Evaluating Performance Jumps for Pavement Preventive Maintenance Treatments in Wet Freeze Climates Using Artificial Neural Network
JOURNAL OF TRANSPORTATION ENGINEERING PART B-PAVEMENTS(2022)
摘要
Preventive maintenance treatments are extensively used due to their active cost-effectiveness, and evaluating their short-term effectiveness is an important criterion. In this study, the performance jump, which is defined as the instantaneous decrease in distresses when a treatment is applied, was used. Long-Term Pavement Performance (LTPP) data from wet freeze climates was used to compare the performance jumps of different treatments under different weather, traffic, and asphalt pavement conditions. Maximum performance jumps of a thin asphalt overlay, slurry seal, crack seal, and chip seal were measured as 311, 307, 251, and 307 m, respectively. Overall, the performance jumps of the thin overlay and chip seal were most effective in eliminating non-wheel-path longitudinal cracking, whereas the slurry seal was less effective, and the crack seal was least effective. An artificial neural network was used to model the complex behavior among input variables and performance jumps. In a sensitivity analysis, the performance jump was predicted by varying one input variable to its maximum or minimum at a time while keeping other variables at their average values. The results showed that the maximum performance jump of 296.3 m was predicted when the annual average temperature was minimum for the chip seal, 279.6 m when the average annual daily truck traffic was maximum for the chip seal, and 277.3 m when last distress measured before applying a treatment was maximum in the thin overlay while other variables were at their average values. A principal component analysis was performed to transform the correlated input variables to uncorrelated principal components. The first and second principal components varied from 91.96% to 92.28% and 4.82% to 5.74%, respectively. The highest coefficient contributions for the first principal component were from annual average daily traffic and average annual daily truck traffic, and the highest coefficient contributions for the second principal component were from the annual average freeze index and annual average precipitation.
更多查看译文
关键词
Pavement preventive maintenance, Performance jump, Artificial neural network (ANN), Principal component analysis, Levenberg-Marquardt algorithm, Feedforward network
AI 理解论文
溯源树
样例
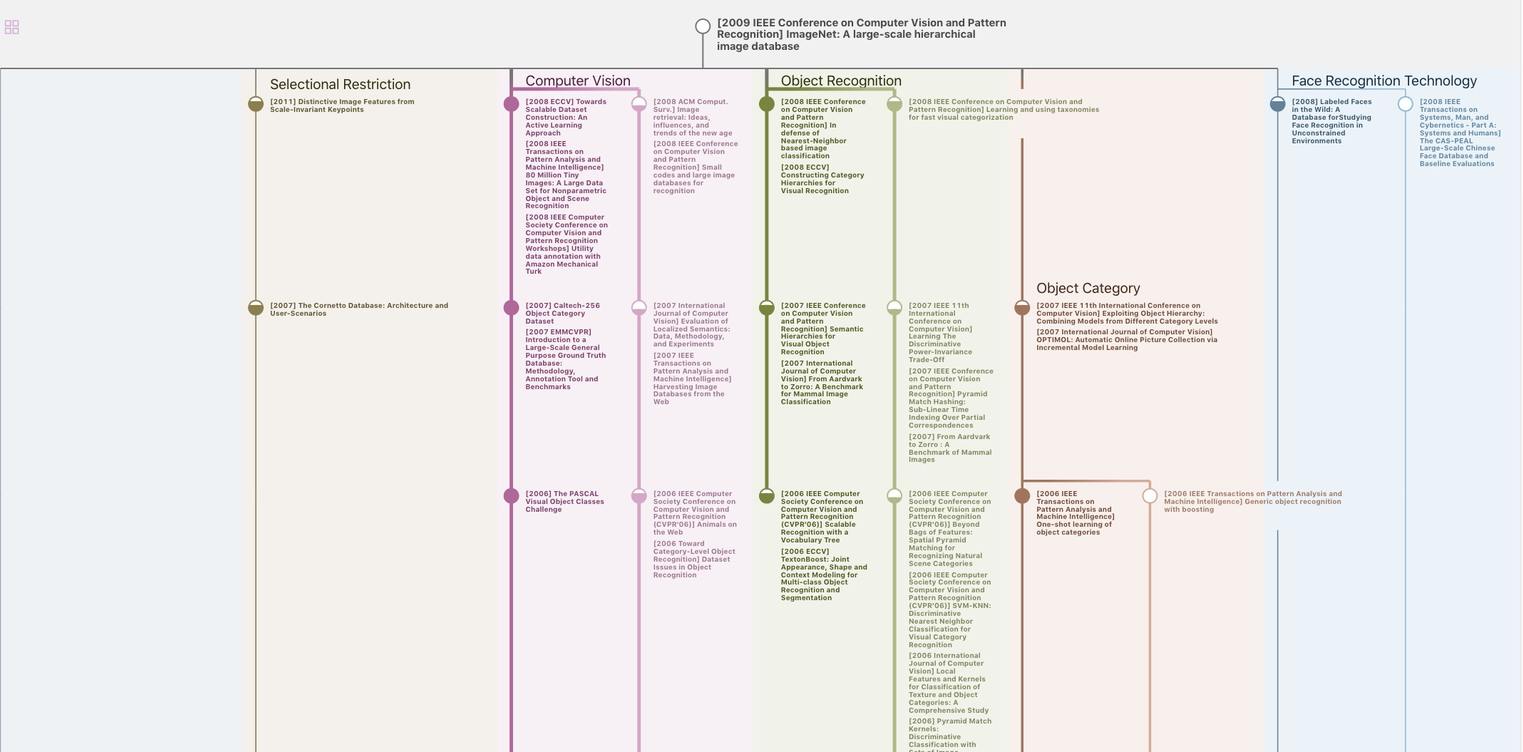
生成溯源树,研究论文发展脉络
Chat Paper
正在生成论文摘要