A Multi-task Text Classification Model Based on Label Embedding Learning
CYBER SECURITY, CNCERT 2021(2022)
摘要
Different text classification tasks have specific task features and the performance of text classification algorithm is highly affected by these task-specific features. It is crucial for text classification algorithms to extract task-specific features and thus improve the performance of text classification in different text classification tasks. The existing text classification algorithms use the attention-based neural network models to capture contextualized semantic features while ignores the task-specific features. In this paper, a text classification algorithm based on label-improved attention mechanism is proposed by integrating both contextualized semantic and task-specific features. Through label embedding to learn both word vector and modified-TF-IDF matrix, the task-specific features can be extracted and then attention weights are assigned to different words according to the extracted features, so as to improve the effectiveness of the attention-based neural network models on text classification. Experiments are carried on three text classification task data sets to verify the performance of the proposed method, including a six-category question classification data set, a two-category user comment data set, and a five-category sentiment data set. Results show that the proposed method has an average increase of 3.02% and 5.85% in F1 value compared with the existing LSTMAtt and SelfAtt models.
更多查看译文
关键词
Text classification, Label embedding, Attention mechanism, Multi-task
AI 理解论文
溯源树
样例
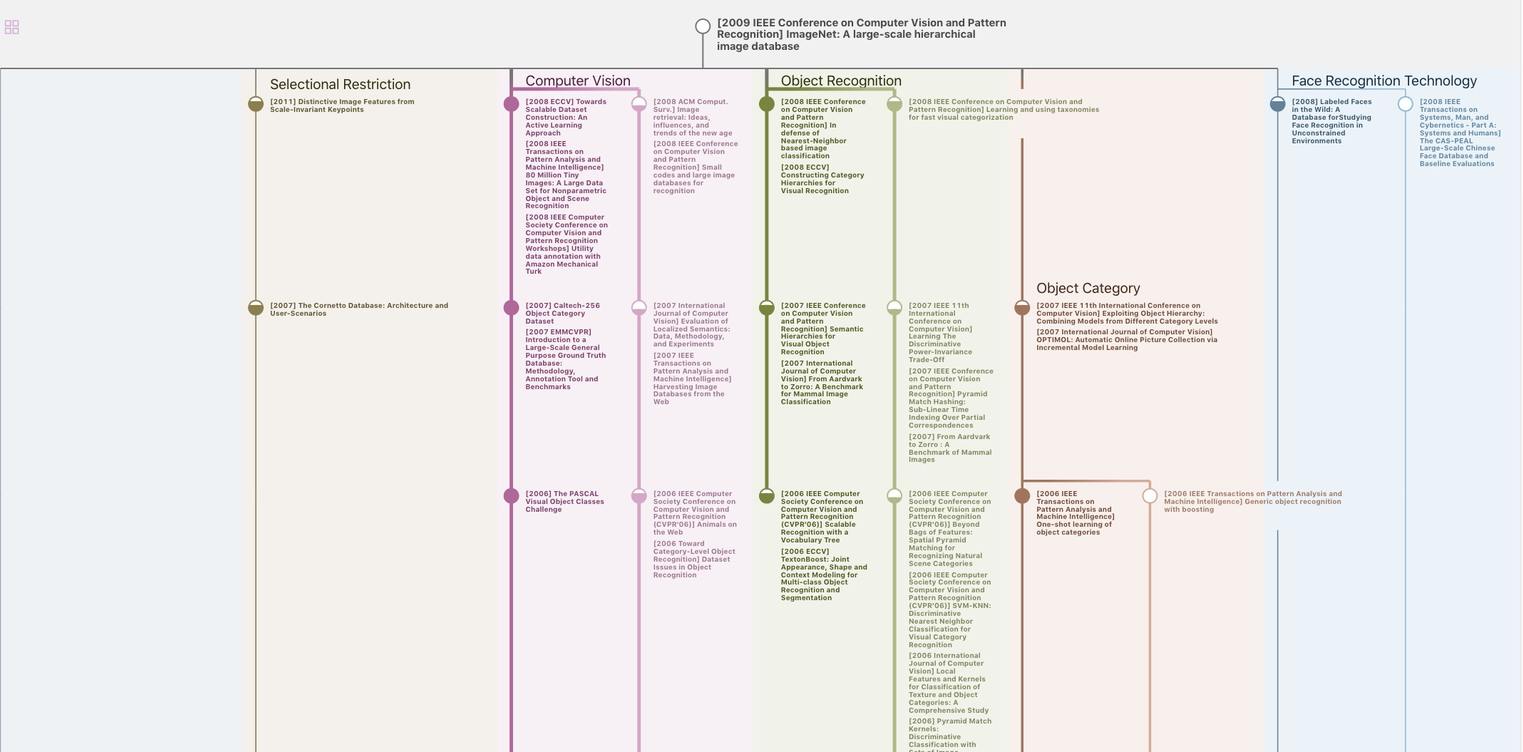
生成溯源树,研究论文发展脉络
Chat Paper
正在生成论文摘要