Fault diagnosis of wind turbine bearing using a multi-scale convolutional neural network with bidirectional long short term memory and weighted majority voting for multi-sensors
RENEWABLE ENERGY(2022)
摘要
In order to solve the problems of insufficient extrapolation of intelligent models for the fault diagnosis of bearings in real wind turbines, this study has developed a multi-scale convolutional neural network with bidirectional long short term memory (MSCNN-BiLSTM) model for improving the generalization abilities under complex working and testing environments. A weighted majority voting rule has been proposed to fuse the information from multi-sensors for improving the extrapolation of multisensory diagnosis. The superiority of the MSCNN-BiLSTM model is examined through experimental data. The results indicate that the MSCNN-BiLSTM model has 97.12% mean F1 score, which is higher than existing advanced methods. Real wind turbine dataset and an experimental dataset are used to demonstrate the effectiveness of the weighted majority voting rule for multisensory diagnosis. The results present that the diagnosis result of the MSCNN-BiLSTM model with weighted majority voting rule is higher respectively 1.32% and 5.7% than the model with traditional majority voting or fusion of multisensory information in feature-level. (c) 2021 Published by Elsevier Ltd.
更多查看译文
关键词
Bearing, Wind turbine, Convolutional neural network, Fault diagnosis, Information fusion
AI 理解论文
溯源树
样例
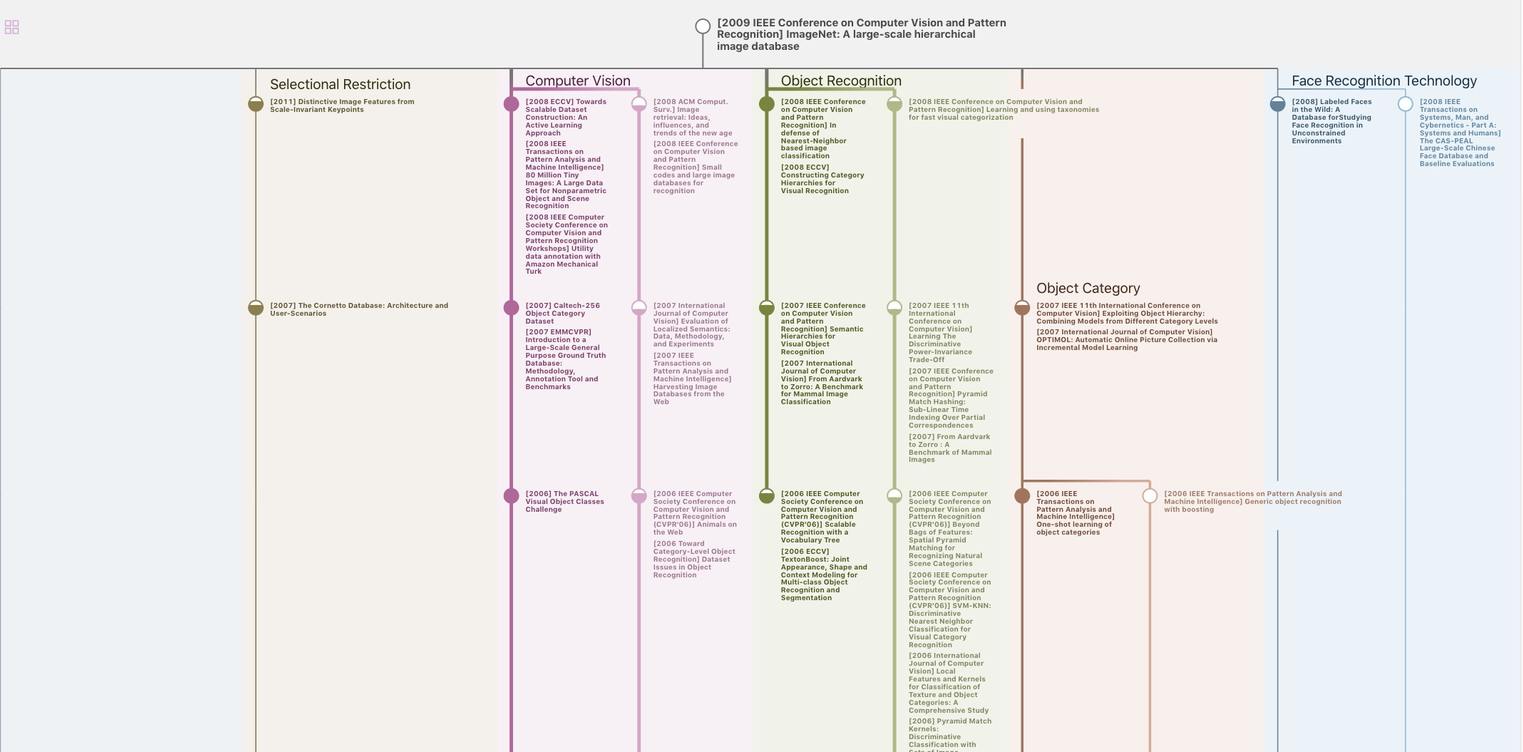
生成溯源树,研究论文发展脉络
Chat Paper
正在生成论文摘要