Process modeling with multi-level categorical inputs via variable selection and level aggregation
IISE TRANSACTIONS(2023)
摘要
An Industrial IoT-enabled manufacturing system often involves multiple categorical variables, denoting the process configurations and product customizations. These categorical variables lead to a flexible relationship between the input process variables and output quality measurements, as there are many potential configurations of the manufacturing process. This causes significant challenges for data-driven process modeling and root cause diagnosis. This article proposes a data-driven additive model to address the effects of different categorical variables on the relationship between process variables and quality measurements. The estimation algorithm automatically identifies the variables that have significant effects on the product quality, aggregates the levels of each categorical variable based on a priori knowledge of level similarity, and provides an accurate model that describes the relationship between the process variables and quality measurements. The simulation study validates the accuracy and effectiveness of the proposed method, and a case study on a hot rolling process shows that the method provides useful guidance on the understanding of the production system.
更多查看译文
关键词
Categorical variables, level aggregation, multivariate regression, root cause diagnosis
AI 理解论文
溯源树
样例
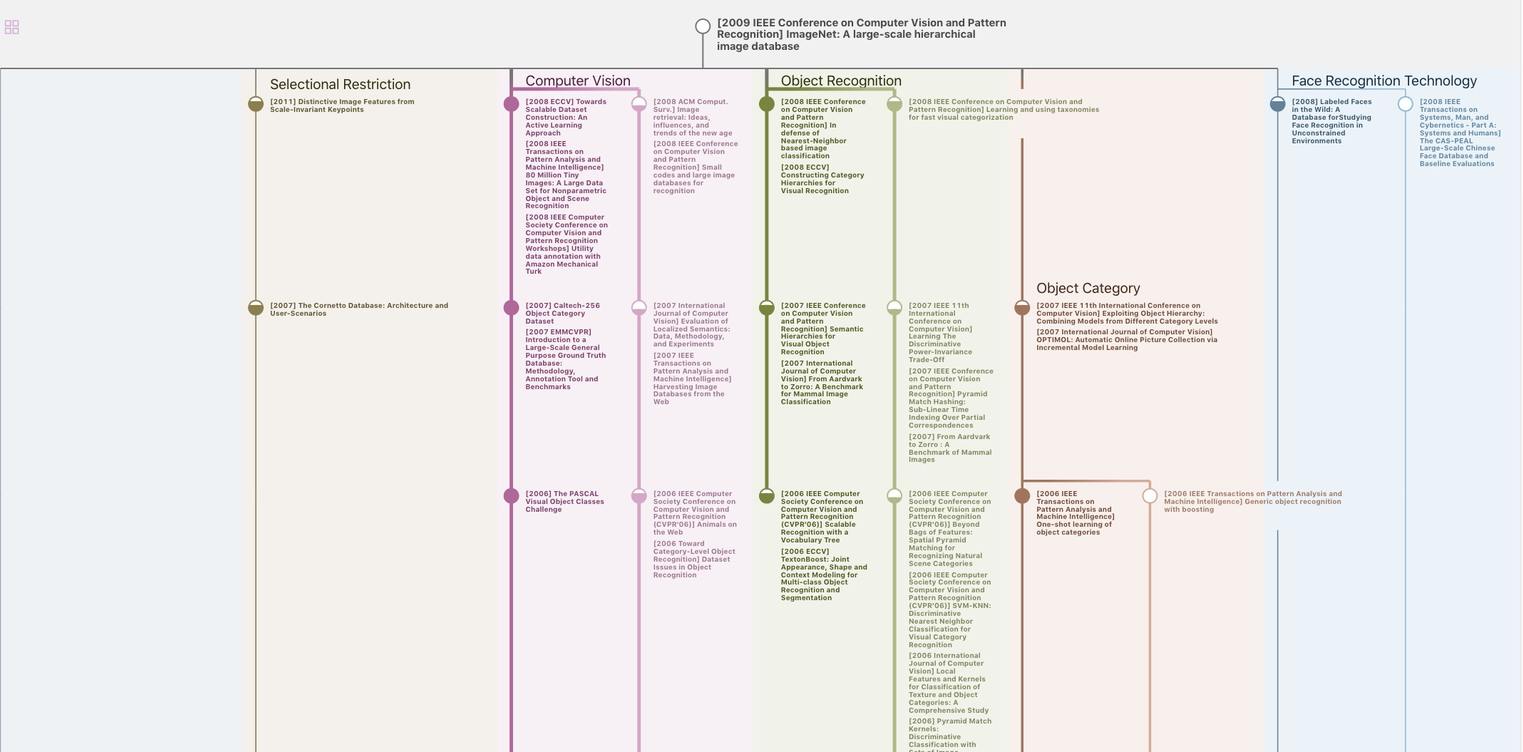
生成溯源树,研究论文发展脉络
Chat Paper
正在生成论文摘要