HRER: A New Bottom-Up Rule Learning for Knowledge Graph Completion
ELECTRONICS(2022)
摘要
Knowledge graphs (KGs) are collections of structured facts, which have recently attracted growing attention. Although there are billions of triples in KGs, they are still incomplete. These incomplete knowledge bases will bring limitations to practical applications. Predicting new facts from the given knowledge graphs is an increasingly important area. We investigate the models based on logic rules in this paper. This paper proposes HRER, a new bottom-up rule learning for knowledge graph completion. First of all, inspired by the observation that the known information of KGs is incomplete and unbalanced, HRER modifies the indicators for screening based on the existing relation rule mining methods. The new metric HRR is more effective than traditional confidences in filtering Horn rules. Besides, motivated by the differences between the embedding-based methods and the methods based on logic rules, HRER proposes entity rules. The entity rules make up for the limited expression of Horn rules to some extent. HRER needs a few parameters to control the number of rules and can provide the explanation for prediction. Experiments show that HRER achieves the state-of-the-art across the standard link prediction datasets.
更多查看译文
关键词
NLP, knowledge graphs, link prediction, reasoning, uneven distribution, reliability, horn rule, entity rule
AI 理解论文
溯源树
样例
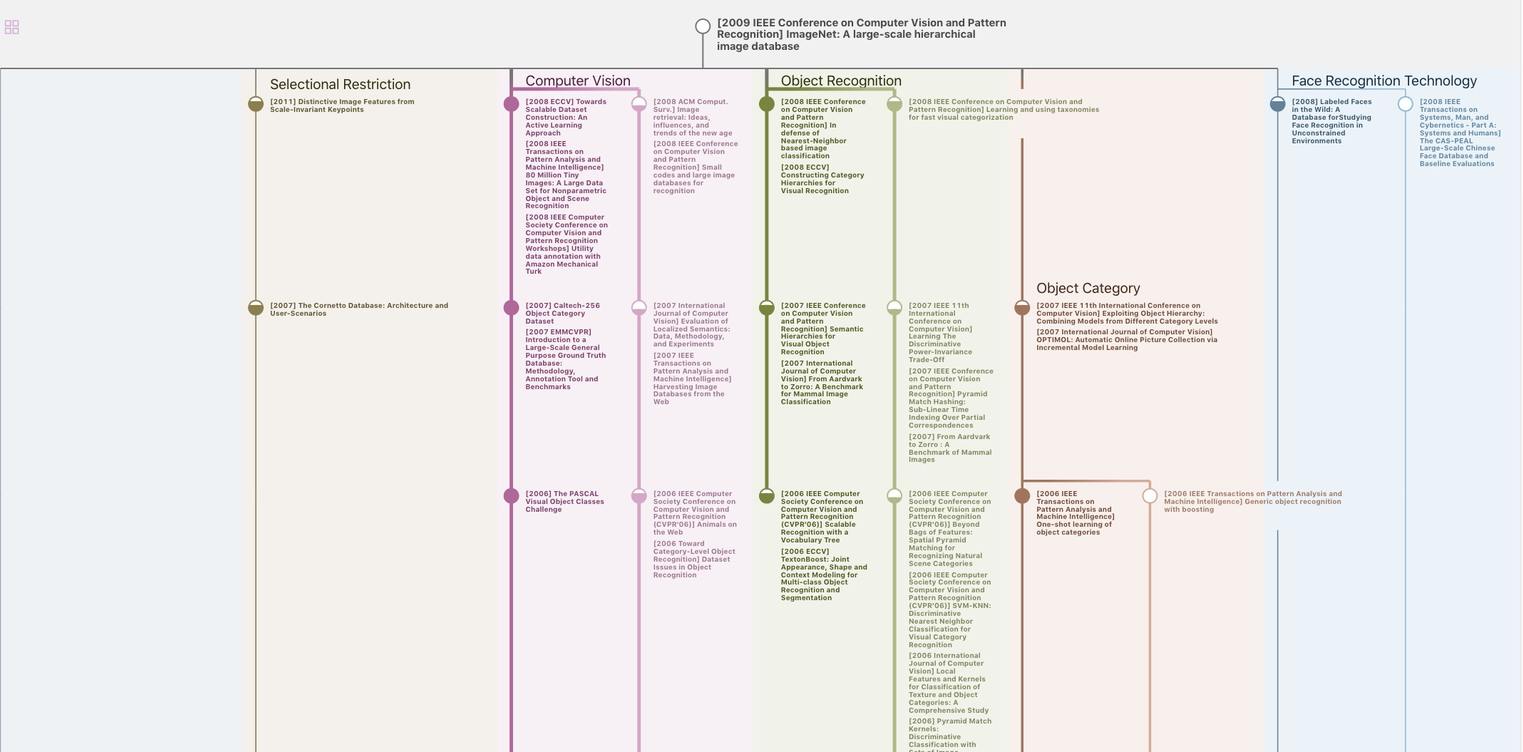
生成溯源树,研究论文发展脉络
Chat Paper
正在生成论文摘要