Developing a Novel Fair-Loan Classifier through a Multi-Sensitive Debiasing Pipeline: DualFair
MACHINE LEARNING AND KNOWLEDGE EXTRACTION(2022)
摘要
Machine learning (ML) models are increasingly being used for high-stake applications that can greatly impact people's lives. Sometimes, these models can be biased toward certain social groups on the basis of race, gender, or ethnicity. Many prior works have attempted to mitigate this "model discrimination" by updating the training data (pre-processing), altering the model learning process (in-processing), or manipulating the model output (post-processing). However, more work can be done in extending this situation to intersectional fairness, where we consider multiple sensitive parameters (e.g., race) and sensitive options (e.g., black or white), thus allowing for greater real-world usability. Prior work in fairness has also suffered from an accuracy-fairness trade-off that prevents both accuracy and fairness from being high. Moreover, the previous literature has not clearly presented holistic fairness metrics that work with intersectional fairness. In this paper, we address all three of these problems by (a) creating a bias mitigation technique called DualFair and (b) developing a new fairness metric (i.e., AWI, a measure of bias of an algorithm based upon inconsistent counterfactual predictions) that can handle intersectional fairness. Lastly, we test our novel mitigation method using a comprehensive U.S. mortgage lending dataset and show that our classifier, or fair loan predictor, obtains relatively high fairness and accuracy metrics.
更多查看译文
关键词
machine learning, algorithmic fairness, bias mitigation, mortgage lending, accuracy-fairness trade-off
AI 理解论文
溯源树
样例
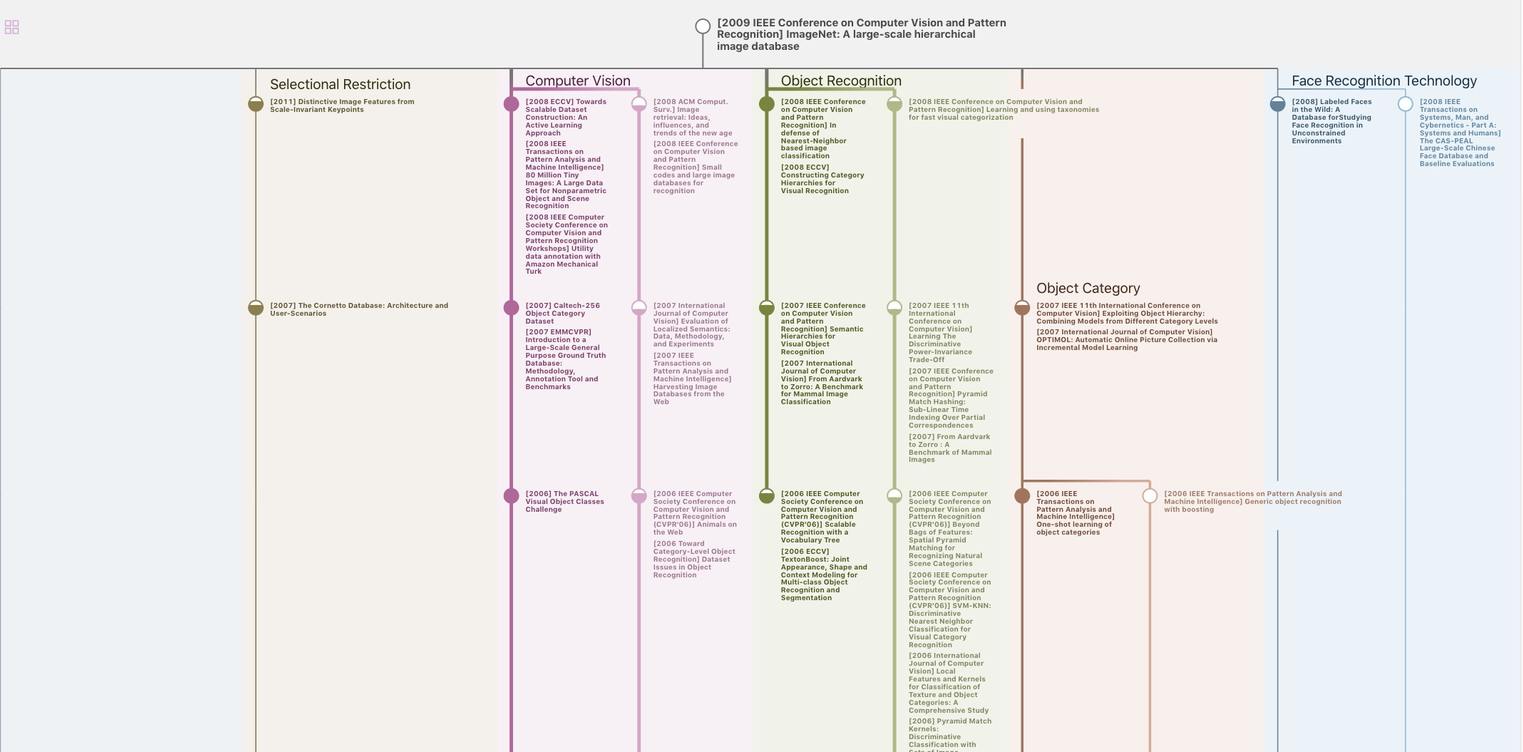
生成溯源树,研究论文发展脉络
Chat Paper
正在生成论文摘要