Reduction of estimation risk in optimal portfolio choice using redundant constraints
International Review of Financial Analysis(2021)
摘要
It is well known that when the moments of the distribution governing returns are estimated from sample data, the out-of-sample performance of the optimal solution of a mean–variance (MV) portfolio problem deteriorates as a consequence of the so-called “estimation risk”. In this document we provide a theoretical analysis of the effects caused by redundant constraints on the out-of-sample performance of optimal MV portfolios. In particular, we show that the out-of-sample performance of the plug-in estimator of the optimal MV portfolio can be improved by adding any set of redundant linear constraints. We also illustrate our findings when risky assets are equally correlated and identically distributed. In this specific case, we report an emerging trade-off between diversification and estimation risk and that the allocation of estimation risk across portfolios forming the optimal solution changes dramatically in terms of number of assets and correlations.
更多查看译文
关键词
G23
AI 理解论文
溯源树
样例
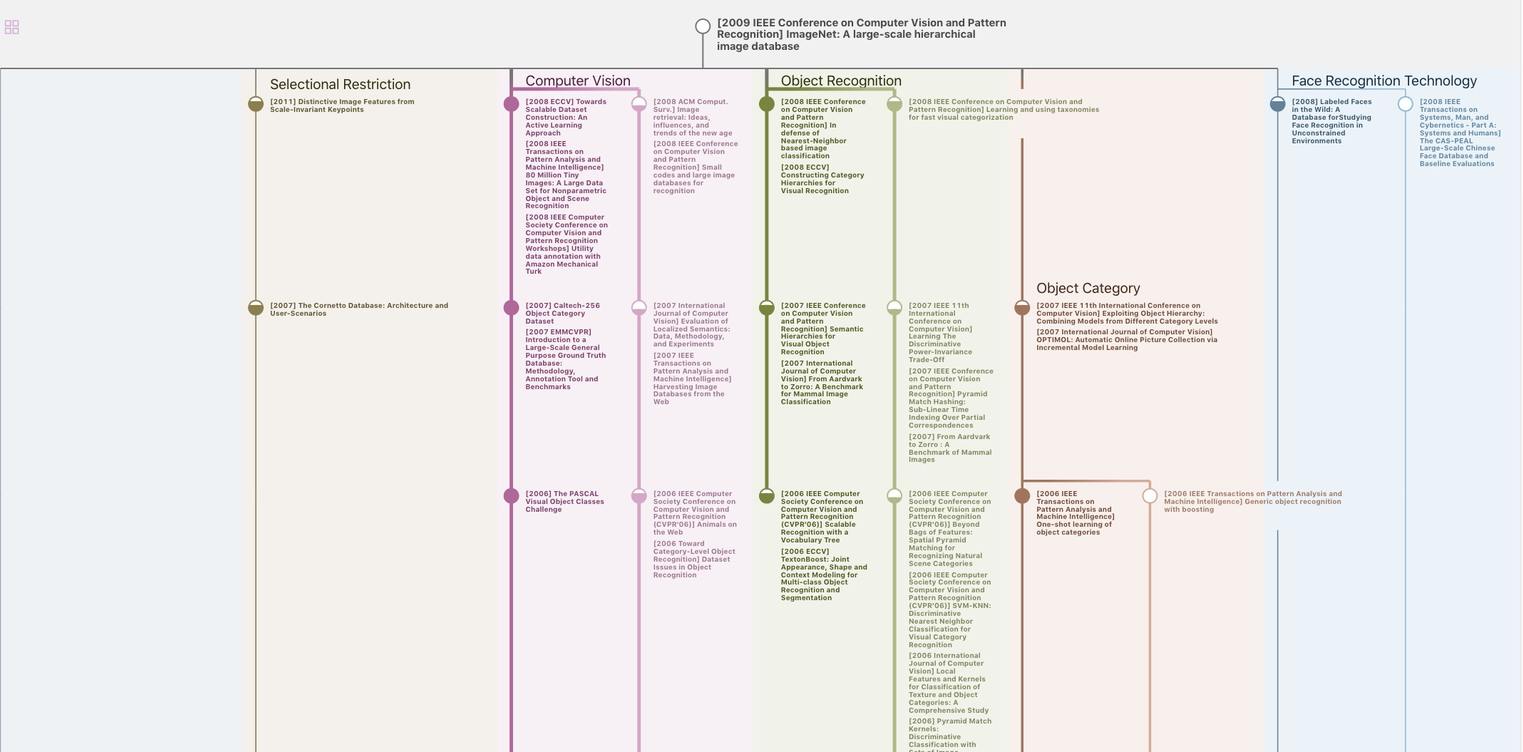
生成溯源树,研究论文发展脉络
Chat Paper
正在生成论文摘要