A Combined, Fully Automated Electrocardiogram and Echocardiogram Strategy for Detection of Hypertrophic Cardiomyopathy
CIRCULATION(2021)
摘要
Introduction: Hypertrophic cardiomyopathy (HCM) is a disorder characterized by abnormal thickening of the heart muscle and may affect up to 1 in 200 people in the general population. Detection of HCM, like other rare diseases, is challenging both because of its low prevalence and the fact that there are other more common diseases such as aortic valve stenosis (AS) and hypertension that also manifest as cardiac hypertrophy. Distinguishing among such conditions, though challenging for human interpretation, may be feasible with artificial intelligence (AI)-based approaches, but strategies to date have looked only at data from single centers using single modalities. Methods: Here we developed and validated a robust approach to accelerate detection of HCM using a combination of fully automated interpretation of electrocardiograms (ECG) and echocardiograms using convolutional neural networks and evaluated it on data from multiple institutions in multiple countries. The ECG model was trained on 4,935 ECGs from Brigham and Women’s Hospital (BWH) and was tested on an internal test set of 1,950 ECGs along with 842 and 1,103 ECGs from 2 different academic medical centers (AMC) in the United States (US). The echocardiogram model was trained on 4,483 studies from BWH and was tested on an internal test set of 1,945 studies, 1,025 and 554 studies from 2 AMCs in the US, and 239 studies from an AMC in Japan. Results: Both models had excellent accuracy for detecting HCM with C-statistics of 0.84-0.91 and 0.91-0.94 for the ECG and echocardiography models, respectively. The echocardiography model, which uses only a single commonly acquired view, discriminates HCM from AS and HTN with C-statistics of 0.92 and 0.86, respectively. Simulating deployment on 13,906 and 7,775 patients with ECG-echocardiography paired data for AMC2, and AMC3 indicated a positive predictive value (PPV) for the ECG model of 7% and 8% at 50% and 59% recall, respectively. Pre-screening with ECG enhanced the echocardiography model performance from PPV 43% and 36% to PPV 71% and 73% at 46% recall, respectively, for AMC2 and AMC3. Conclusions: We have developed a robust pipeline to augment detection of HCM, and that could enable patient identification in less specialized settings.
更多查看译文
AI 理解论文
溯源树
样例
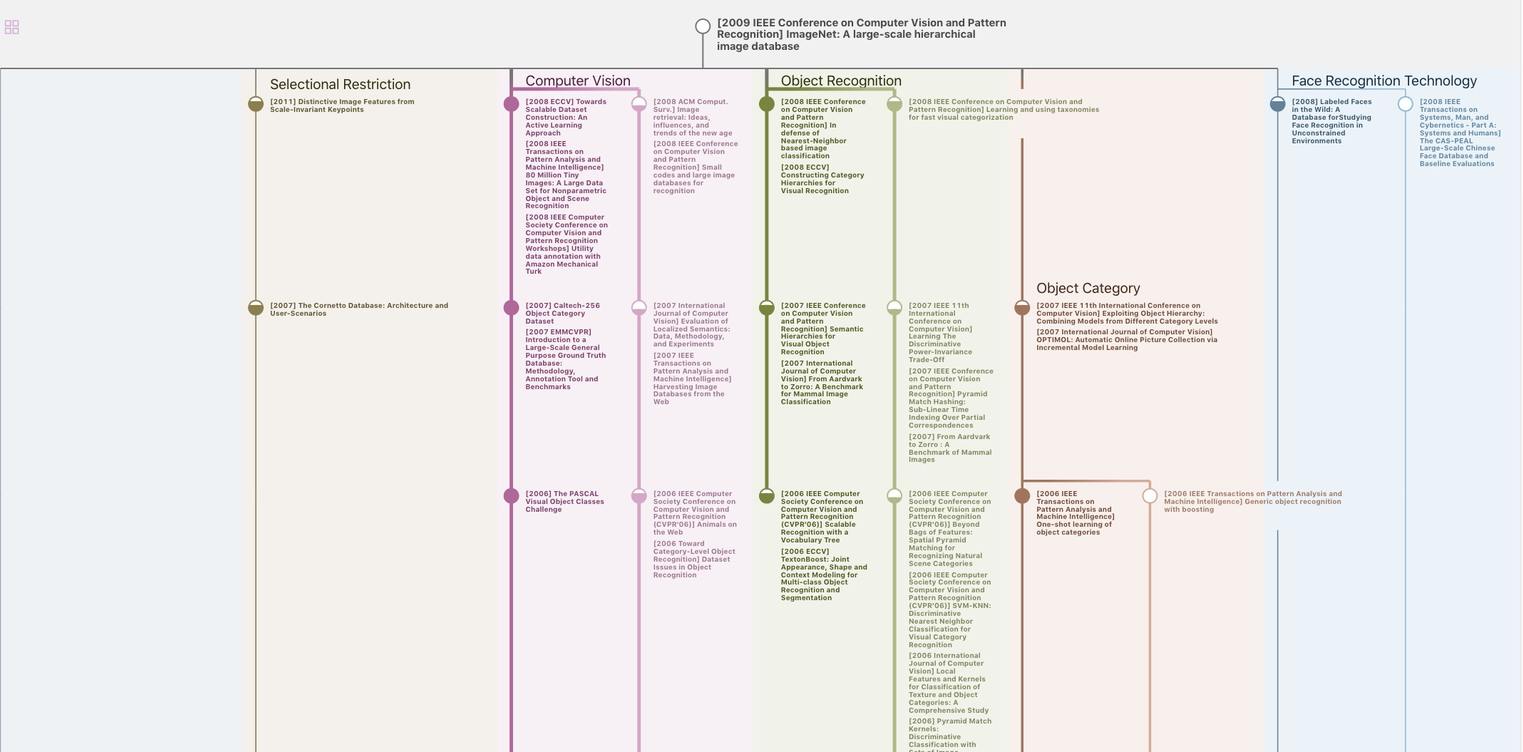
生成溯源树,研究论文发展脉络
Chat Paper
正在生成论文摘要