Data-Driven Control for Radiative Collapse Avoidance in Large Helical Device
PLASMA AND FUSION RESEARCH(2022)
摘要
A radiative collapse predictor has been developed using a machine-learning model with high-density plasma experiments in the Large Helical Device (LHD). The model is based on the collapse likelihood, which is quantified by the parameters selected by the sparse modeling, including (n) over bar (e), CIV, OV, and T-e,(edge). The control system implementing this model has been constructed with a single-board computer to apply this predictor model to the LHD experiment. The controller calculates the collapse likelihood and regulates gas-puff fueling and boosts electron cyclotron resonance heating in real-time. In density ramp-up experiments with hydrogen plasma, high-density plasma has been maintained by the control system while avoiding radiative collapse. This result has shown that the predictor based on the collapse likelihood has the capability to predict a radiative collapse in real-time. (C) 2022 The Japan Society of Plasma Science and Nuclear Fusion Research
更多查看译文
关键词
Large Helical Device (LHD), radiative collapse, density limit, stellarator-heliotron plasmas, sparse modeling, data-driven science, plasma control, collapse avoidance
AI 理解论文
溯源树
样例
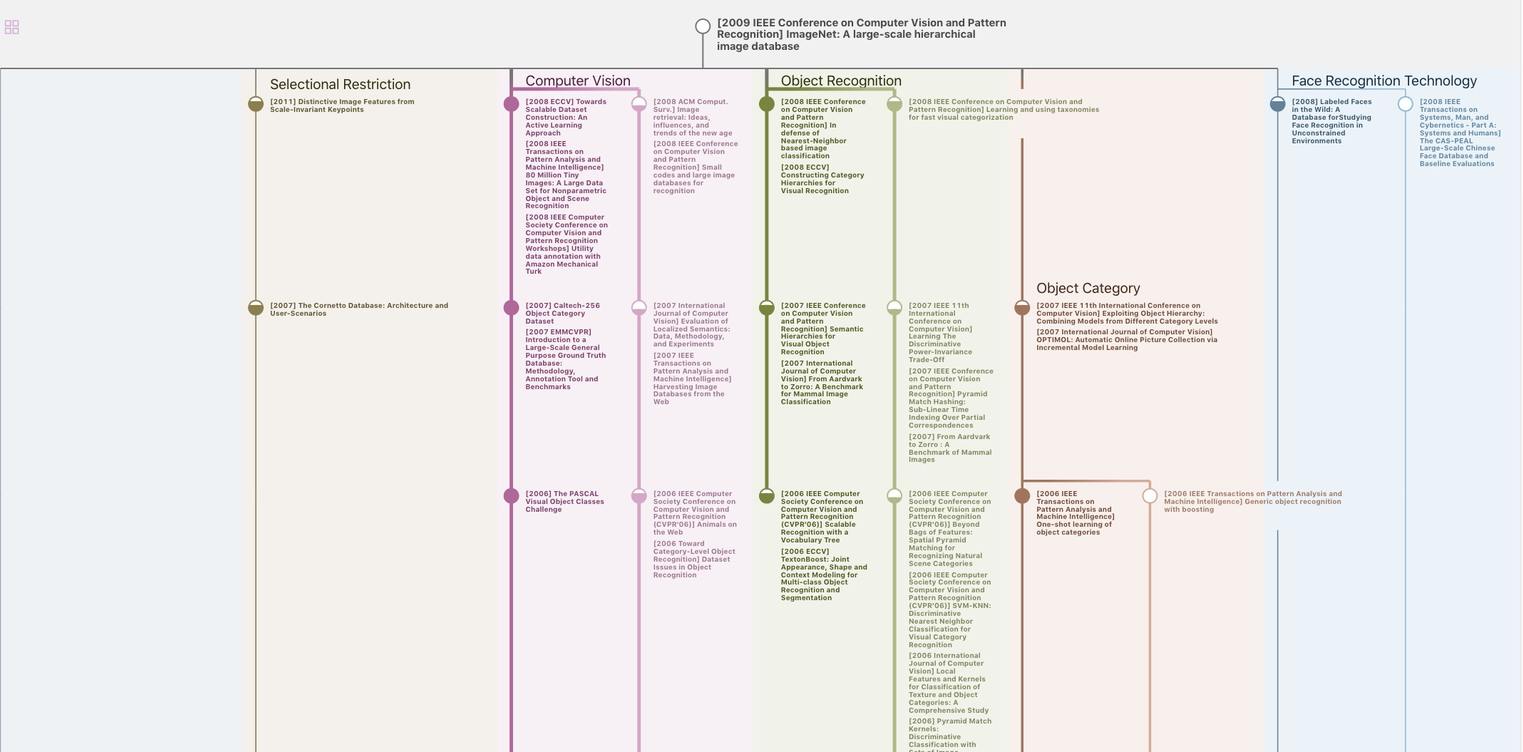
生成溯源树,研究论文发展脉络
Chat Paper
正在生成论文摘要