Using Options to Improve Robustness of Imitation Learning Against Adversarial Attacks
ARTIFICIAL INTELLIGENCE AND MACHINE LEARNING FOR MULTI-DOMAIN OPERATIONS APPLICATIONS III(2021)
摘要
Imitation learning has been shown to be a successful learning technique in scenarios where autonomous agents have to adapt their operation across diverse environments or domains. The main principle underlying imitation learning is to determine a state-to-action mapping, called a policy, from trajectories demonstrated by an expert. We consider the problem of imitation learning under adversarial settings where the expert could be malicious and intermittently give incorrect demonstrations to misguide the learning agent. We propose a technique using temporally extended policies called options to make a learning agent robust against adversarial expert demonstrations. Experimental evaluation of our proposed technique for a game playing AI shows that a learning agent using our options based technique can successfully resist deterioration in its task performance as compared to using conventional reinforcement learning, when an expert adversarially modifies the demonstrations either randomly or strategically.
更多查看译文
AI 理解论文
溯源树
样例
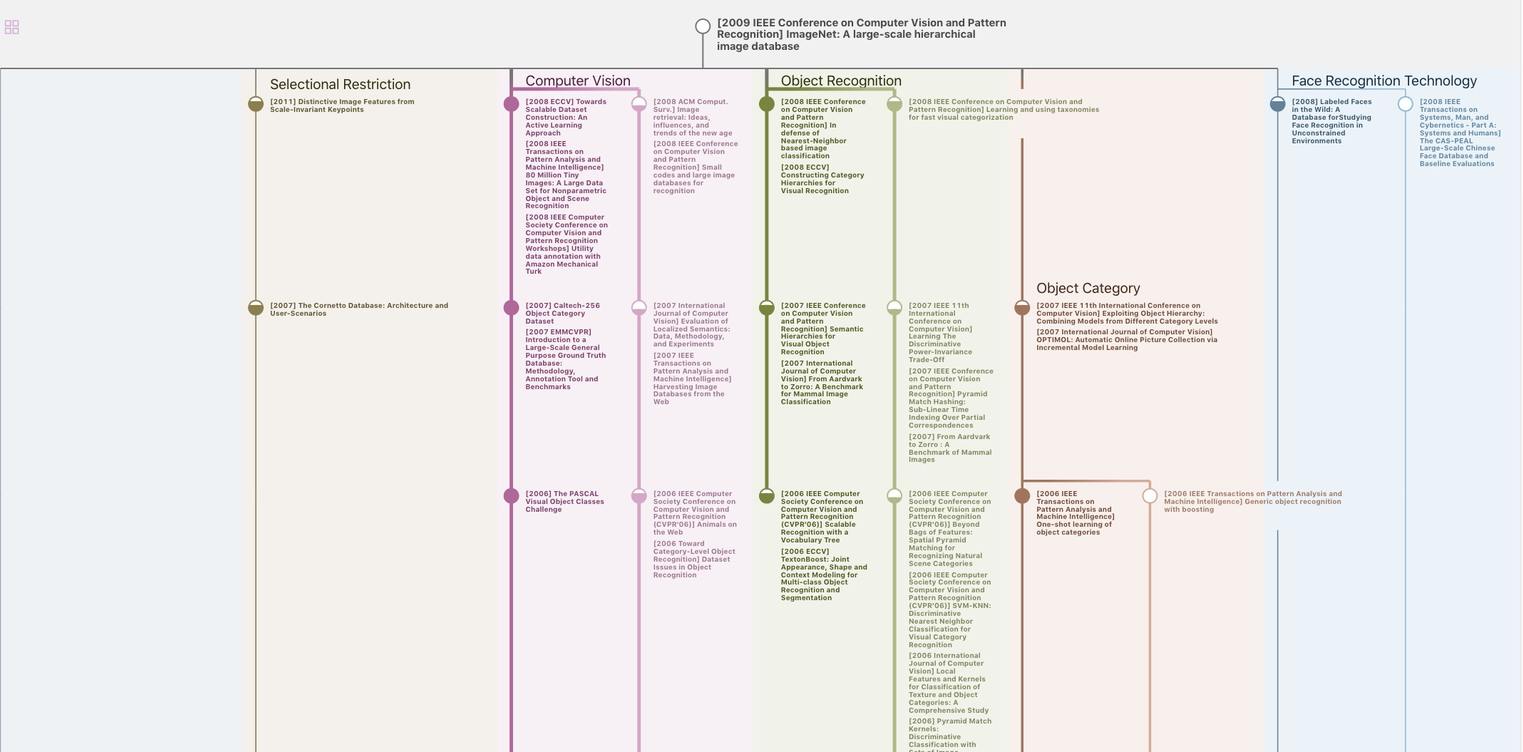
生成溯源树,研究论文发展脉络
Chat Paper
正在生成论文摘要