Solving the shortest path interdiction problem via reinforcement learning
INTERNATIONAL JOURNAL OF PRODUCTION RESEARCH(2023)
摘要
This paper addresses the shortest path interdiction problem, in which the leader aims to maximise the length of the shortest path that the follower can traverse subject to a limited interdiction budget. To solve this problem, we propose a reinforcement learning framework and use the pointer network to handle the situation of variable output sizes. To evaluate the performance of our proposed reinforcement learning model, we conduct extensive computational experiments on a set of instances that are generated from two different network topologies, i.e. the grid networks and the random graphs. To train the pointer network, we consider three different baselines, i.e. the exponential, critical, and rollout baselines, among which the rollout baseline policy achieves the best computational results, and thus is used as the default baseline during our computational experiments. Moreover, when the size of instances increases, we find that solving the equivalent single-level mixed integer program of the problem could be quite time-consuming, while our proposed reinforcement learning approach can still obtain solutions with good performance effectively for both the grid networks and the random graphs.
更多查看译文
关键词
Network interdiction, shortest paths, reinforcement learning, pointer network, rollout baseline
AI 理解论文
溯源树
样例
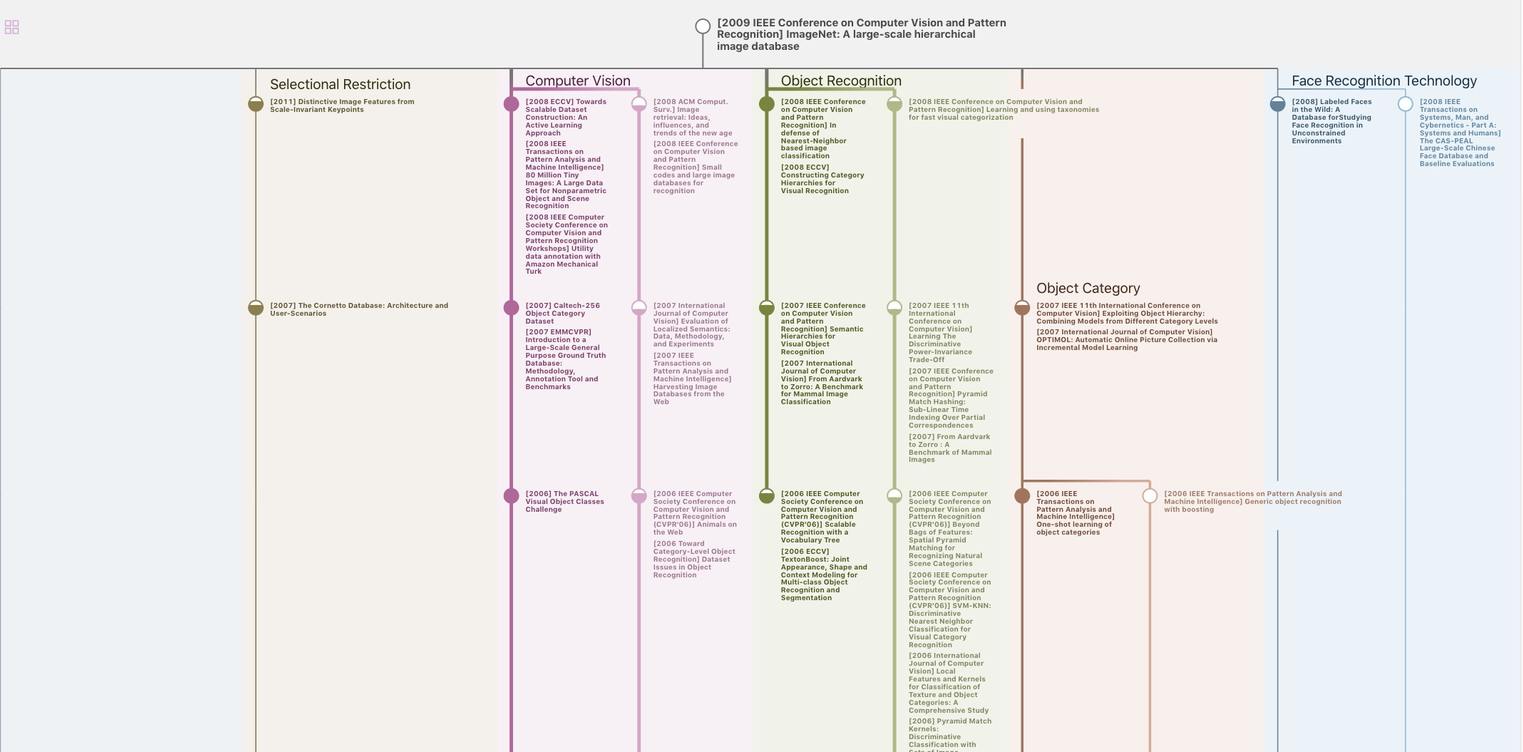
生成溯源树,研究论文发展脉络
Chat Paper
正在生成论文摘要