Multi-Size Object Detection in Large Scene Remote Sensing Images Under Dual Attention Mechanism
IEEE ACCESS(2022)
摘要
The remote sensing images in large scenes have a complex background, and the types, sizes, and postures of the targets are different, making object detection in remote sensing images difficult. To solve this problem, an end-to-end multi-size object detection method based on a dual attention mechanism is proposed in this paper. First, the MobileNets backbone network is used to extract multi-layer features of remote sensing images as the input of MFCA, a multi-size feature concentration attention module. MFCA employs an attention mechanism to suppress noise, enhance effective feature reuse, and improve the adaptability of the network to multi-size target features through multi-layer convolution operation. Then, TSDFF (two-stage deep feature fusion module)deeply fuses the feature maps output by MFCA to maximize the correlation between the feature sets and especially improve the feature expression of small targets. Next, the GLCNet (global-local context network) and the SSA (significant simple attention module) distinguish the fused features and screen out useful channel information, which makes the detected features more representative. Finally, the loss function is improved to truly reflect the difference between the candidate frames and the real frames, enhancing the network's ability to predict complex samples. The performance of our proposed method is compared with other advanced algorithms on NWPU VHR-10, DOTA, RSOD open datasets. Experimental results show that our proposed method achieves the best AP (average precision) and mAP (mean average precision), indicating that the method can accurately detect multi-type, multi-size, and multi-posture targets with high adaptability.
更多查看译文
关键词
Feature extraction, Object detection, Remote sensing, Deep learning, Convolution, Classification algorithms, Sensors, Deep learning, dual attention mechanism, multi-size object detection, remote sensing images
AI 理解论文
溯源树
样例
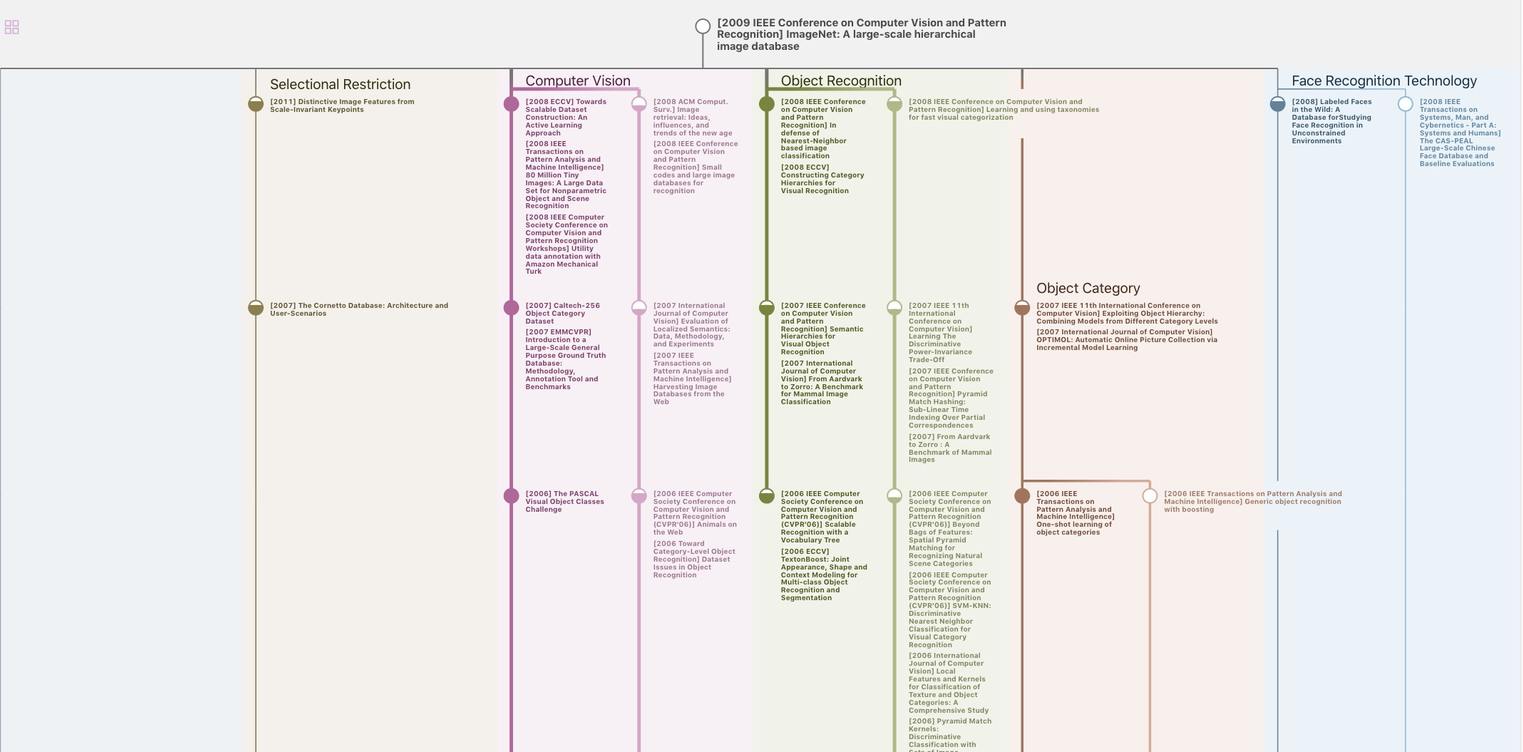
生成溯源树,研究论文发展脉络
Chat Paper
正在生成论文摘要