Predictive Behavioural Monitoring and Deviation Detection in Activities of Daily Living of Older Adults
HEALTHINF: PROCEEDINGS OF THE 15TH INTERNATIONAL JOINT CONFERENCE ON BIOMEDICAL ENGINEERING SYSTEMS AND TECHNOLOGIES - VOL 5: HEALTHINF(2021)
摘要
Predictive behaviour monitoring of Activities of Daily Living (ADLs) can provide unique, personalised insights about an older person's physical and cognitive health and lead to unique opportunities to support self-management, proactive intervention and promote independent living. In this paper, we analyse ADL data from ambient sensors to model behaviour markers on a daily basis. Using a number of machine learning and statistical methods we model a predicted daily routine for each marker, detect deviations based on a set of relative thresholds and calculate long-term drifts. We further analyse the causal factors of deviations by investigating relationships between different activities. We demonstrate our results using data from a sample of 11 participants from the CASAS dataset. Finally, we develop a dashboard to visualize our computed daily routines and quantified deviations in an attempt to offer useful feedback to the monitored person and their caregivers.
更多查看译文
关键词
Activities of Daily Living, Behaviour Monitoring, Deviation Detection, Predictive Modelling, Sensor Data
AI 理解论文
溯源树
样例
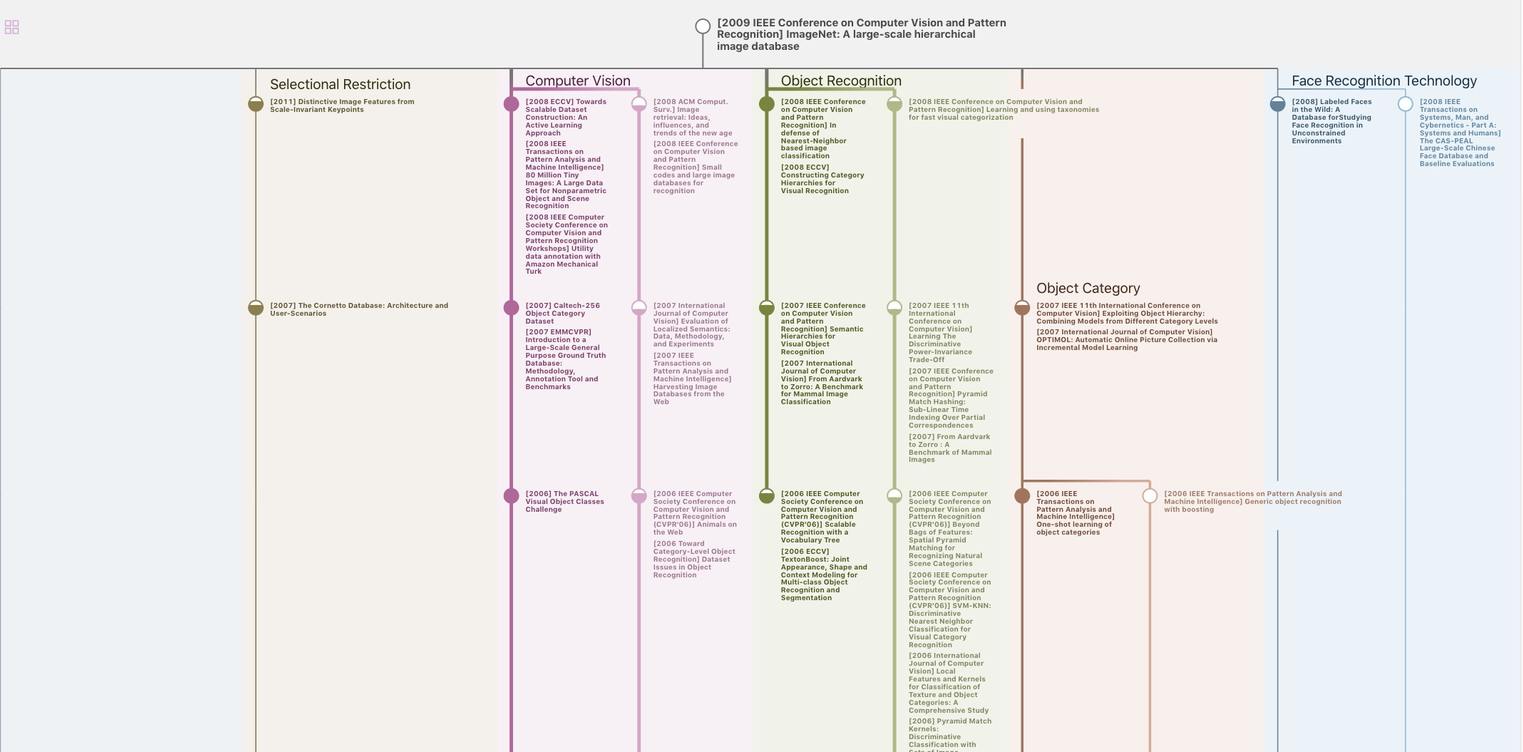
生成溯源树,研究论文发展脉络
Chat Paper
正在生成论文摘要