Baseline Clinical and Genetic Data Based Machine Learning Predictions of 1-Year Ischemic Outcomes Following Percutaneous Coronary Intervention
CIRCULATION(2021)
摘要
Background: Ischemic event rates are low after percutaneous coronary intervention (PCI), conversely second generation P2Y12 inhibitors increase bleeding therefore identifying patients at risk to enable precision in prescribing dual anti-platelet therapy (DAPT) is of clinical interest. Prior machine-learning predictive analyses have been limited by use of older generation stents and lack of genetic data. We utilized clinical and genetic data of patients with new generation stents enrolled in TAILOR-PCI, a 40-center international trial to predict ischemic events after PCI. Methods: There were 4398 eligible post PCI patients with acute coronary syndromes or stable coronary artery disease who were randomly split into training (n=3299) and validation (n=1099) cohorts. The primary endpoint comprising of cardiovascular death, myocardial infarction, stroke, or stent thrombosis within 12 months of PCI was predicted with patient characteristics prior to hospital discharge. First, random forests were used for feature selection with 1,000 bootstraps comprising equal numbers of those with and without ischemic events to minimize the bias of sample imbalance. Features were selected by a consensus on variable-importance over bootstraps. Second, using these features in the training cohort, support vector machines (SVM) with a polynomial kernel was trained with repeated 5-fold cross validation to choose a model with highest area under the receiver operating characteristic curve (AUC) which was then used to predict ischemic events in the validation cohort. Results: The primary endpoint occurred in 2.4% of patients. Using 89 clinical and genetic variables in the random-forest feature selection step, variable importance-based consensus identified 50 top predictors. These 50 predictors achieved AUC=0.703 in the SVM training cohort and AUC=0.744 (specificity=0.84; sensitivity =0.43) in the SVM validation cohort. Conclusions: Machine learning methods using baseline clinical and genetic data enables prediction of infrequent ischemic events in post PCI patients receiving new generation stents. The role of such artificial intelligence algorithms in individualization of DAPT by identifying high or low risk patients needs to be explored.
更多查看译文
AI 理解论文
溯源树
样例
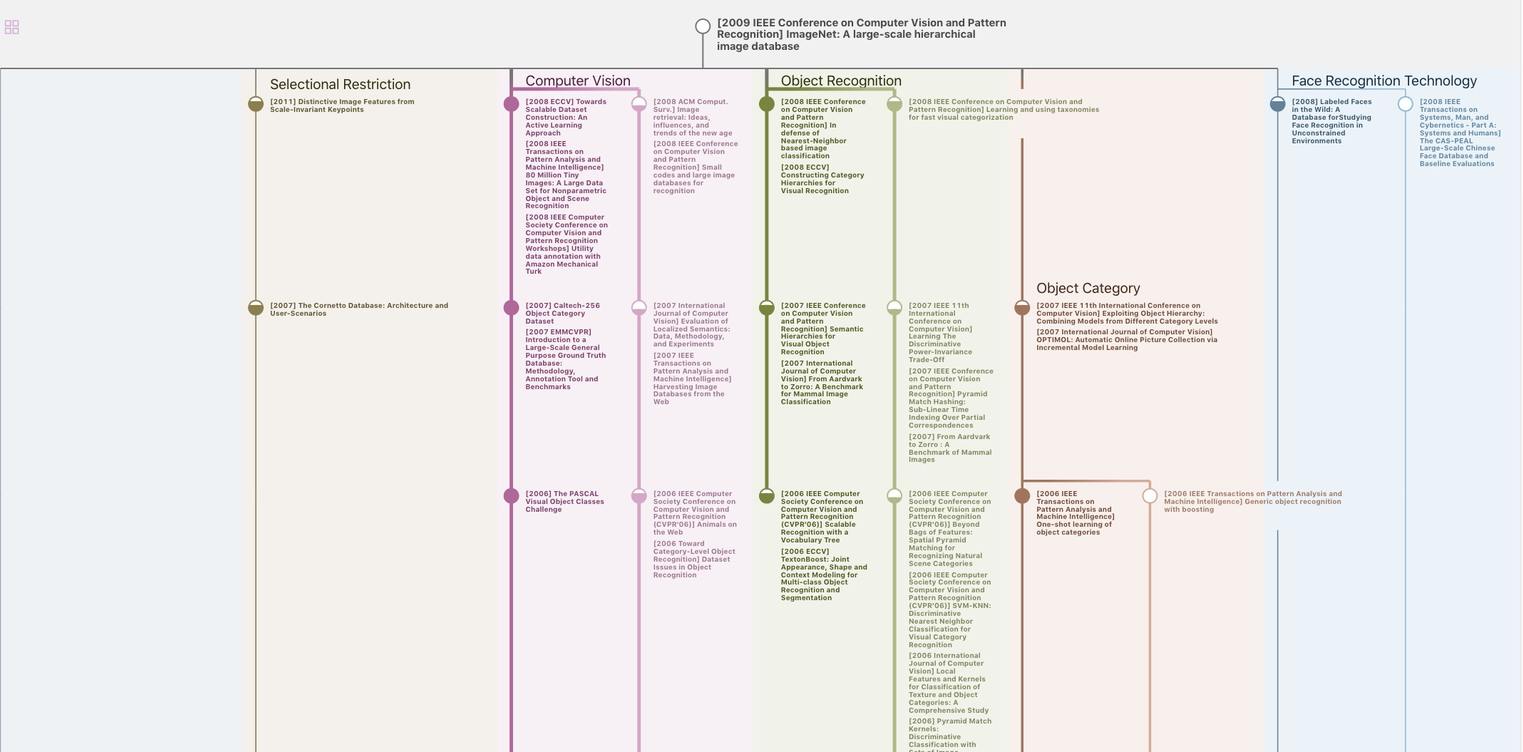
生成溯源树,研究论文发展脉络
Chat Paper
正在生成论文摘要