An Analysis on the Architecture and the Size of Quantized Hardware Neural Networks Based on Memristors
ELECTRONICS(2021)
摘要
We have performed different simulation experiments in relation to hardware neural networks (NN) to analyze the role of the number of synapses for different NN architectures in the network accuracy, considering different datasets. A technology that stands upon 4-kbit 1T1R ReRAM arrays, where resistive switching devices based on HfO2 dielectrics are employed, is taken as a reference. In our study, fully dense (FdNN) and convolutional neural networks (CNN) were considered, where the NN size in terms of the number of synapses and of hidden layer neurons were varied. CNNs work better when the number of synapses to be used is limited. If quantized synaptic weights are included, we observed that NN accuracy decreases significantly as the number of synapses is reduced; in this respect, a trade-off between the number of synapses and the NN accuracy has to be achieved. Consequently, the CNN architecture must be carefully designed; in particular, it was noticed that different datasets need specific architectures according to their complexity to achieve good results. It was shown that due to the number of variables that can be changed in the optimization of a NN hardware implementation, a specific solution has to be worked in each case in terms of synaptic weight levels, NN architecture, etc.
更多查看译文
关键词
memristor, multilevel operation, hardware neural network, deep neural network, convolutional neural network, network architecture, synaptic weight
AI 理解论文
溯源树
样例
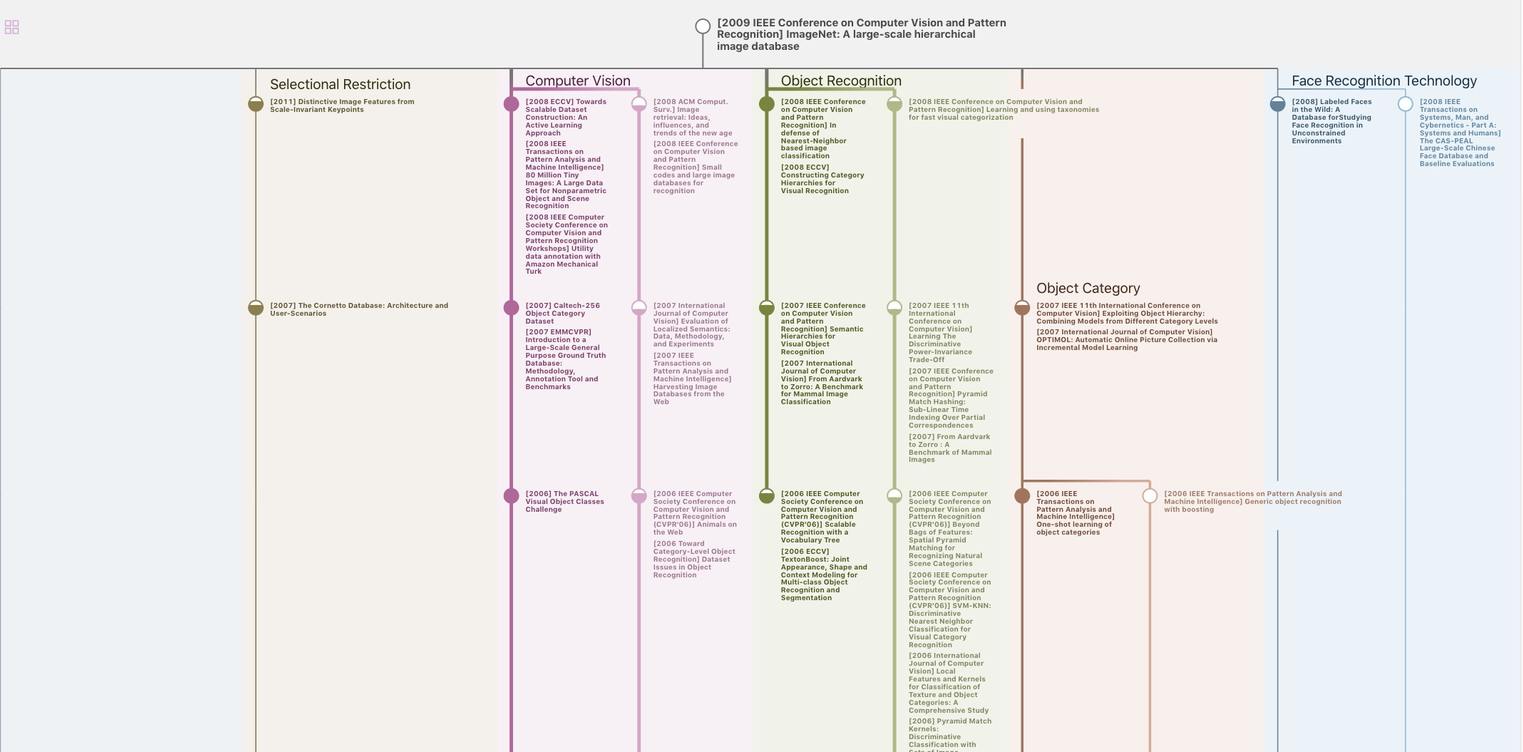
生成溯源树,研究论文发展脉络
Chat Paper
正在生成论文摘要