Landslide susceptibility mapping using artificial neural network tuned by metaheuristic algorithms
ENVIRONMENTAL EARTH SCIENCES(2021)
摘要
As a frequent natural disaster, landslides incur significant economic and human losses worldwide. The main idea of this paper is to propose novel integrative models for landslide susceptibility evaluation in a prone area of Chaharmahal va Bakhtiari Province in Iran. To do this, four metaheuristic techniques, namely chimp optimization algorithm (ChOA), crow search algorithm (CSA), satin bowerbird optimization (SBO), and water cycle algorithm (WCA) are used to supervise the training of an artificial neural network (ANN). A spatial database is created by 170 historical landslides and 14 most common conditioning factors. The models are optimized in terms of hyper-parameters and elite ones are then used to produce the susceptibility maps. During the training phase, all models could acquire a reliable understanding of the landslide pattern. The obtained accuracy index of area under the receiving operating characteristic curve (AUROC) showed that the maps produced by the WCA-ANN (AUROC = 0.925) and SBO-ANN (AUROC = 0.900) are more accurate than those of the ChOA-ANN (AUROC = 0.851) and CSA-ANN (AUROC = 0.855). Considering this assessment, the suggested hybrid models are reliable enough for landslide susceptibility modeling. Accordingly, the generated susceptibility map can be used for urban planning and generating alert systems over the studied area.
更多查看译文
关键词
Natural hazard, Landslide, Susceptibility modeling, Machine learning, Metaheuristic optimization
AI 理解论文
溯源树
样例
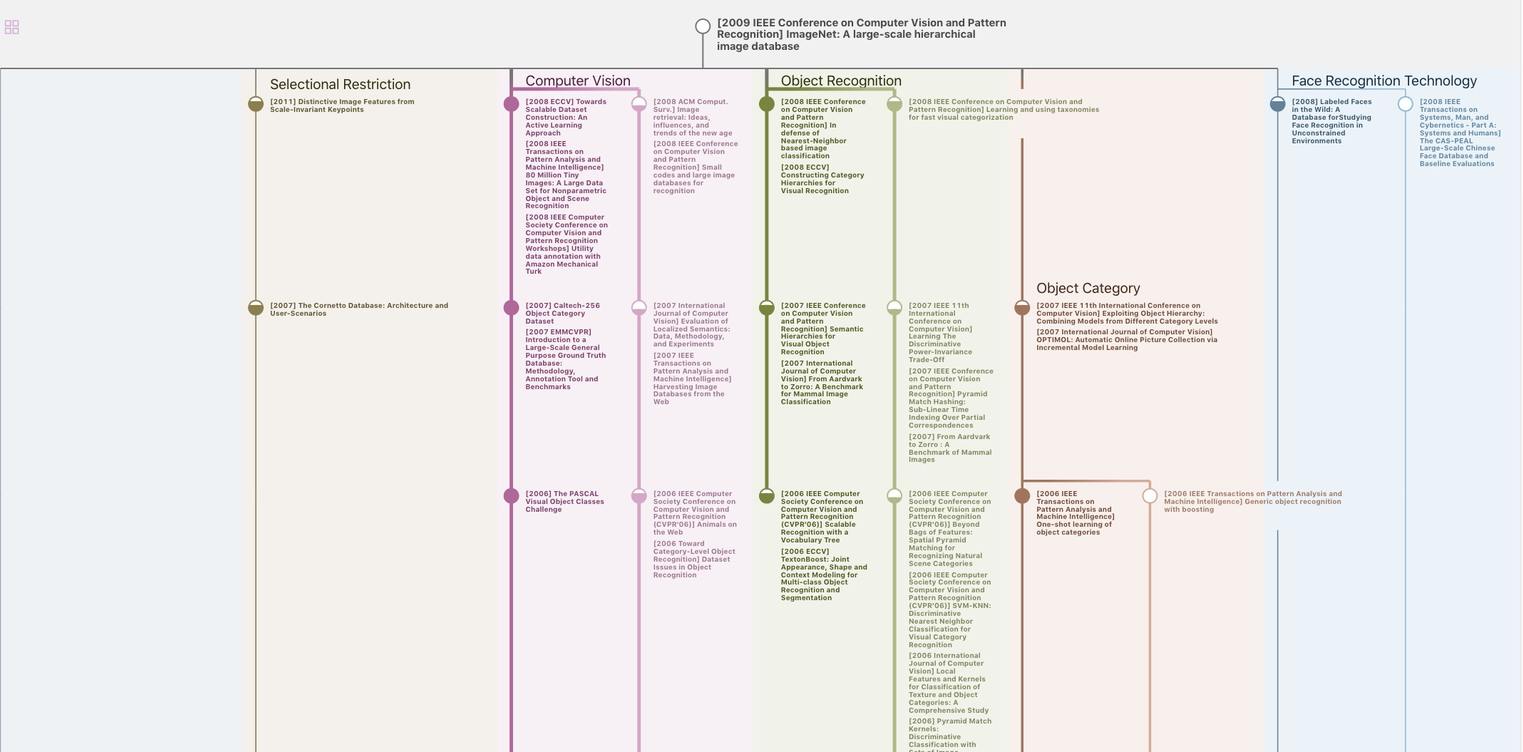
生成溯源树,研究论文发展脉络
Chat Paper
正在生成论文摘要