Machine Learning Algorithm for Estimating Surface PM2.5 in Thailand
AEROSOL AND AIR QUALITY RESEARCH(2021)
摘要
We have used NASA's Modern-Era Retrospective analysis for Research and Applications, Version 2 (MERRA2) reanalysis data of aerosols and meteorology into a machine learning algorithm (MLA) to estimate surface PM2.5 concentration in Thailand. One year of hourly data from 51 ground monitoring stations in Thailand was spatiotemporally collocated with MERRA2 fields. The integrated data then used to train and validate a supervised MLA' random forest' to estimate hourly and daily PM2.5 concentrations. The MLA is cross-validated using a 10-fold random sampling approach. The trained MLA can estimate PM2.5 with close to zero mean bias across the country. The correlation coefficient of 0.95 with slope and intercept values of 0.95 and 0.88 are achieved between observed and estimated PM2.5. The MLA also shows underestimation at hourly scale under very clean conditions (PM2.5 10 mu g m-3) and overestimation during high loading (PM2.5 80 mu g m-3). The hourly data also demonstrate high skill in following the diurnal cycle during different seasons of the year. The daily mean PM2.5 (24-hour) values follow day-to-day variability very well (correlation coefficient of 0.98, RMSE = 3.14 mu g m-3), showing high value during winter months (November- February) and lower during other seasons. The trained MLA has the potential to reprocess the MERRA2 timeseries for the region, and the bias corrected data can be used in other applications such as long-term trend analysis and health exposure studies. The MLA can also be applied to GEOS forecasted fields to generate bias corrected air quality forecasts for the region.
更多查看译文
关键词
Thailand, MERRA2, PM2, 5, Air quality, Machine learning
AI 理解论文
溯源树
样例
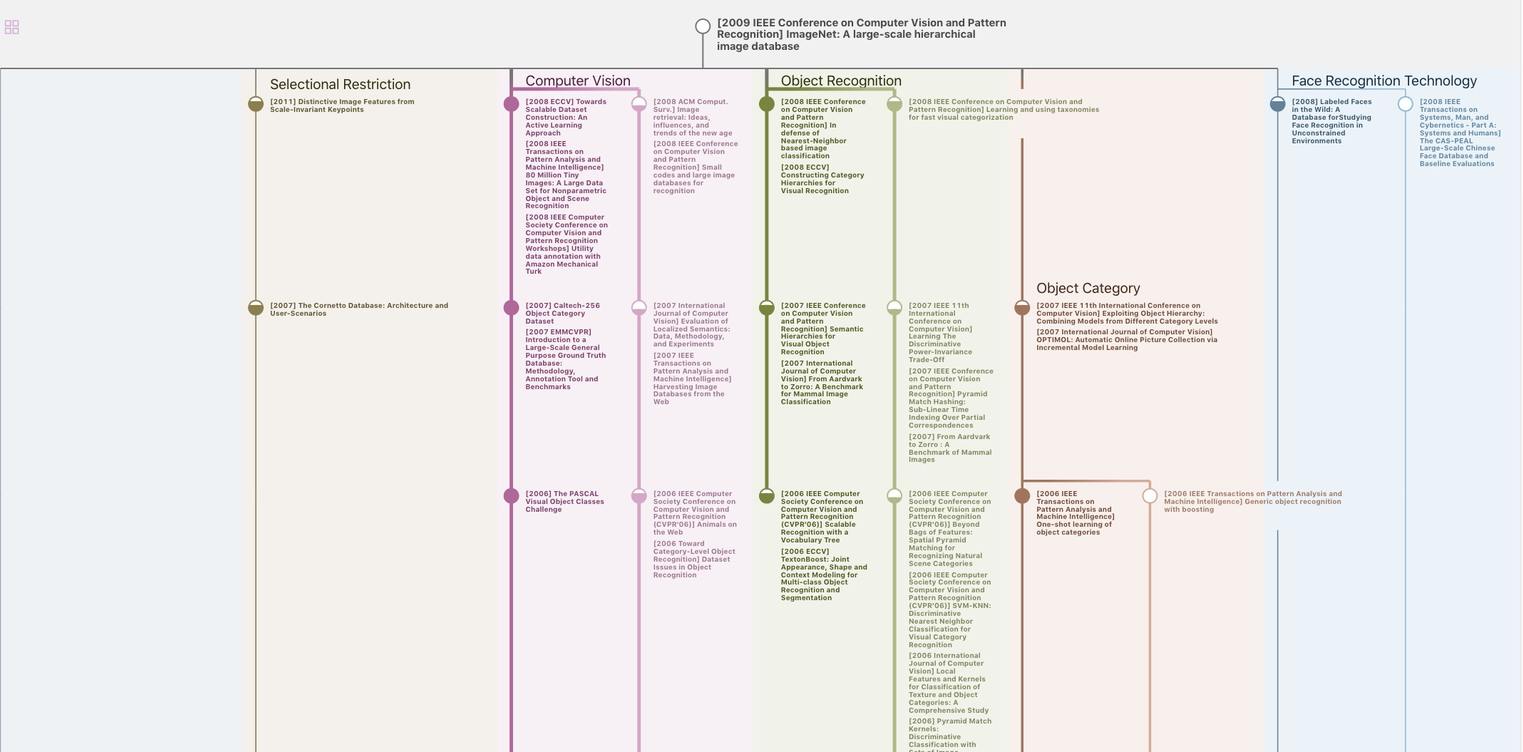
生成溯源树,研究论文发展脉络
Chat Paper
正在生成论文摘要