Imputation of Missing Covariates in Randomized Controlled Trials with Continuous Outcomes: Simple, Unbiased and Efficient Methods
JOURNAL OF BIOPHARMACEUTICAL STATISTICS(2022)
摘要
The literature on dealing with missing covariates in nonrandomized studies advocates the use of sophisticated methods like multiple imputation (MI) and maximum likelihood (ML)-based approaches over simple methods. However, these methods are not necessarily optimal in terms of bias and efficiency of treatment effect estimation in randomized studies, where the covariate of interest (treatment group) is independent of all baseline (pre-randomization) covariates due to randomization. This has been shown in the literature, but only for missingness on a single baseline covariate. Here, we extend the situation to multiple baseline covariates with missingness and evaluate the performance of MI and ML compared with simple alternative methods under various missingness scenarios in RCTs with a quantitative outcome. We first derive asymptotic relative efficiencies of the simple methods under the missing completely at random (MCAR) scenario and then perform a simulation study for non-MCAR scenarios. Finally, a trial on chronic low back pain is used to illustrate the implementation of the methods. The results show that all simple methods give unbiased treatment effect estimation but with increased mean squared residual. It also turns out that mean imputation and the missing-indicator method are most efficient under all covariate missingness scenarios and perform at least as well as MI and LM in each scenario.
更多查看译文
关键词
Randomized trials,missing covariates,linear mixed model,multiple imputation,mean imputation,missing-indicator method
AI 理解论文
溯源树
样例
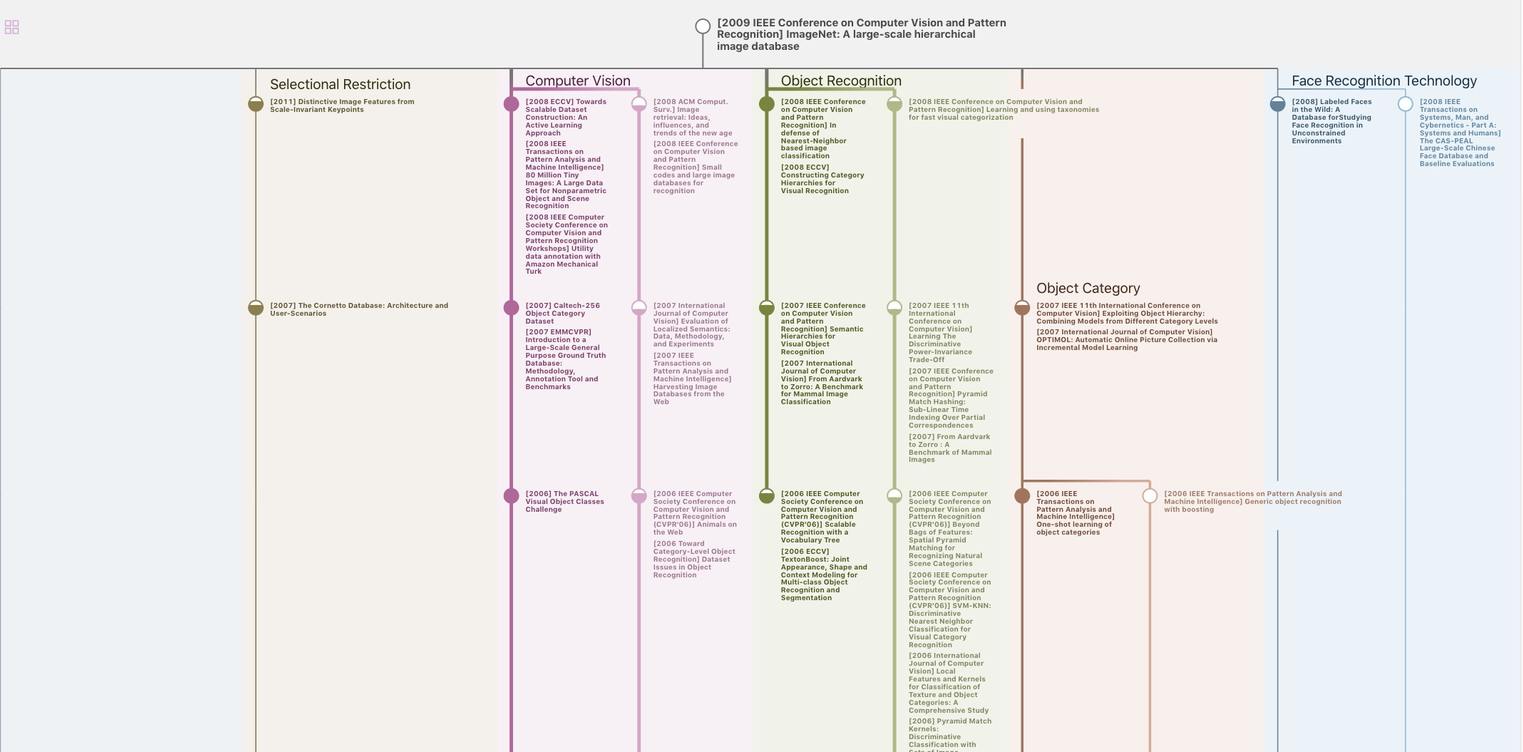
生成溯源树,研究论文发展脉络
Chat Paper
正在生成论文摘要