Torch-NILM: An Effective Deep Learning Toolkit for Non-Intrusive Load Monitoring in Pytorch
ENERGIES(2022)
摘要
Non-intrusive load monitoring is a blind source separation task that has been attracting significant interest from researchers working in the field of energy informatics. However, despite the considerable progress, there are a very limited number of tools and libraries dedicated to the problem of energy disaggregation. Herein, we report the development of a novel open-source framework named Torch-NILM in order to help researchers and engineers take advantage of the benefits of Pytorch. The aim of this research is to tackle the comparability and reproducibility issues often reported in NILM research by standardising the experimental setup, while providing solid baseline models by writing only a few lines of code. Torch-NILM offers a suite of tools particularly useful for training deep neural networks in the task of energy disaggregation. The basic features include: (i) easy-to-use APIs for running new experiments, (ii) a benchmark framework for evaluation, (iii) the implementation of popular architectures, (iv) custom data loaders for efficient training and (v) automated generation of reports.
更多查看译文
关键词
non-intrusive load monitoring, energy disaggregation, nilm, deep learning, pytorch
AI 理解论文
溯源树
样例
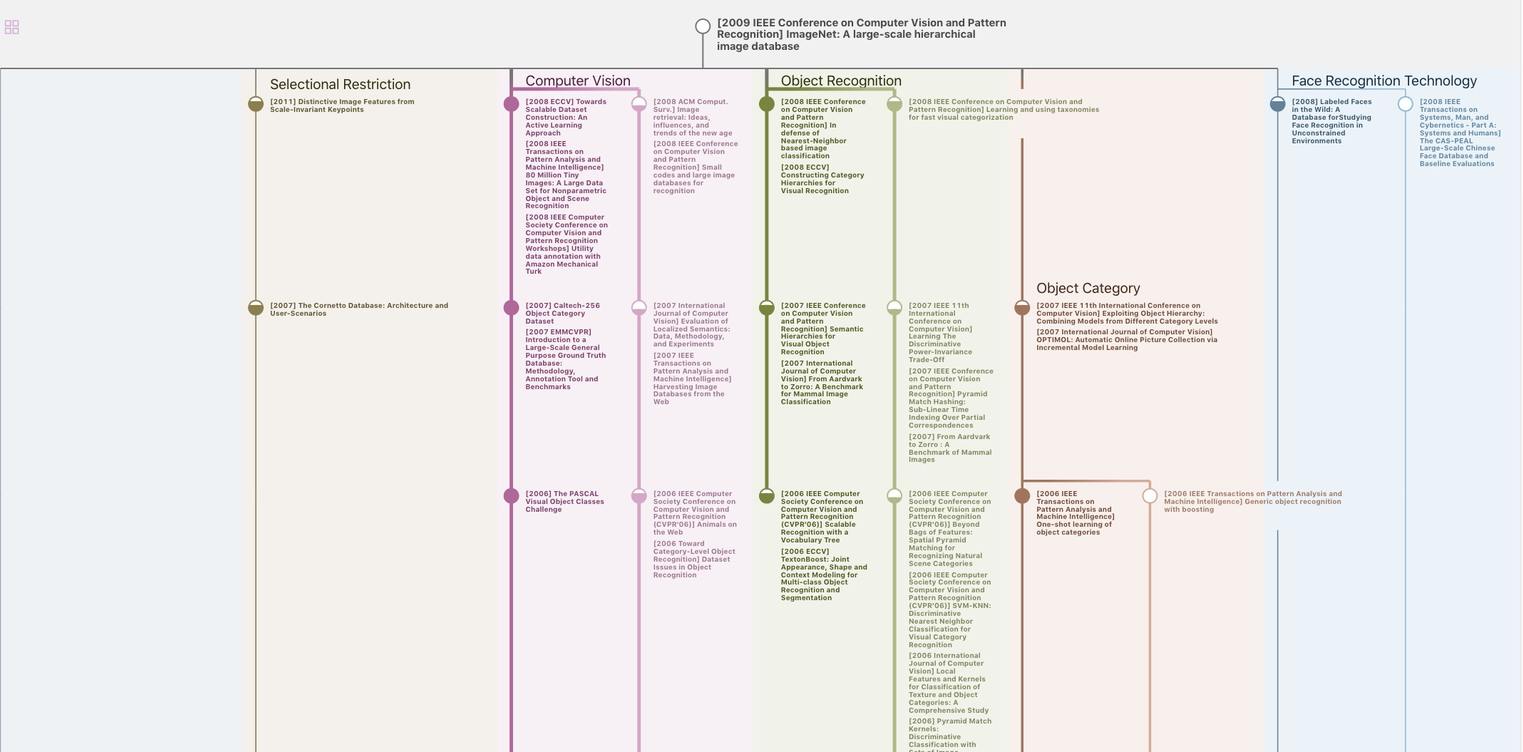
生成溯源树,研究论文发展脉络
Chat Paper
正在生成论文摘要