An Adaptive Modeling Framework for Bearing Failure Prediction
ELECTRONICS(2022)
摘要
Rolling element bearings are a common component in rotating equipment, a class of machines that is essential in a wide range of industries. Detecting and predicting bearing failures is then vital for reducing maintenance and production costs due to unplanned downtime. In previous literature, significant efforts have been devoted to building data-driven health models from historical bearing data. However, a common limitation is that these methods are typically tailored to specific failure instances and have limited ability to model bearing failures between repairs in the same system. In this paper, we propose a multi-state health model to predict bearing failures before they occur. The model employs a regression-based method to detect health state transition points and applies an exponential random coefficient model with a Bayesian updating process to estimate time-to-failure distributions. A model training framework is also introduced to make our proposed model applicable to more bearing instances in the same system setting. The proposed method has been tested on a publicly available bearing prognostics dataset. Case study results show that the proposed method provides accurate failure predictions across several system failures, and that the training approach can significantly reduce the time necessary to generate an effective, generalized model.
更多查看译文
关键词
predictive maintenance, bearings, remaining useful life, prognostic model training
AI 理解论文
溯源树
样例
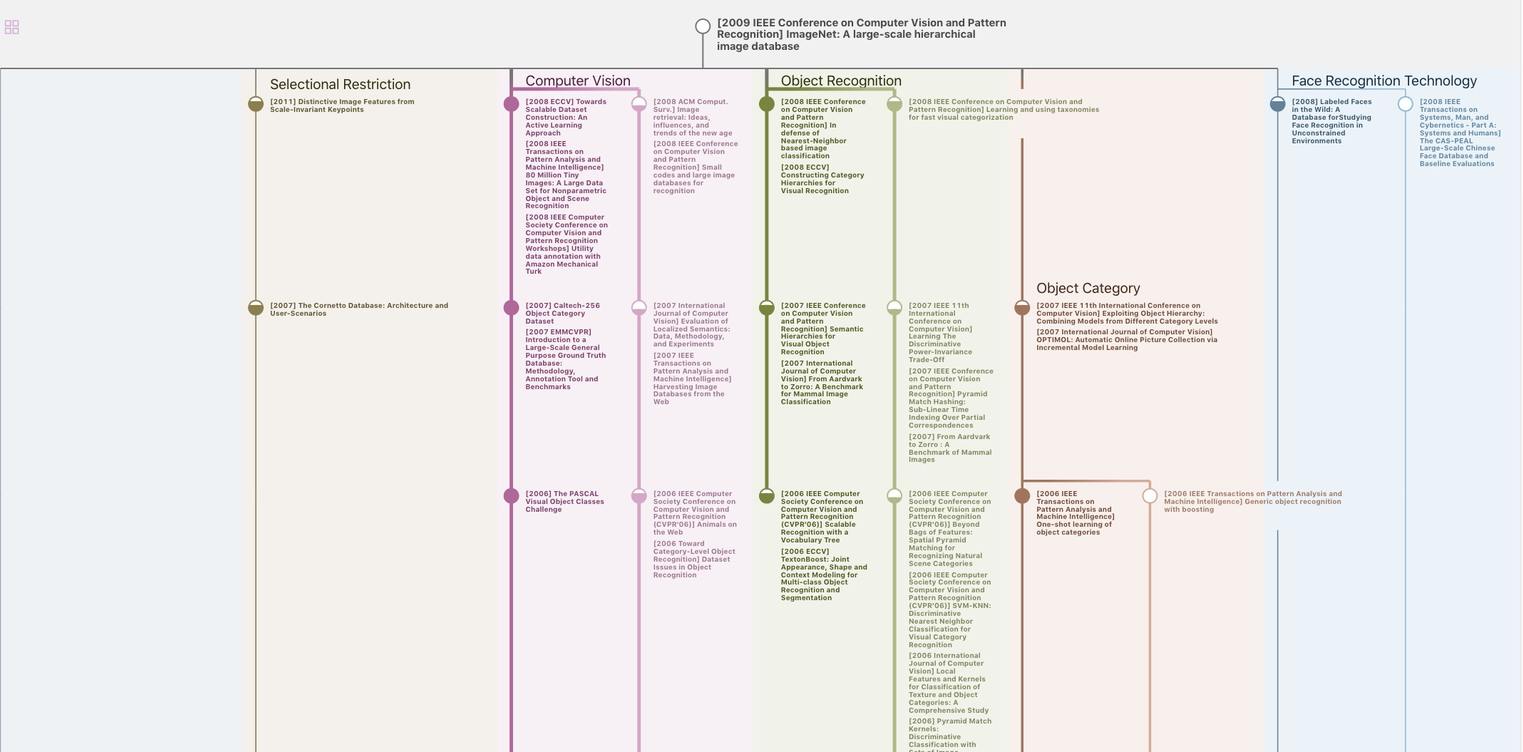
生成溯源树,研究论文发展脉络
Chat Paper
正在生成论文摘要