A Reliable GSHH-DL Routing Protocol for Monitoring the Thermohaline Environment Condition
Wireless Personal Communications(2022)
摘要
In underwater acoustic sensor networks (UASN), the main challenging issues are bandwidth, higher propagation delay, and heavy packet loss during data transmission. The existing UASN routing algorithms have larger latency in the network link and a high rate of packet loss because of the salinity and temperature in the water at different depths. In this nominal, an innovative method called Gravitational Search Hybrid Hexagon-Deep Learning algorithm is proposed. By combining Deep Learning and Gravitation Search, the optimized weighting factor is determined after that which is given to ANN to classify the relay nodes. The weighting value of the classifier is calculated by the GS algorithm to get an accurate reliable relay node status. The classified data has the details about the relay node status in the network which attains whether the relay node is in good status or worst status. These relay node statuses has given to the Hybrid Hexagon scheme to identify the best relay path, by comparing the primary relay node value with one standard value. If the present value is greater than the primary value, then it chooses that value among all neighbour relay node data. Hence the way, the relay node routing is accomplished, if there is any worst stage relay present in the relay path, it can suddenly change the relay path without wasting the time to lose the packet. The routing protocol has been implemented in the ns2-AqaSim simulator and testbed for measurement of the performance metrics of the UASN. The simulation result showed that there is a 45% improvement in throughput, 37% in delay, 47.45% in network lifetime and 48% in packet delivery ratio using the proposed method. It is concluded that the proposed method effectively routing the data packet among the Underwater Acoustic network (UWAN) and successfully improve the network capability with worthy overhead.
更多查看译文
关键词
Routing,Gravitation search (GS),Deep learning (DL),Artificial neural network (ANN)
AI 理解论文
溯源树
样例
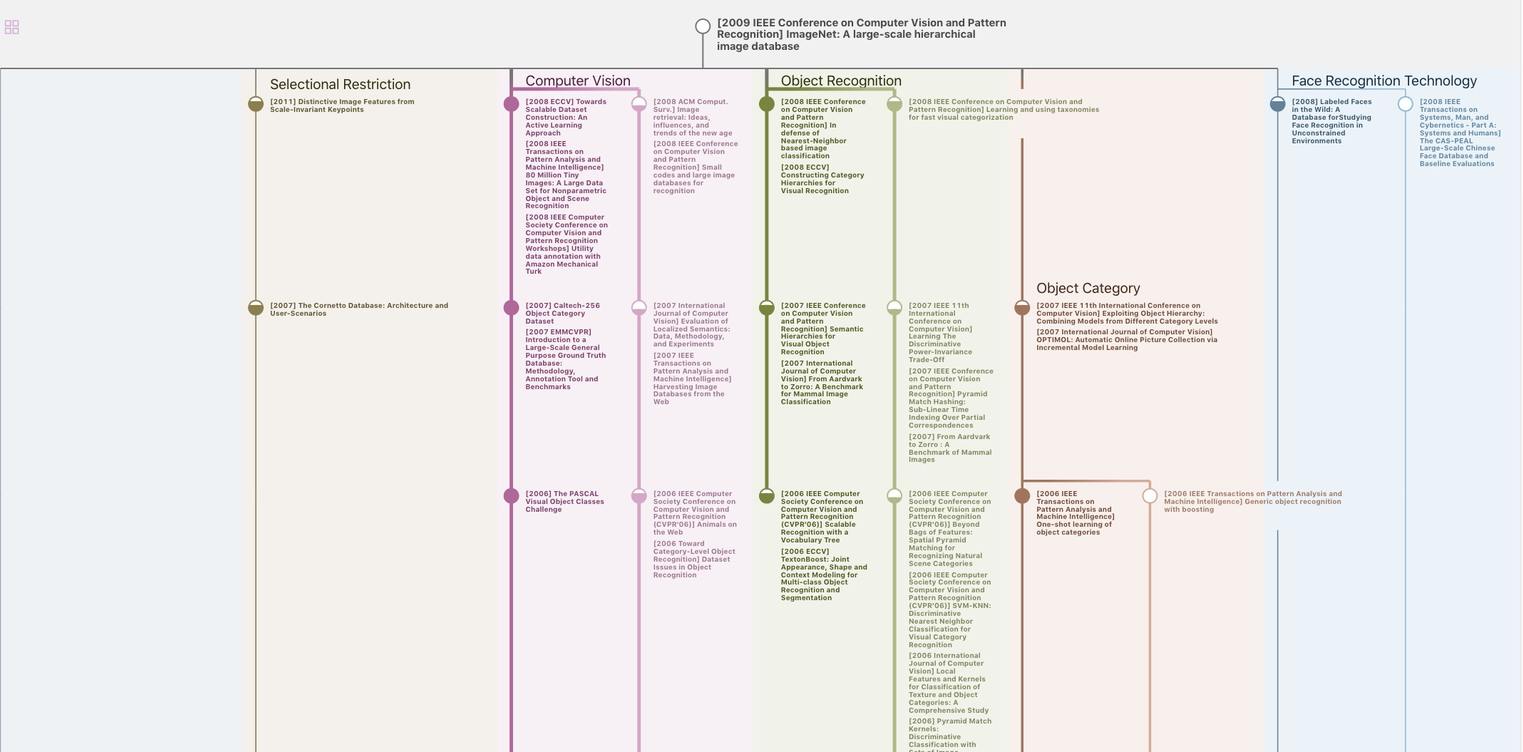
生成溯源树,研究论文发展脉络
Chat Paper
正在生成论文摘要