Interpreting Model Predictions with Constrained Perturbation and Counterfactual Instances
INTERNATIONAL JOURNAL OF PATTERN RECOGNITION AND ARTIFICIAL INTELLIGENCE(2022)
摘要
In recent years, machine learning models have achieved magnificent success in many industrial applications, but most of them are black boxes. It is crucial to understand why such predictions are made in many critical areas such as medicine, financial markets, and auto driving. In this paper, we propose Coco, a novel interpretation method which can interpret any binary classifier by assigning each feature an importance value for a particular prediction. We first adopt MixUp method to generate reasonable perturbations, then apply these perturbations with constraints to obtain counterfactual instances and finally compute a comprehensive metric on these instances to estimate the importance of each feature. To demonstrate the effectiveness of Coco, we conduct extensive experiments on several datasets. The results show our method achieves better performance in identifying the most important features compared with the state-of-the-art interpretation methods, including Shap and Lime.
更多查看译文
关键词
Interpreting model, constrained perturbation, counterfactual instances
AI 理解论文
溯源树
样例
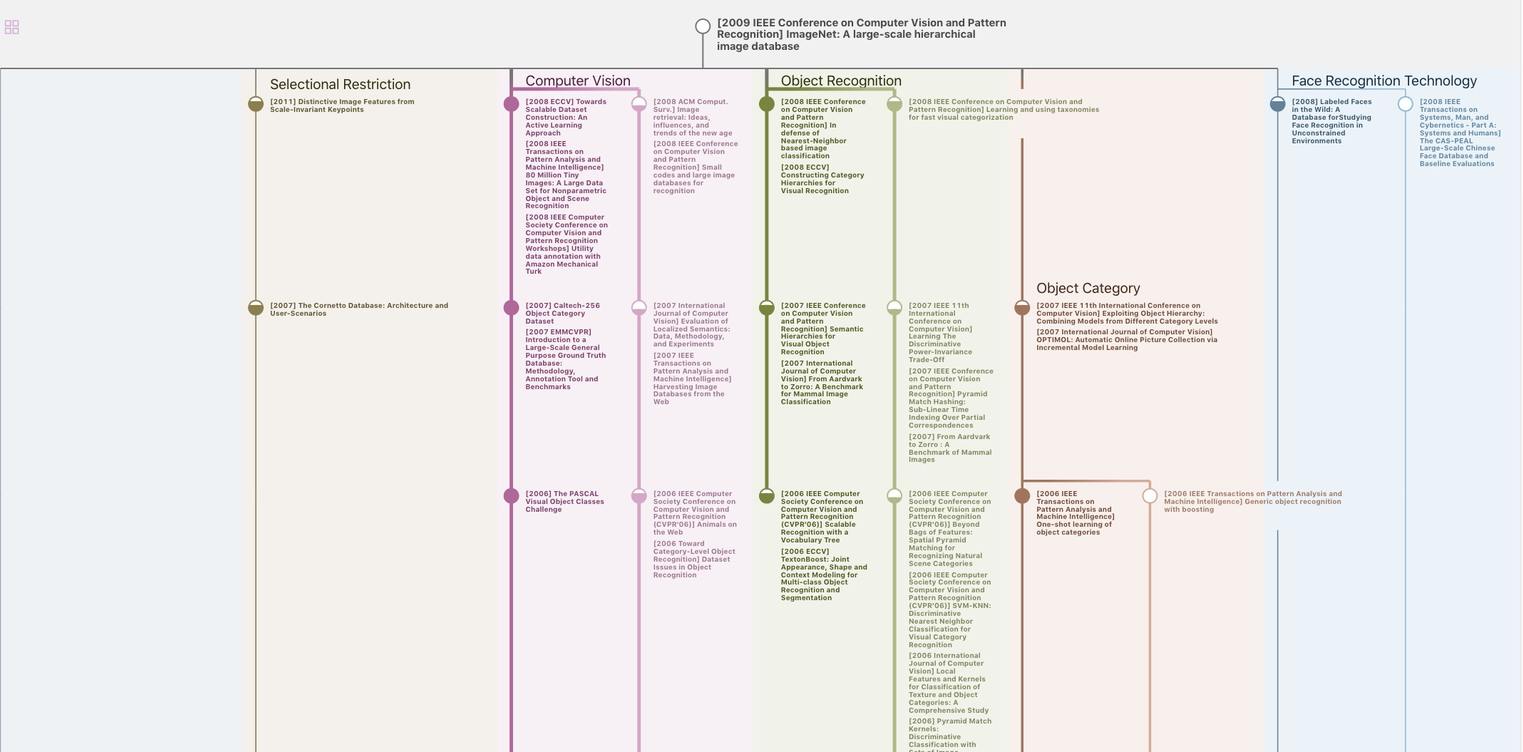
生成溯源树,研究论文发展脉络
Chat Paper
正在生成论文摘要