Assessing Lobe-wise Burden of COVID-19 Infection in Computed Tomography of Lungs Using Knowledge Fusion from Multiple Datasets.
2021 43rd Annual International Conference of the IEEE Engineering in Medicine & Biology Society (EMBC)(2021)
摘要
Segmentation of COVID-19 infection in the lung tissue and its quantification in individual lobes is pivotal to understanding the disease's effect. It helps to determine the disease progression and gauge the extent of medical support required. Automation of this process is challenging due to the lack of a standardized dataset with voxel-wise annotations of the lung field, lobes, and infections like ground-glass opacity (GGO) and consolidation. However, multiple datasets have been found to contain one or more classes of the required annotations. Typical deep learning-based solutions overcome such challenges by training neural networks under adversarial and multi-task constraints. We propose to train a convolutional neural network to solve the challenge while it learns from multiple data sources, each of which is annotated for only a few classes. We have experimentally verified our approach by training the model on three publicly available datasets and evaluating its ability to segment the lung field, lobes and COVID-19 infected regions. Additionally, eight scans that previously had annotations for infection and lung have been annotated for lobes. Our model quantifies infection per lobe in these scans with an average error of 4.5%.
更多查看译文
关键词
COVID-19,Humans,Lung,SARS-CoV-2,Tomography
AI 理解论文
溯源树
样例
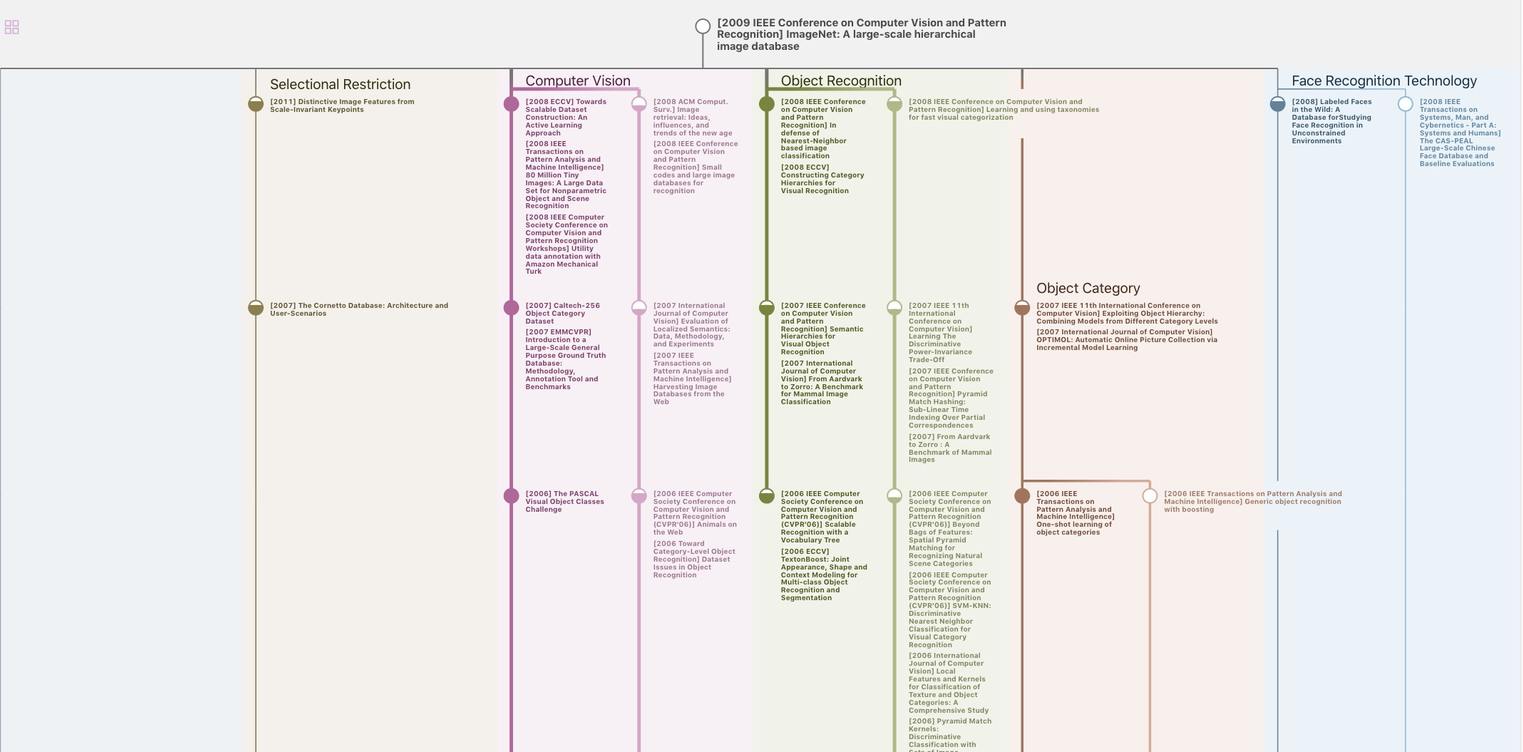
生成溯源树,研究论文发展脉络
Chat Paper
正在生成论文摘要