Parametric and Semi-Parametric Bootstrap-Based Confidence Intervals for Robust Linear Mixed Models (vol 17, pg 271, 2021)
METHODOLOGY-EUROPEAN JOURNAL OF RESEARCH METHODS FOR THE BEHAVIORAL AND SOCIAL SCIENCES(2022)
摘要
The linear mixed model (LMM) is a popular statistical model for the analysis of longitudinal data. However, the robust estimation of and inferential conclusions for the LMM in the presence of outliers (i.e., observations with very low probability of occurrence under Normality) is not part of mainstream longitudinal data analysis. In this work, we compared the coverage rates of confidence intervals (CIs) based on two bootstrap methods, applied to three robust estimation methods. We carried out a simulation experiment to compare CIs under three different conditions: data 1) without contamination, 2) contaminated by within-, or 3) between-participant outliers. Results showed that the semi-parametric bootstrap associated to the composite tau-estimator leads to valid inferential decisions with both uncontaminated and contaminated data. This being the most comprehensive study of CIs applied to robust estimators of the LMM, we provide fully commented R code for all methods applied to a popular example.
更多查看译文
关键词
robustness, linear mixed models, bootstrap, confidence intervals, longitudinal data
AI 理解论文
溯源树
样例
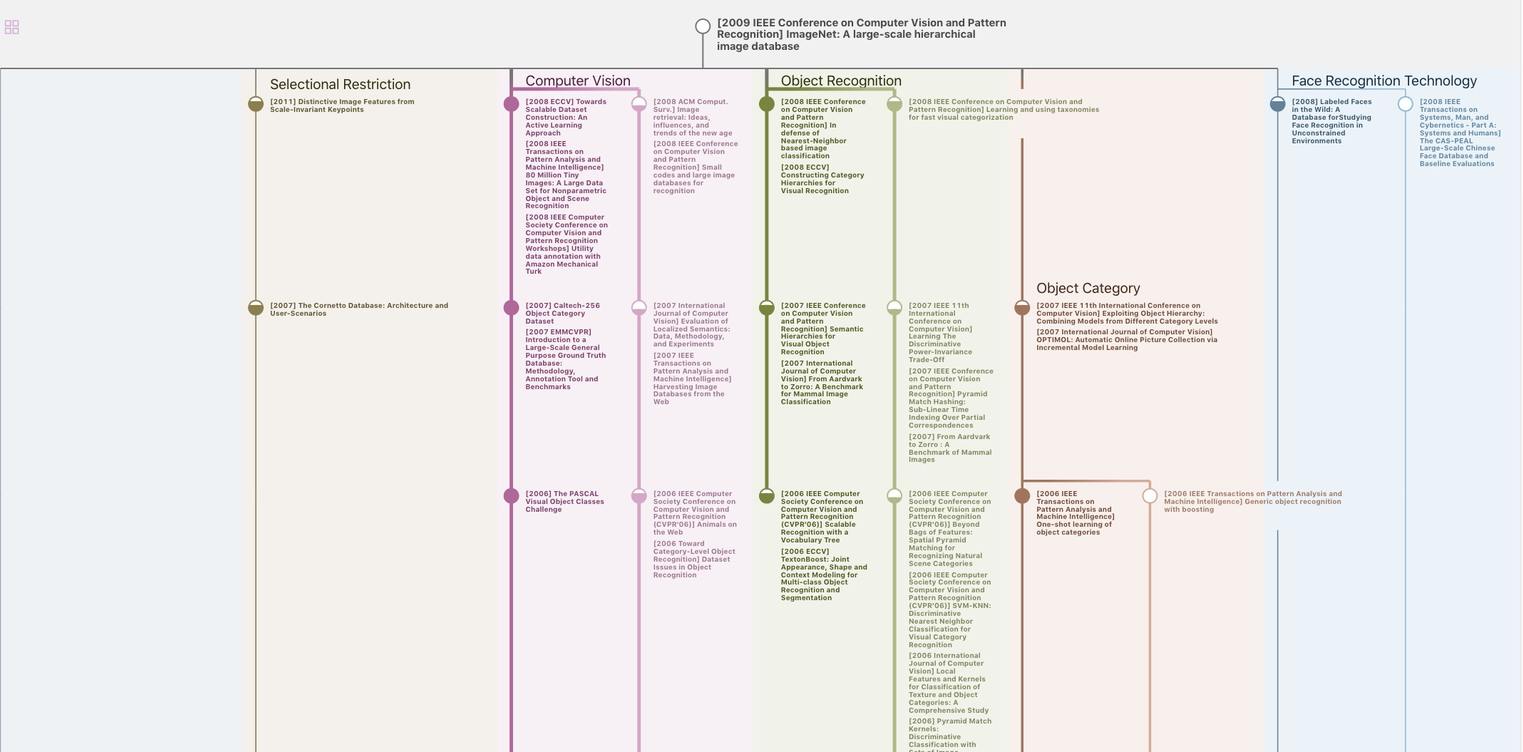
生成溯源树,研究论文发展脉络
Chat Paper
正在生成论文摘要