Adjusting for Ability Differences of Equating Samples when Randomization is Suboptimal
EDUCATIONAL MEASUREMENT-ISSUES and PRACTICE(2022)SCI 4区
Educ Testing Serv
Abstract
Test equating requires collecting data to link the scores from different forms of a test. Problems arise when equating samples are not equivalent and the test forms to be linked share no common items by which to measure or adjust for the group nonequivalence. Using data from five operational test forms, we created five pairs of research forms for each form, such that the equating relationship between each pair was known. Then we compared five approaches to adjusting for group nonequivalence in a situation where not only was group equivalence questionable, but the number of common items was small. We used a resampling approach to evaluate the linking accuracy of group adjustment using sample weights via minimum discriminant information adjustment (MDIA) using test takers' collateral (demographic) information, a weak anchor of only three items, or a mix of both. Overall, the use of both sample weights via MDIA and a weak anchor produced the most accurate result, while the direct (random groups) linking method assuming group equivalence produced the least accurate result due to nontrivial bias. For all five research forms, using both collateral information and anchor items only marginally improved linking accuracy compared to using the weak anchor alone.
MoreTranslated text
Key words
equating,minimum discriminant information adjustment,random groups design
求助PDF
上传PDF
View via Publisher
AI Read Science
AI Summary
AI Summary is the key point extracted automatically understanding the full text of the paper, including the background, methods, results, conclusions, icons and other key content, so that you can get the outline of the paper at a glance.
Example
Background
Key content
Introduction
Methods
Results
Related work
Fund
Key content
- Pretraining has recently greatly promoted the development of natural language processing (NLP)
- We show that M6 outperforms the baselines in multimodal downstream tasks, and the large M6 with 10 parameters can reach a better performance
- We propose a method called M6 that is able to process information of multiple modalities and perform both single-modal and cross-modal understanding and generation
- The model is scaled to large model with 10 billion parameters with sophisticated deployment, and the 10 -parameter M6-large is the largest pretrained model in Chinese
- Experimental results show that our proposed M6 outperforms the baseline in a number of downstream tasks concerning both single modality and multiple modalities We will continue the pretraining of extremely large models by increasing data to explore the limit of its performance
Upload PDF to Generate Summary
Must-Reading Tree
Example
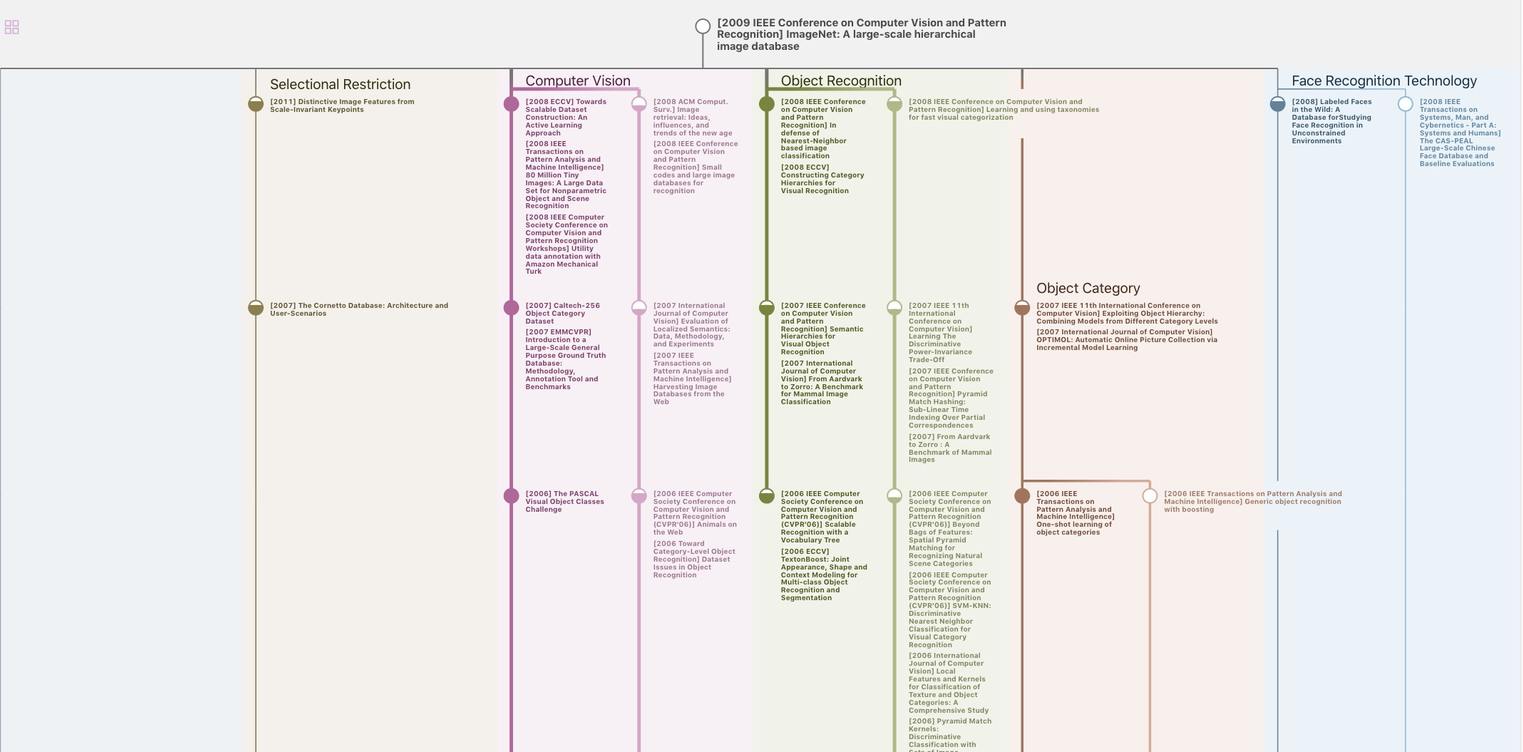
Generate MRT to find the research sequence of this paper
Related Papers
2018
被引用7 | 浏览
Data Disclaimer
The page data are from open Internet sources, cooperative publishers and automatic analysis results through AI technology. We do not make any commitments and guarantees for the validity, accuracy, correctness, reliability, completeness and timeliness of the page data. If you have any questions, please contact us by email: report@aminer.cn
Chat Paper
GPU is busy, summary generation fails
Rerequest