A Vibrational Signal Fault Diagnosis Rule Extraction Method Based on DST-ACI Discriminant Criterion
SHOCK AND VIBRATION(2021)
摘要
A fault diagnosis rule extraction method oriented to machine foot signal based on dynamic support threshold and association coefficient interestingness (DST-ACI) discriminant criterion is proposed in this paper. The new method includes three main innovations. First, the feature state coding method based on K-means clustering fully takes into account the imbalanced distribution of signal feature values due to the noise interference, and divide the signal feature values into several range intervals to generate the feature state code. Second, the frequent feature pattern mining method based on dynamic support threshold (DST) discriminant criterion can dynamically adjust support threshold according to the frequency of the feature states in each candidate pattern. Third, the fault diagnosis rule extraction method based on the association coefficient interestingness (ACI) discriminant criterion introduces a new metrics called ACI to evaluate the correlation between the pattern and the fault. Four types of fault simulation experiments were carried out, and the performance of the DST-ACI method was tested using the collected vibration signal. The results show that compared with the coding method based on equal-width discretization or equal-density discretization, the accuracy of the transactional dataset generated by the feature state coding method based on K-means clustering is higher. Compared with the frequent feature pattern mining method based on the constant support threshold criterion, the pattern mined by the DST-based criterion has generally higher support. Compared with the existing confidence-lift-based and confidence-improint-based fault diagnosis rule extraction frameworks, the positive correlation between the feature states and the fault type of the rules extracted based on the DST-ACI framework is generally stronger.
更多查看译文
AI 理解论文
溯源树
样例
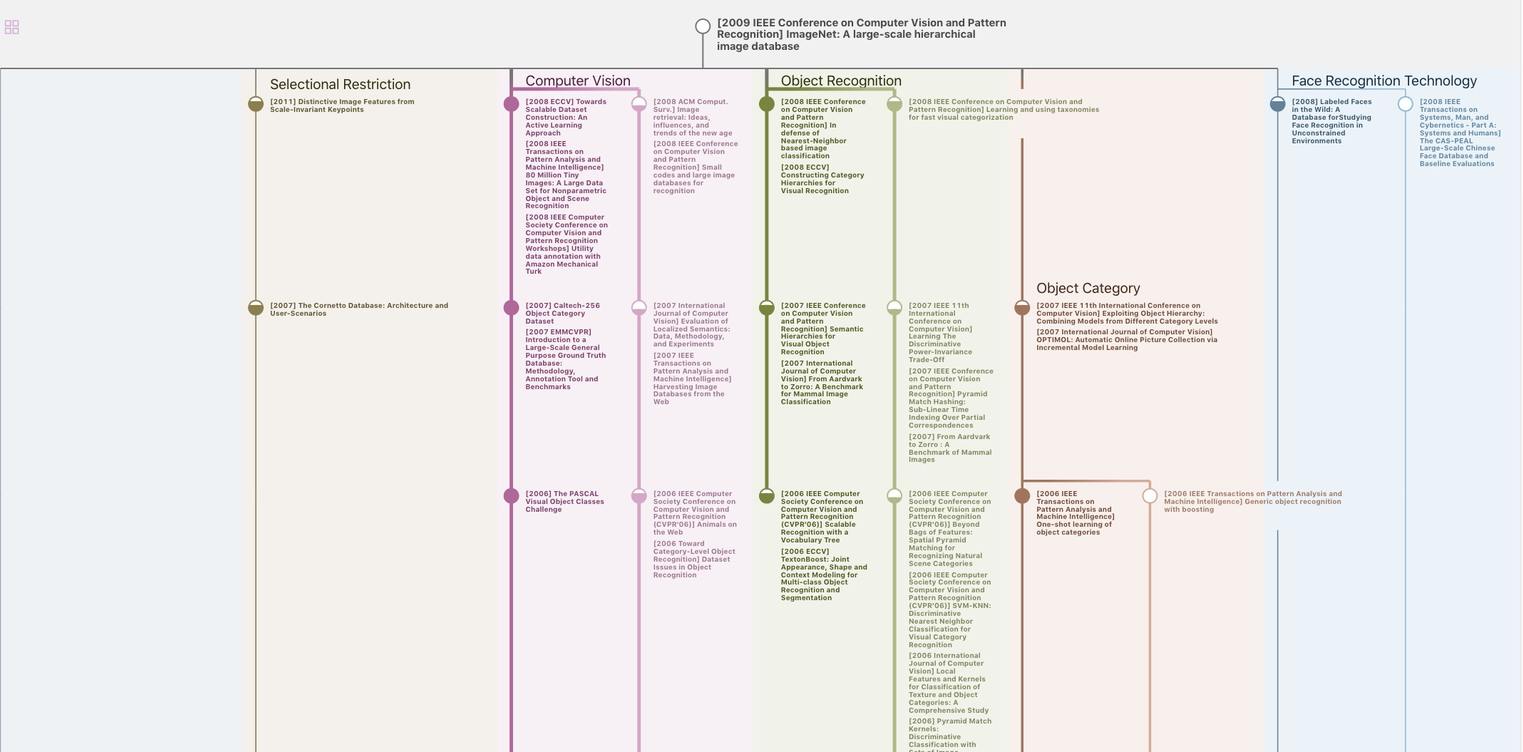
生成溯源树,研究论文发展脉络
Chat Paper
正在生成论文摘要