Semantic edge-guided object segmentation from high-resolution remotely sensed imagery
INTERNATIONAL JOURNAL OF REMOTE SENSING(2021)
摘要
Image segmentation is a basic task of Object-Based Image Analysis (OBIA) in remote sensing. Traditionally, the algorithms for this task are mostly based on region merging procedure. But the segmented objects may correlate poorly with the actual object boundary. Inspired by the recent remarkable improvements on edge detection with deep learning, we propose a semantic edge-guided segmentation with deep learning method to extract meaningful geographic objects from High-resolution Remote Sensing (HRS) images. The method consists of three stages: in the first stage, geographic object boundaries are manually labelled and randomly augmented to generate training data; in the second stage, a fully convolutional neural networks with Encoder-Decoder structure and multiscale supervised nets are trained to detect edges at multiple scales. The detected edges with semantic information do not only include local details but also global edge structure, which are more in accordance with human perception and suitable for conversion to actual geographic boundaries; in the third stage, the detected edges are thinned and extended to construct complete object boundaries according to calculated edge strength. The average precision of our method for the two datasets was 0.902 and 0.866, which is higher than the state-of-the-art deep learning models including RCF, BDCN and DexiNed have obtained. And line IoU improvement of at least 8.46% and F1 score improvement of at least 8.13% in the two datasets. The code of DDLNet is publicly available at https://github.com/Pikachu-zzZ/SEGOS.
更多查看译文
AI 理解论文
溯源树
样例
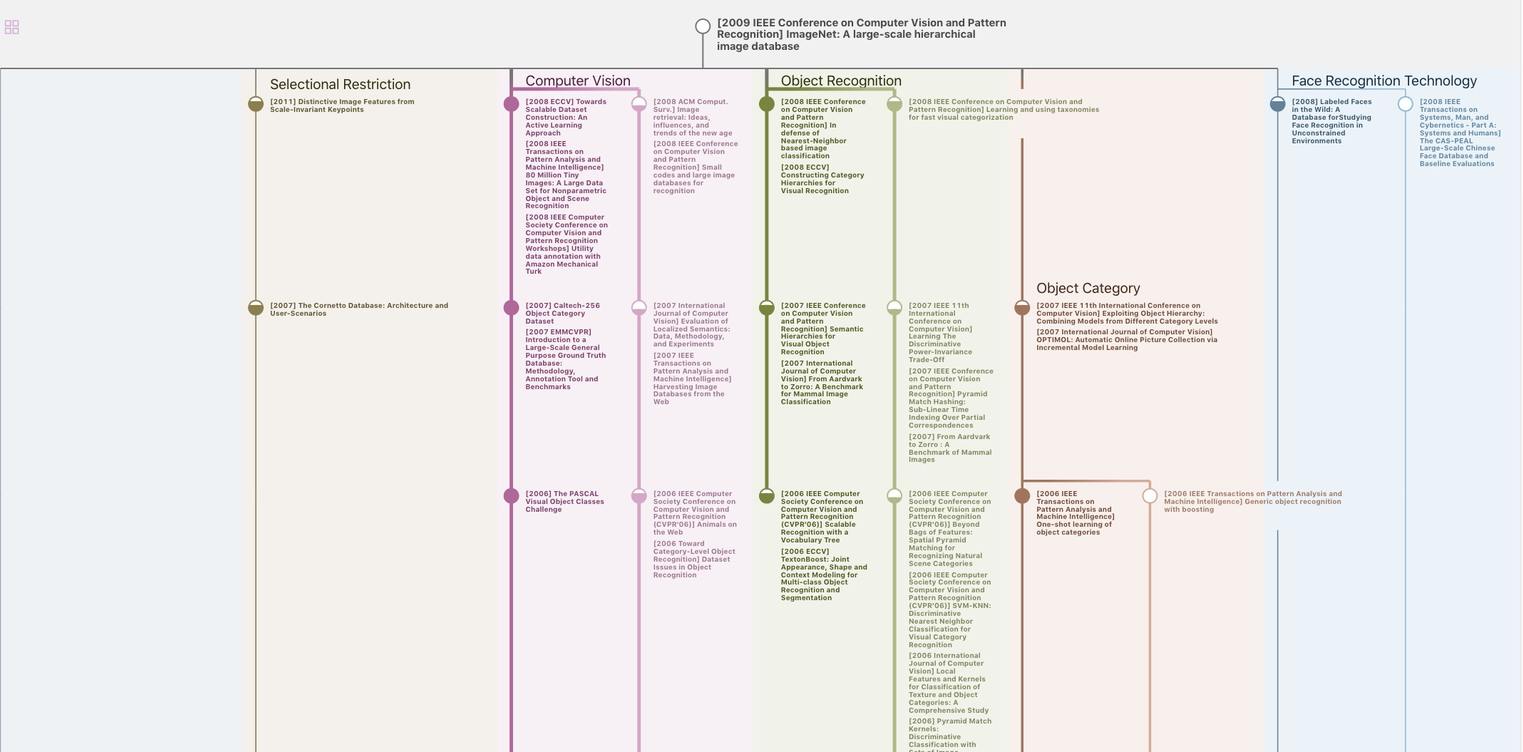
生成溯源树,研究论文发展脉络
Chat Paper
正在生成论文摘要