Development of a High-Latitude Convection Model by Application of Machine Learning to superDARN Observations
SPACE WEATHER-THE INTERNATIONAL JOURNAL OF RESEARCH AND APPLICATIONS(2022)
摘要
A new model of northern hemisphere high-latitude convection derived using machine learning (ML) is presented. The ML algorithm random forests regression was applied to a database of velocities derived from the Super Dual Auroral Radar Network (SuperDARN) observations processed with the potential mapping technique, Map-Potential (Ruohoniemi & Baker, 1998, https://doLorg/10.1029/98ja01288). The features used to train the model were the interplanetary magnetic field components B-x, B-y, and B-z; the solar wind velocity, nu(sw); the auroral indices, A(u) and A(l); and the geomagnetic index, SYM-H. The SuperDARN velocities were separated into north-south, and east-west components and sorted into a magnetic local time - magnetic latitude grid that ran from 55 degrees to the magnetic pole with a bin size of 2 degrees in latitude, and 1-hr in MLT. Separate models were created for each velocity component in each bin of the grid. It is found that even though the models in each bin are independent of one another a coherent convection pattern is formed when the models are viewed in aggregate. The resulting convection pattern responds to changes in the auroral indices by expanding and contracting in a way that is consistent with expectations for a substorm cycle. Further it is found that the mean-squared difference between predictions of the model and observed values of the velocity are substantially lower than the same quantity calculated for an existing climatology that was not formed with ML techniques. Plain Language Summary This paper presents a new model of northern hemisphere high-latitude convection derived using machine learning (ML). ML was applied to a database of velocities derived from the Super Dual Auroral Radar Network (SuperDARN) observations. The features used to train the model were the interplanetary magnetic field components B-x, B-y, and B-z; the solar wind velocity, nu(sw); the auroral indices, A(u) and A(l); and the geomagnetic index, SYM-H. The SuperDARN velocities were separated into north-south, and east-west components and sorted into a magnetic local time - magnetic latitude grid that ran from 55 degrees to the magnetic pole with a bin size of 2 degrees in latitude, and 1-hr in MLT. Separate models were created for each velocity component in each bin of the grid. The convection patterns generated by the model respond to changes in the auroral indices by expanding and contracting in a way that is consistent with expectations for a substorm cycle. Further it is found that the mean-squared difference between predictions of the model and observed values of the velocity are substantially lower than the same quantity calculated for an existing climatology that was not formed with ML techniques.
更多查看译文
关键词
convection,machine learning,observations
AI 理解论文
溯源树
样例
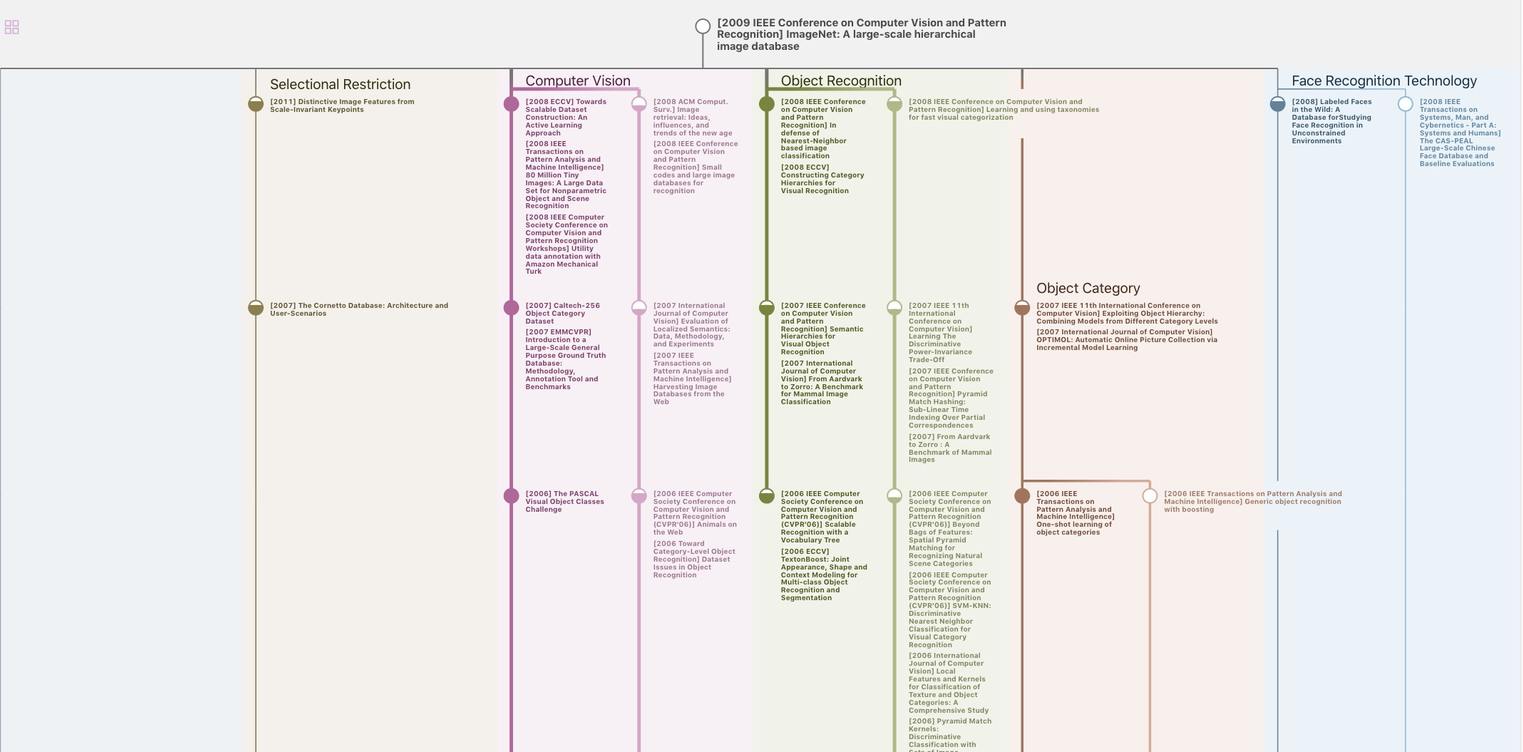
生成溯源树,研究论文发展脉络
Chat Paper
正在生成论文摘要