Simulating Segregation in a Ternary Cu-Pd-Au Alloy with Density Functional Theory, Machine Learning, and Monte Carlo Simulations
JOURNAL OF PHYSICAL CHEMISTRY C(2022)
摘要
Simulation of the segregation profile of multi-component alloys is important to investigate the catalytic properties of alloy catalysts. Density functional theory (DFT) is too expensive to use directly to evaluate the potential energies of the slab configurations during the simulations. In this work, we build a neural network (NN) based on 5278 DFT calculations as a surrogate model to evaluate the potential energies of the fcc(111) slabs for a ternary Cu-Pd-Au alloy. The trained NN is capable of predicting the Cu-Pd-Au potential energies across the whole ternary space with high accuracy. Combining the NN with Monte Carlo simulation, we obtained the segregation profile of Cu-Pd-Au at 600 K across the bulk composition space. The simulation results are qualitatively consistent with the experimental data for PdAu and CuAu, but they are incorrect along the PdCu line. Further DFT calculations show that the perfect fcc(111) slab is not capable of capturing the CuPd segregation behavior on undercoordinated surfaces under the realistic conditions.
更多查看译文
关键词
cu–pd–au alloy,ternary cu–pd–au,density functional theory,monte carlo simulations
AI 理解论文
溯源树
样例
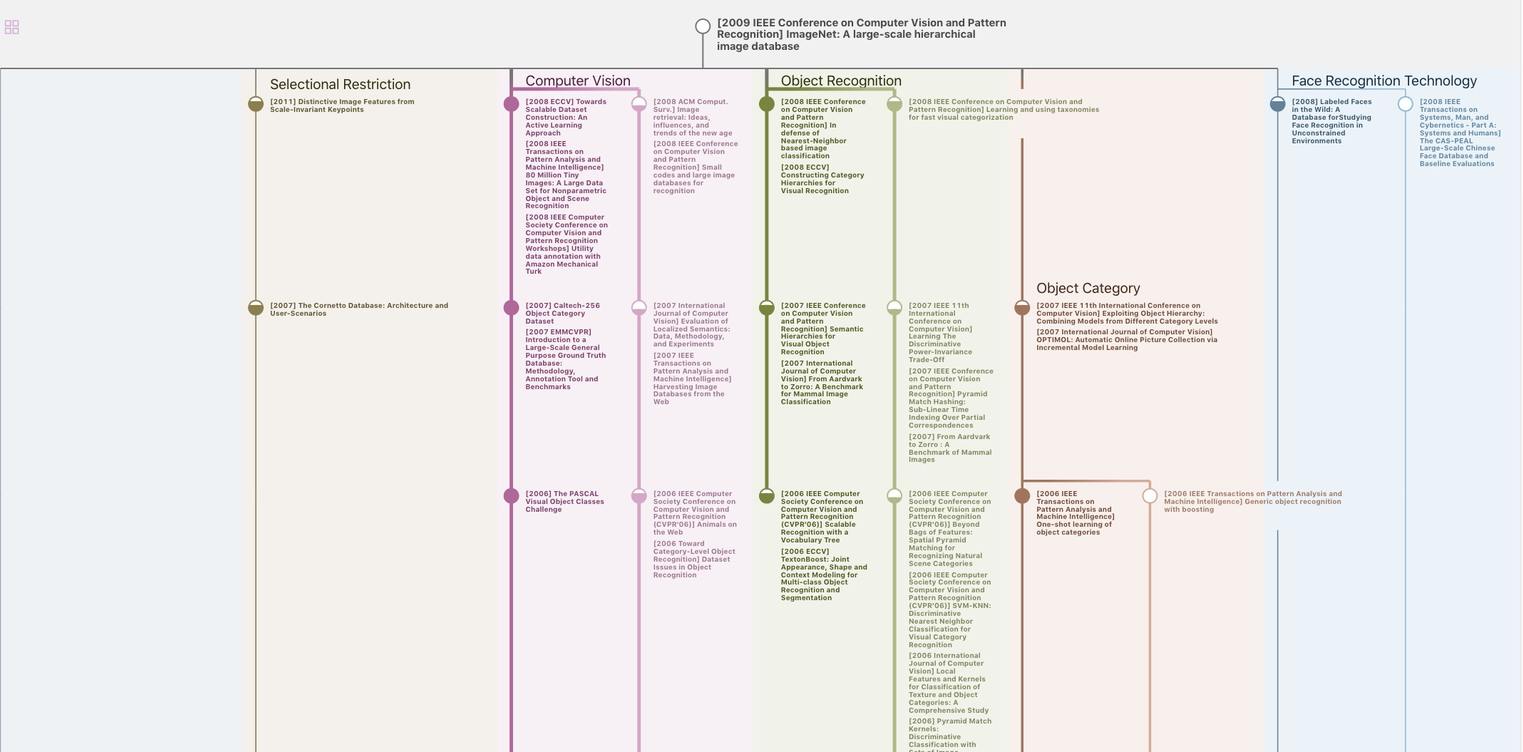
生成溯源树,研究论文发展脉络
Chat Paper
正在生成论文摘要