Unsupervised Machine Learning Identified High-Risk Phenotype in Heart Failure Patients with Syncope
CIRCULATION(2021)
摘要
Introduction: Syncope was reported to be associated with sudden cardiac death in heart failure patients regardless of the etiology of syncope. We attempted to use unsupervised machine learning to identify the prognostically distinct phenotypes in a large population of heart failure patients presenting with syncope. Hypothesis: Unsupervised machine learning can identify prognostically distinct phenotypes in a large population of heart failure patients presenting with syncope. Methods: Patients with a diagnosis of systolic or diastolic heart failure and syncope at Mayo Clinic sites were identified for baseline and follow-up data. Variables with ≥ 20% missing data were excluded; a total of 57 variables were used for k-means clustering analysis. Patients were grouped according to 4 distinct k-means determined clusters for statistical analysis. Results: A total of 3,557 patients with proper follow-up and echocardiography data were included in the final analysis. The median follow-up duration was 36.3 months, and 2908 (81.7%) patients died. Cluster 2 (n= 680) was identified as the cluster with the highest mortality (92%), which contained patients with high proportions of cardiovascular comorbidities, especially chronic kidney disease. The Kaplan-Meier survival curves are shown in Figure 1. In multivariate Cox regression analysis, the presence of CKD independently predicts mortality (HR 1.62, 95%CI 1.50-1.76, p <0.0001) after adjusting for age and sex. Conclusions: Unsupervised machine learning identified patients with CKD, CAD and DM are high-risk phenotypes in heart failure patients with syncope, and can be used to guide future studies in this highly heterogeneous, unexplored population.
更多查看译文
AI 理解论文
溯源树
样例
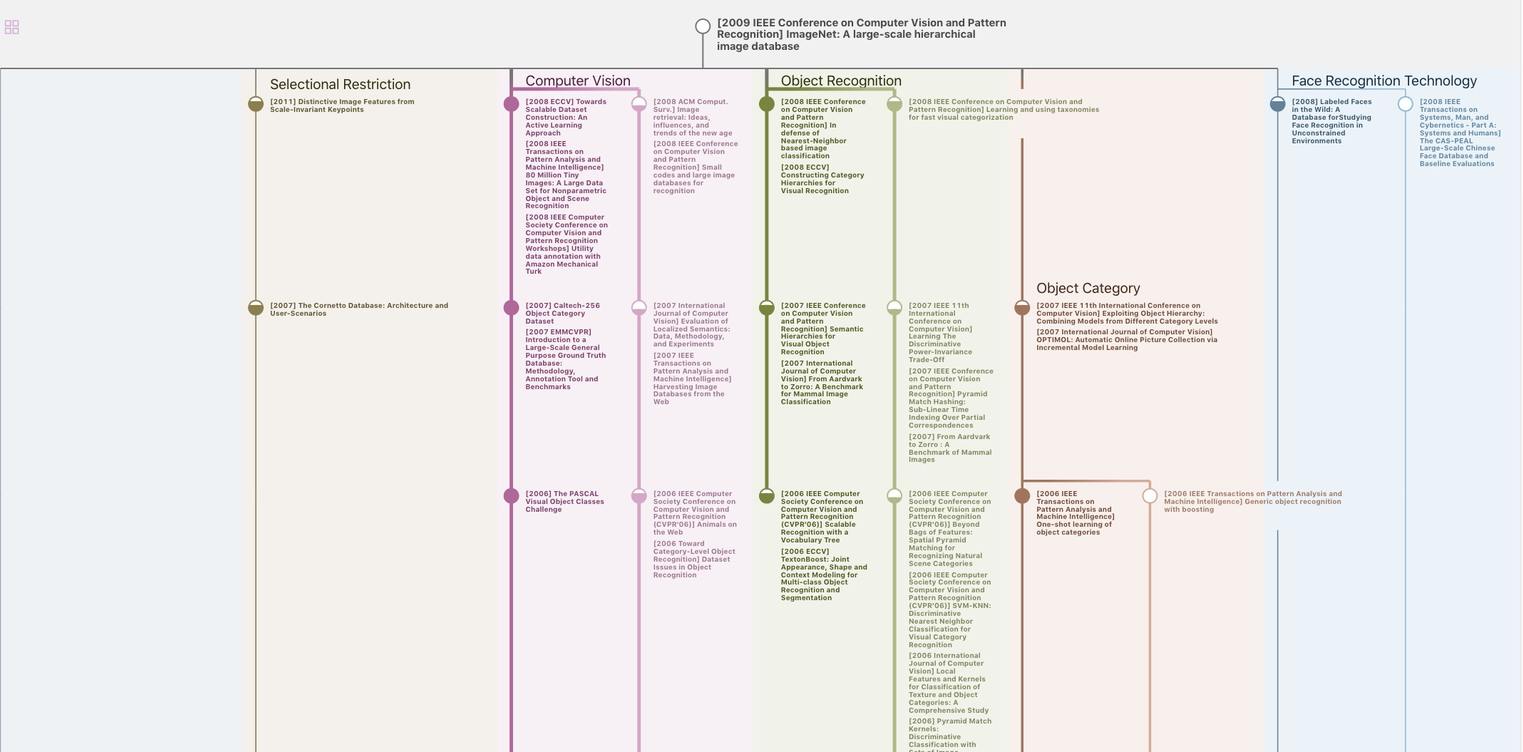
生成溯源树,研究论文发展脉络
Chat Paper
正在生成论文摘要