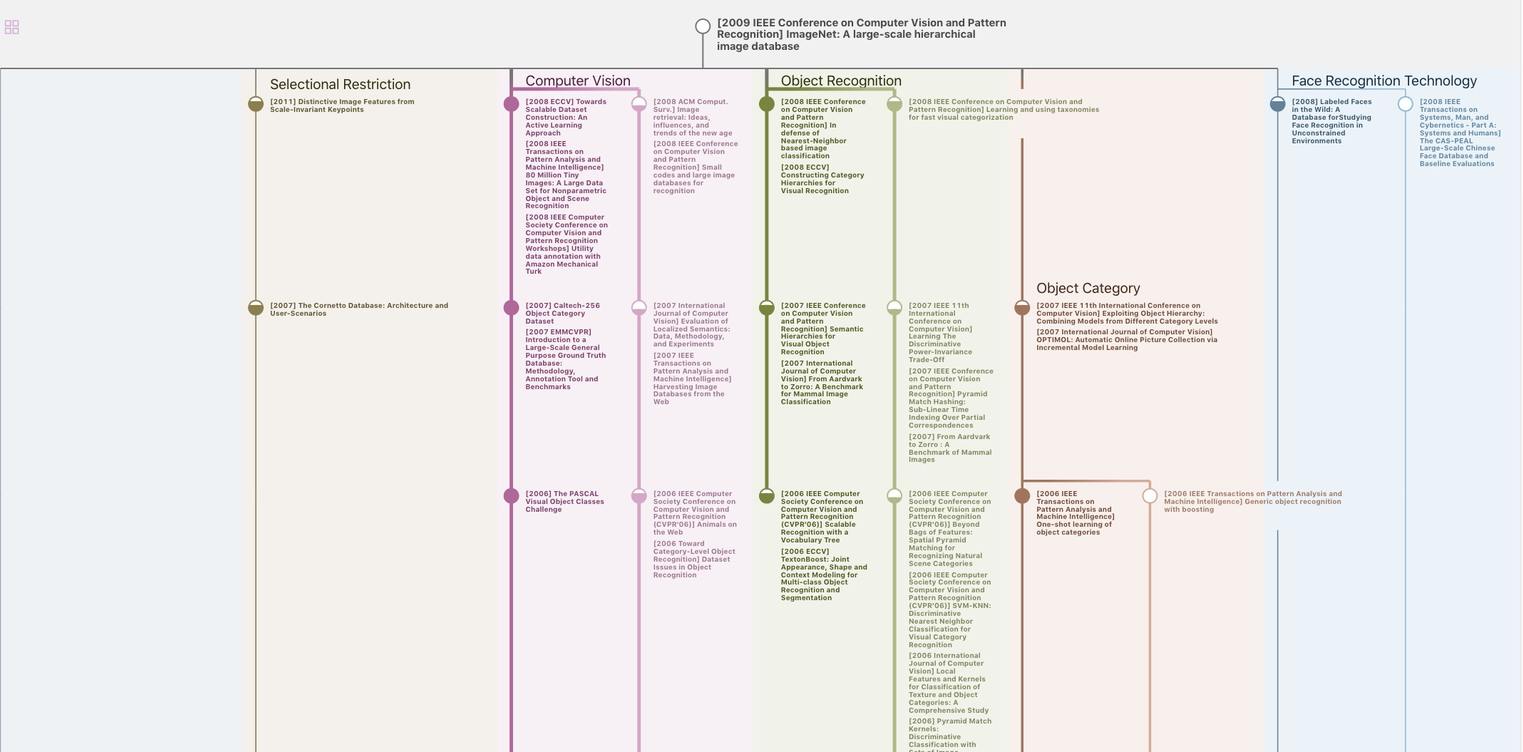
生成溯源树,研究论文发展脉络
Chat Paper
正在生成论文摘要