RIMS: Residual-Inception Multiscale Image Super-Resolution Network
INTERNATIONAL JOURNAL OF COMPUTER SCIENCE AND NETWORK SECURITY(2022)
摘要
The growth of deep learning-based convolutional neural networks (CNNs) for image super-resolution (SR) tasks has improved every day and achieved tremendous performance in recent years. Many deep CNNs based image SR are restricted in practical applications due to their high computational cost, more memory consumption, and more training time. In this paper, we propose a residual-inception multiscale image super-resolution network known as RIMS. Proposed network architecture stacked a 3 CNN layers, 2 skip connection ResNet (SCRB) block and 2 multiscale inception blocks (MSIB) are followed by Leaky ReLU (LReLU) activation function. In addition, shrinking and expanding layers are also used to further reduce the number of parameters while preventing the over-fitting problem during the training. Furthermore, we used a deconvolution layer instead of interpolation to extract the rich features information for reconstruing the high-resolution (HR) output image. The experimental evaluations in terms of both quantitative, as well as qualitative, suggest that the proposed method achieves comparable performance to the existing state-of-the-art methods.
更多查看译文
关键词
Supper-resolution, Convolutional neural networks, Leaky ReLU, PSNR
AI 理解论文
溯源树
样例
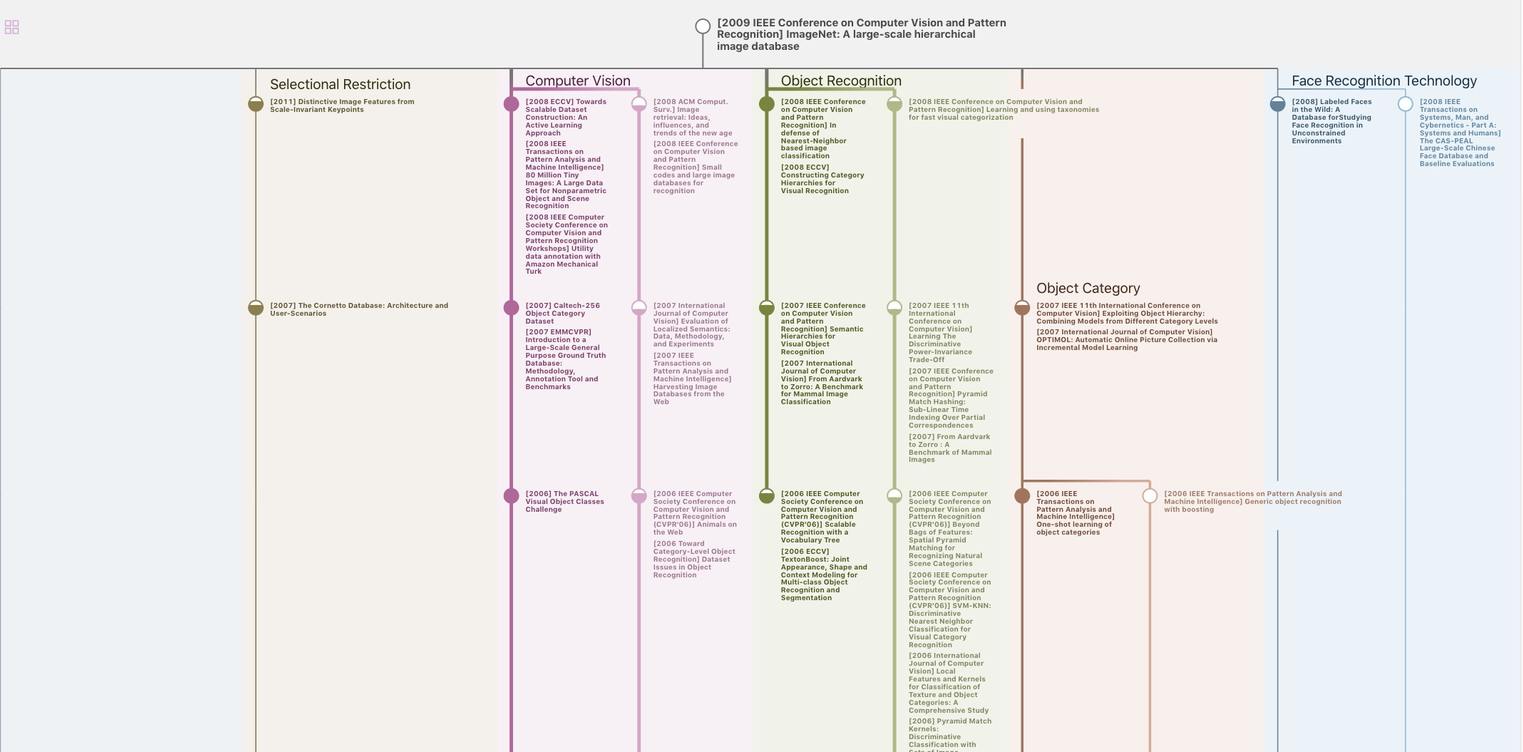
生成溯源树,研究论文发展脉络
Chat Paper
正在生成论文摘要