Improving Learning Efficiency for Wireless Resource Allocation with Symmetric Prior
IEEE Wireless Communications(2022)
摘要
Improving learning efficiency is paramount for learning resource allocation with deep neural networks (DNNs) in wireless communications over highly dynamic environments. Incorporating domain knowledge into learning is a promising approach to dealing with this issue. It is also an emerging topic in the wireless community. In this article, we briefly summarize two approaches for using domain knowledge: introducing a mathematical model and prior knowledge to deep learning. Then, we consider a type of symmetric prior permutation equivariance, which widely exists in wireless tasks. To explain how such a generic prior is harnessed to improve learning efficiency, we resort to ranking, which jointly sorts the input and output of a DNN. We use power allocation among subcarriers, probabilistic content caching, and interference coordination to illustrate the improvement of learning efficiency by exploiting the property. From the case study, we find that the required training samples to achieve given system performance decreases with the number of subcarriers or contents, owing to an interesting phenomenon called “sample hardening.” Simulation results show that the training samples, the free parameters in DNNs, and the training time can be reduced dramatically by harnessing the prior knowledge. The samples required to train a DNN after ranking can be reduced by 15 ∼ 2,400 folds to achieve the same system performance as the counterpart without using prior.
更多查看译文
关键词
wireless resource allocation,learning resource allocation,deep neural networks,wireless communications,highly dynamic environments,deep learning,symmetric prior permutation equivariance,power allocation,DNNs
AI 理解论文
溯源树
样例
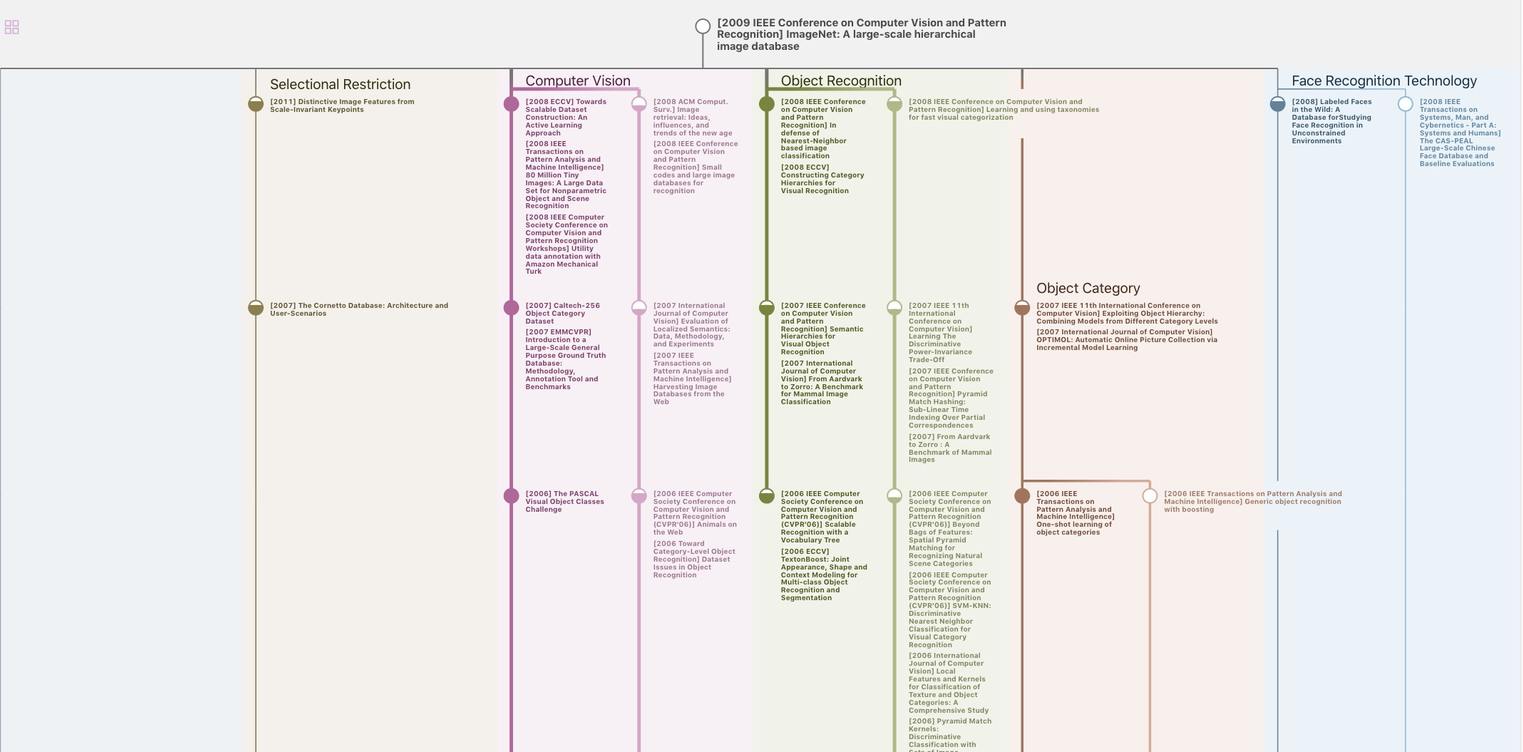
生成溯源树,研究论文发展脉络
Chat Paper
正在生成论文摘要