Active monitoring of powder bed fusion process by training an artificial neural net classifier on layer-by-layer surface laser profilometry data
The International Journal of Advanced Manufacturing Technology(2022)
摘要
This paper reports some recent results related to active monitoring of powder bed fusion (PBF-LB) processes through analysis of layer-by-layer surface profile data. Estimation of fault probability was carried out experimentally in a Renishaw AM250 machine, by collecting Fe3Si powder bed height data, in situ, during the metal additive manufacturing of a heat exchanger section, comprised a series of conformal channels. Specifically, high-resolution powder bed surface height data from a laser profilometer was linked to post-print ground-truth labels (faulty or nominal) for each site from computed tomography (CT) scans, by training a shallow artificial neural net (ANN). The ANN demonstrated interesting capabilities for discovering correlations between surface roughness characteristics and the presence and size of faults. Strong performance was achieved with respect to several standard metrics for classifying faulty and nominal sites. These developments can potentially enable active monitoring processes to become a future component of a layer-by-layer feedback system for better control of PBF-LB processes.
更多查看译文
关键词
Powder bed fusion (PBF-LB),In situ inspection,Laser profilometry,Machine learning
AI 理解论文
溯源树
样例
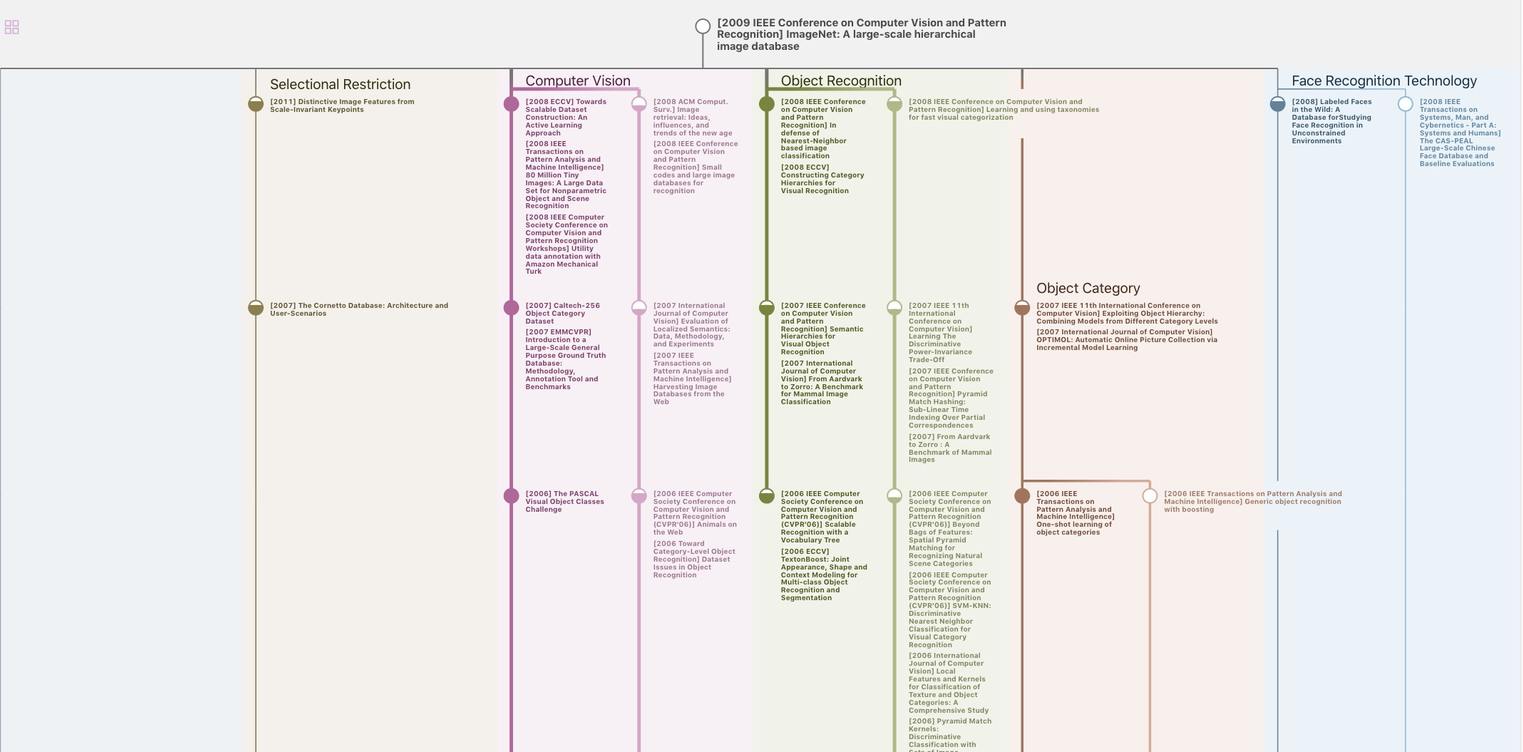
生成溯源树,研究论文发展脉络
Chat Paper
正在生成论文摘要