Parkinson's Detection Using RNN-Graph-LSTM with Optimization Based on Speech Signals
CMC-COMPUTERS MATERIALS & CONTINUA(2022)
摘要
Early detection of Parkinson's Disease (PD) using the PD patients' voice changes would avoid the intervention before the identification of physical symptoms. Various machine learning algorithms were developed to detect PD detection. Nevertheless, these ML methods are lack in generalization and reduced classification performance due to subject overlap. To overcome these issues, this proposed work apply graph long short term memory (GLSTM) model to classify the dynamic features of the PD patient speech signal. The proposed classification model has been further improved by implementing the recurrent neural network (RNN) in batch normalization layer of GLSTM and optimized with adaptive moment estimation (ADAM) on network hidden layer. To consider the importance of feature engineering, this proposed system use Linear Discriminant analysis (LDA) for dimensionality reduction and Sparse Auto-Encoder (SAE) for extracting the dynamic speech features. Based on the computation of energy content transited from unvoiced to voice (onset) and voice to voiceless (offset), dynamic features are measured. The PD datasets is evaluated under 10 fold cross validation without sample overlap. The proposed smart PD detection method called RNN-GLSTM-ADAM is numerically experimented with persistent phonations in terms of accuracy, sensitivity, and specificity and Matthew correlation coefficient. The evaluated result of RNN-GLSTM-ADAM extremely improves the PD detection accuracy than static feature based conventional ML and DL approaches.
更多查看译文
关键词
Dimensionality reduction, LDA, feature extraction, auto encoder, classification, recurrent neural network, LSTM and optimization
AI 理解论文
溯源树
样例
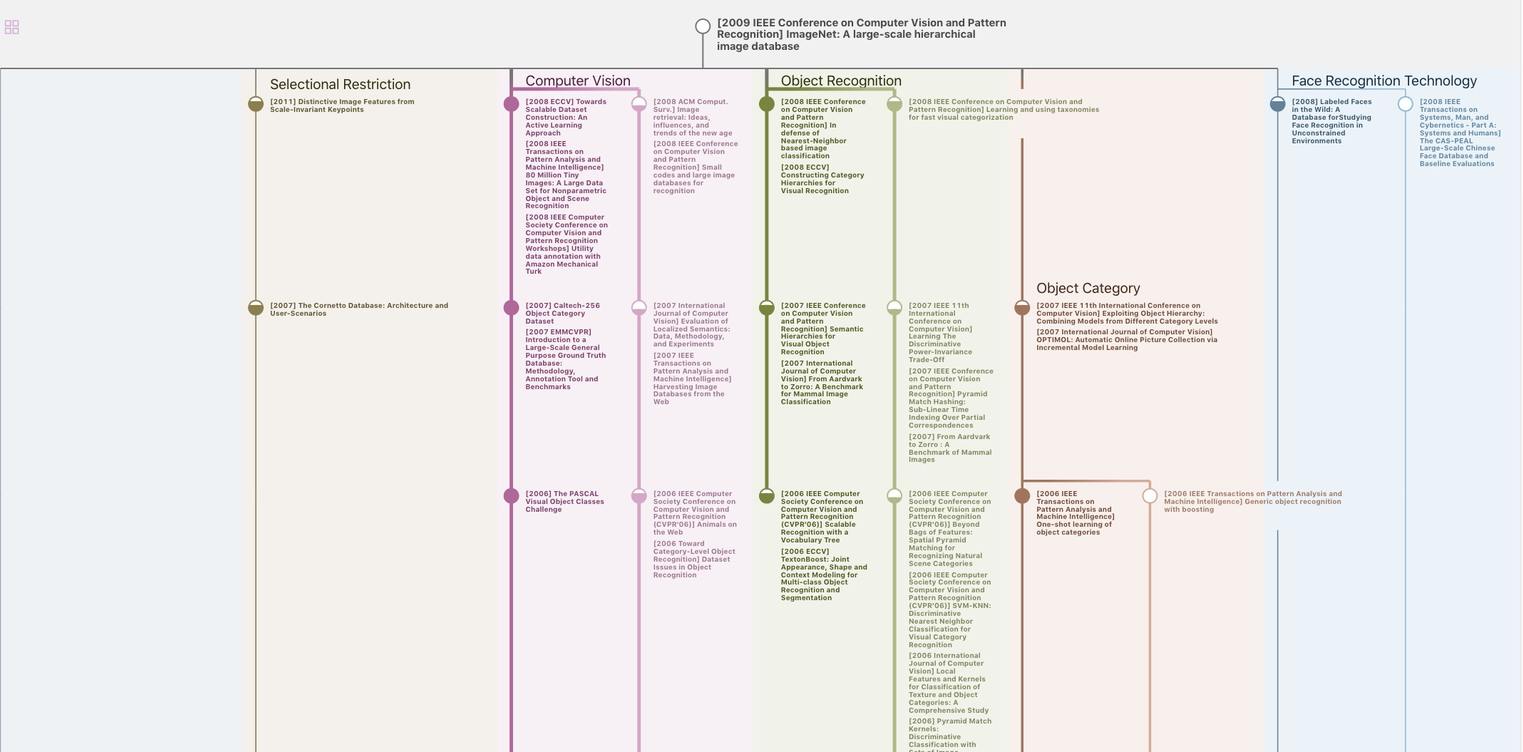
生成溯源树,研究论文发展脉络
Chat Paper
正在生成论文摘要