Surrogate modeling for efficiently, accurately and conservatively estimating measures of risk
Reliability Engineering & System Safety(2022)
摘要
We present a surrogate modeling framework for conservatively estimating measures of risk from limited realizations of an expensive physical experiment or computational simulation. Risk measures combine objective probabilities with the subjective values of a decision maker to quantify anticipated outcomes. Given a set of samples, we construct a surrogate model that produces estimates of risk measures that are always greater than their empirical approximations obtained from the training data. These surrogate models limit over-confidence in reliability and safety assessments and produce estimates of risk measures that converge much faster to the true value than purely sample-based estimates. We first detail the construction of conservative surrogate models that can be tailored to a stakeholder’s risk preferences and then present an approach, based on stochastic orders, for constructing surrogate models that are conservative with respect to families of risk measures. Our surrogate models include biases that permit them to conservatively estimate the target risk measures. We provide theoretical results that show that these biases decay at the same rate as the L2 error in the surrogate model. Numerical demonstrations confirm that risk-adapted surrogate models do indeed overestimate the target risk measures while converging at the expected rate.
更多查看译文
关键词
Surrogate models,Risk measures,Stochastic dominance,Least-squares regression,Statistical Models,Polynomial Chaos,Risk Management
AI 理解论文
溯源树
样例
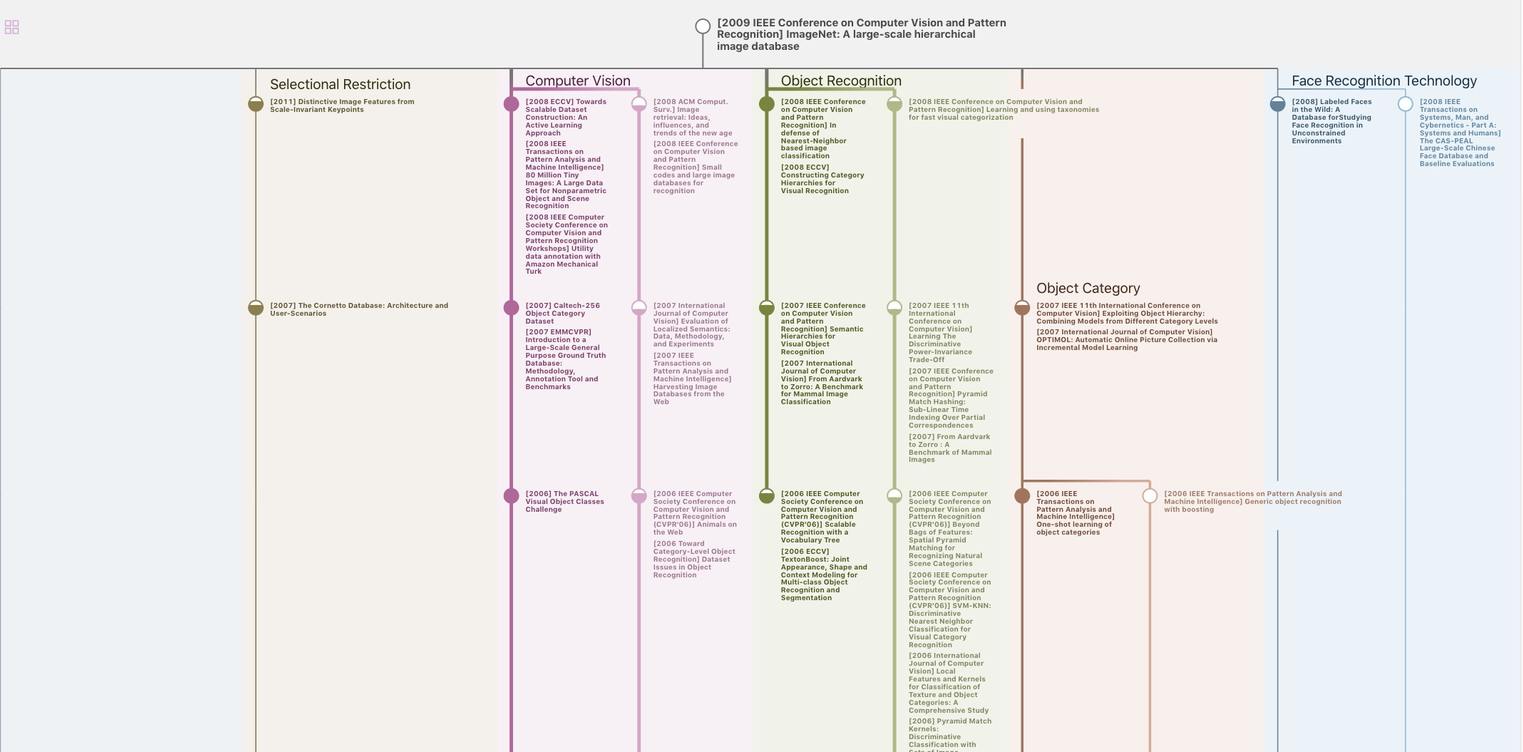
生成溯源树,研究论文发展脉络
Chat Paper
正在生成论文摘要